Nelder-Mead Optimization Of Elastic Metamaterials Via Machine-Learning-Aided Surrogate Modeling
INTERNATIONAL JOURNAL OF APPLIED MECHANICS(2020)
Abstract
One of the fundamental challenges of structural optimization of elastic metamaterials (EMMs) with complex geometry lies within the high consumption of computational power associated with finite element analysis (FEA) simulations, which often render the numerical solution an ineffective and costly attempt. Additionally, due to the inherent mesh dependence of the FEA method, minuscule geometry features, which often arise during optimization, demand very fine elements, resulting in enormously high time consumption, especially when repetitive solutions are needed for objective function validations. In this study, we developed a surrogate modeling algorithm to reduce computational time in structural optimization of EM Ms. The surrogate model was constructed based on a multilayer feedforward artificial neural network (ANN) architecture, trained with machine learning techniques and optimized through a genetic algorithm (GA) program to improve its accuracy in predicting the location and width of the primary elastic band gap. With the trained and optimized ANN model at the core, a Nelder-Mead (NM) algorithm was established and its performance inspected in comparison to the traditional FEA method. The ANN-NM model shows remarkable accuracy in predicting the band gap width and impressive reduction in the time consumption.
MoreTranslated text
Key words
Artificial neural network, mechanical metamaterial, welder-Mead method, machine learning, surrogate modeling
AI Read Science
Must-Reading Tree
Example
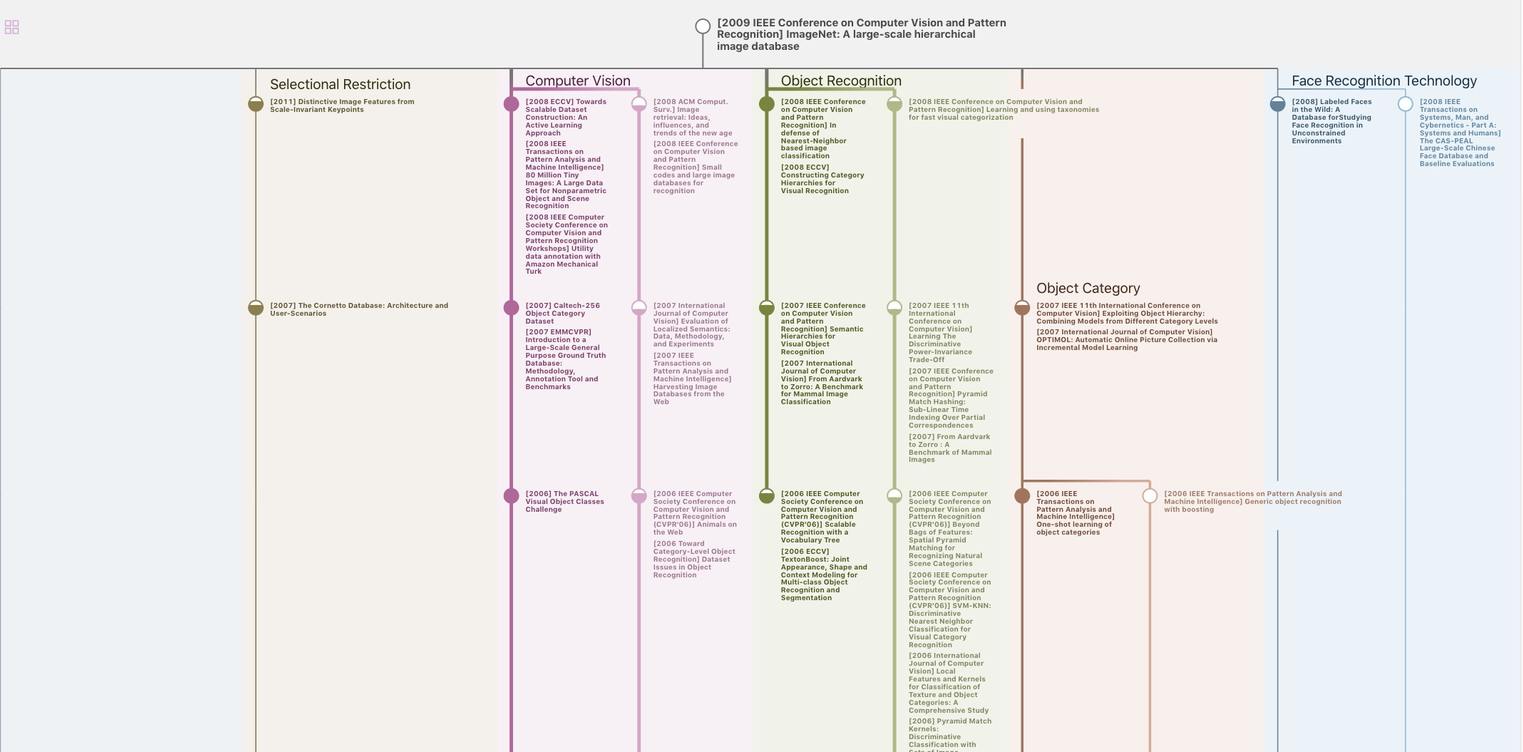
Generate MRT to find the research sequence of this paper
Chat Paper
Summary is being generated by the instructions you defined