A novel rolling bearing defect detection method based on bispectrum analysis and cloud model-improved EEMD
IEEE Access(2020)
Abstract
Mechanical signals are not only disturbed by Gaussian noise, but also by non-Gaussian noise. These Gaussian noise and non-Gaussian noise have gravely impeded detecting of rolling bearing defects using traditional methods. In this context, the paper develops a novel detection method for rolling bearing, which combines bispectrum analysis with an improved ensemble empirical mode decomposition (EEMD). To effectively eliminate Gaussian noise in the signal, bispectrum analysis is adopted. In order to effectively reduce non-Gaussian noise, a cloud model-improved EEMD is proposed, where the cloud model is introduced to restrain the mode mixing phenomenon. Then a rolling bearing defect detection plan based on the proposed method is put forward. From theoretical analysis and experimental verification, it is demonstrated that the proposed method has superior performance in reducing multiple background noise. Furthermore, compared with other three methods, the results show that the proposed method can detect the defect of rolling bearings more effectively.
MoreTranslated text
Key words
Bispectrum analysis, cloud model, defect detection, ensemble empirical mode decomposition, mode mixing, rolling bearing
AI Read Science
Must-Reading Tree
Example
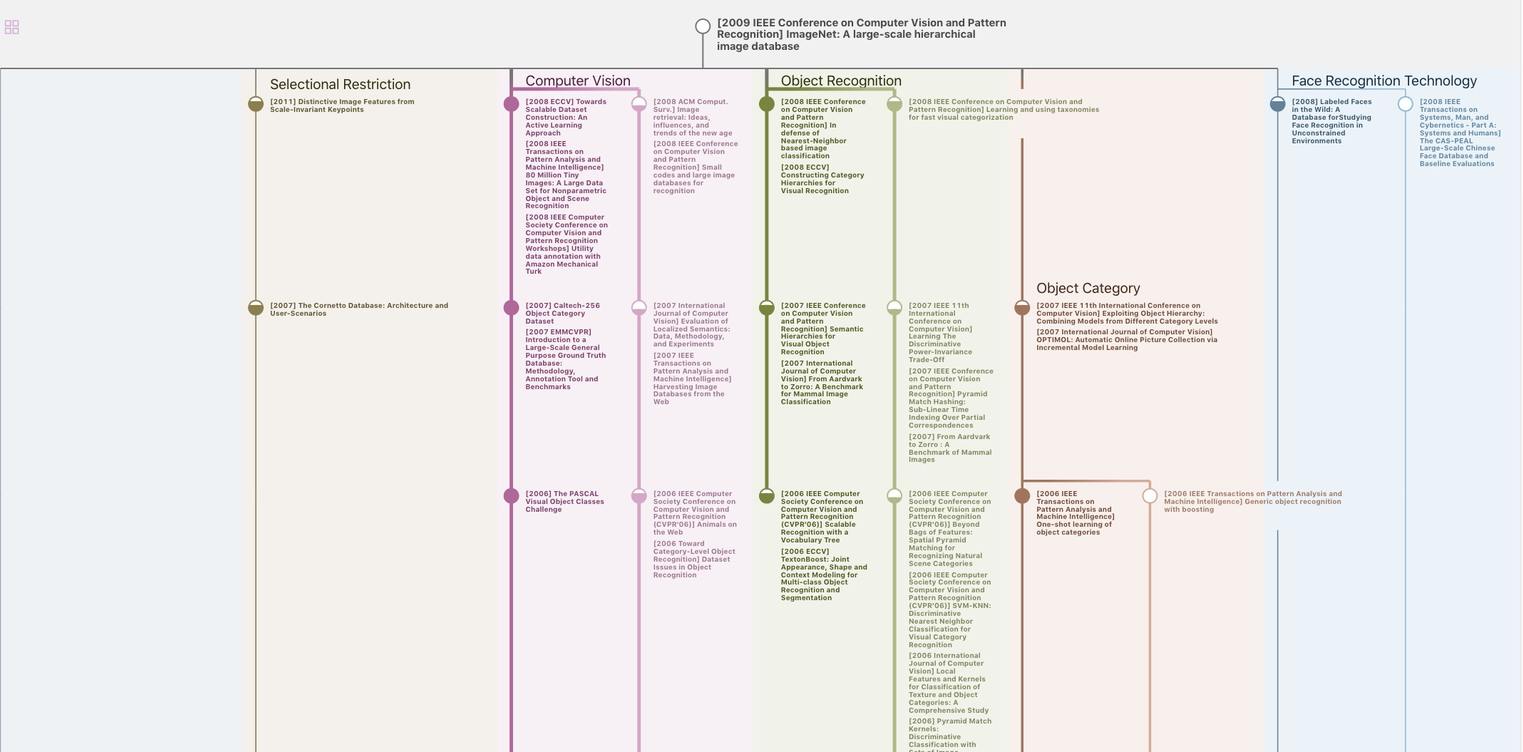
Generate MRT to find the research sequence of this paper
Chat Paper
Summary is being generated by the instructions you defined