MAGEC - Machine Assisted Geometry Extraction and Creation
Proceedings of SPIE(2020)
Abstract
The GIS industry relies heavily on manual efforts to build and maintain digital maps. This approach is timeconsuming and requires a sizable workforce not only for map-making but also for quality-checks that are required to resolve the potential errors resulting from manual digitization. With recent advancements in computer vision, several organizations are using machine-learning algorithms to generate map data from images. In the current machine learning based geometry creation process three limitations prevails. Firstly, the output of the algorithms is never served on-demand to a map editing tool. Secondly, after being further fine-tuned manually by annotators/validators, the results are never fed back to the algorithms to identify the errors incurred and improve accuracy. Finally, a lot of manual effort is required to create training data for new terrains and regions. We propose an end-to-end machine learning system integrated with current map-making tools to address these limitations and reduce the manual effort in creating and updating geometry.
MoreTranslated text
Key words
map editing,semantic segmentation,GIS,computer vision,map making,CNN
AI Read Science
Must-Reading Tree
Example
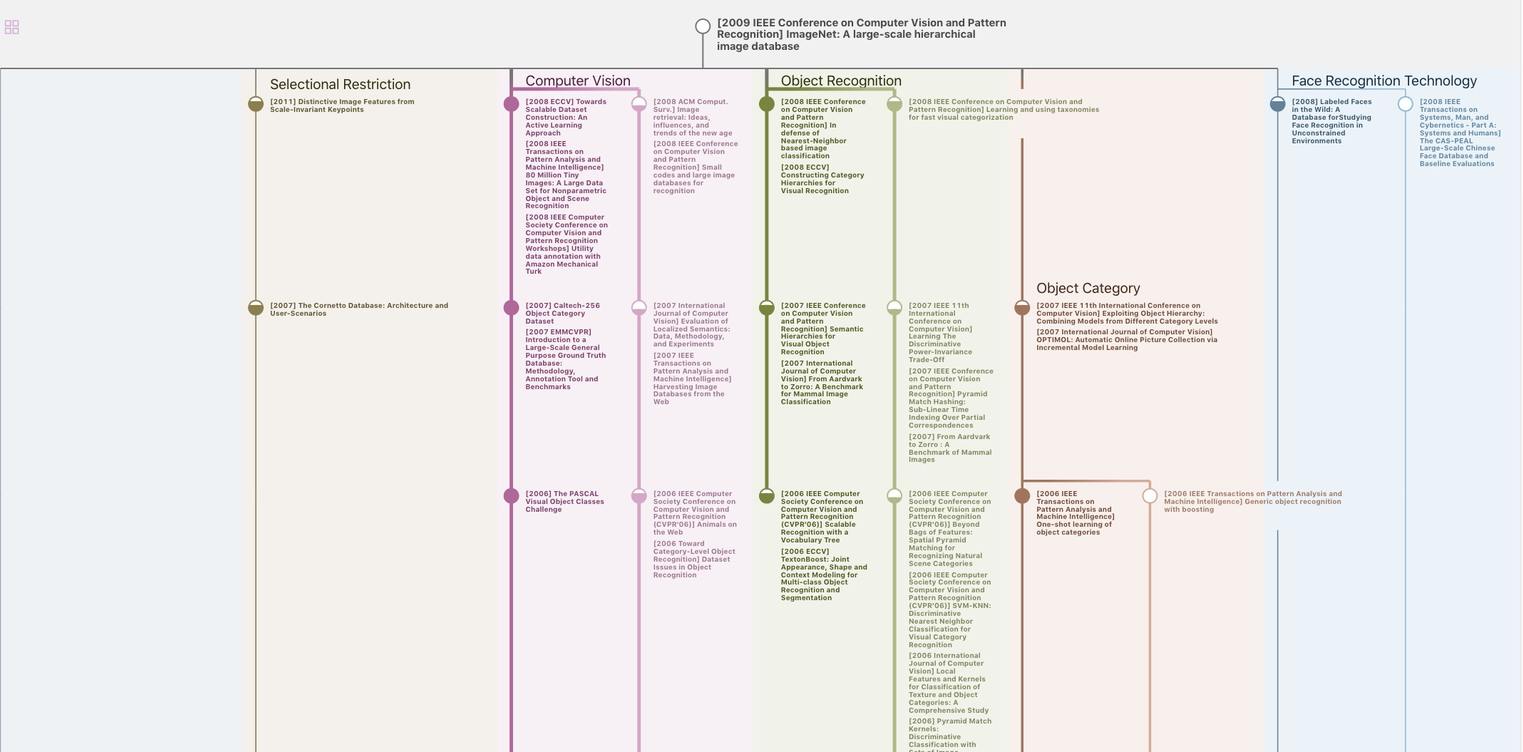
Generate MRT to find the research sequence of this paper
Chat Paper
Summary is being generated by the instructions you defined