Learning the Time-Varying Massive MIMO Channels: Robust Estimation and Data-Aided Prediction
IEEE Transactions on Vehicular Technology(2020)
摘要
The quasi-static assumption of channels becomes invalid in a number of emerging applications of massive multiple-input multiple-output (MIMO) systems with high base station (BS)/user mobility, such as high speed train and unmanned aerial vehicle communications. In these situations, the time variation of channels shortens the channel coherence time and decreases the efficiency of traditional channel estimation schemes significantly. This paper focuses on the time-varying channel estimation problem in massive MIMO systems, where the mobility of BS/user is assumed to be high. The sparse property of the time-varying massive MIMO channels is analyzed, which reveals that the angular domain channels exhibit two kinds of spatial-temporal sparse (STS) structures, namely the temporal-common sparse structure and spatial-clustered sparse structure. By exploiting the STS structures, a novel structured variational Bayesian inference (VBI) framework is formulated to make a robust channel estimation during the training phase. A data-aided channel predication scheme is further proposed to combat the time variation of the channels during the data transmission phase without increasing the pilot consumption. The simulation results demonstrate the superiority of the proposed scheme in the time-varying scenarios with different BS/user mobilities.
更多查看译文
关键词
Massive MIMO systems,time-varying channel estimation,spatial-temporal sparse structures,structured variational Bayesian inference,data-aided prediction
AI 理解论文
溯源树
样例
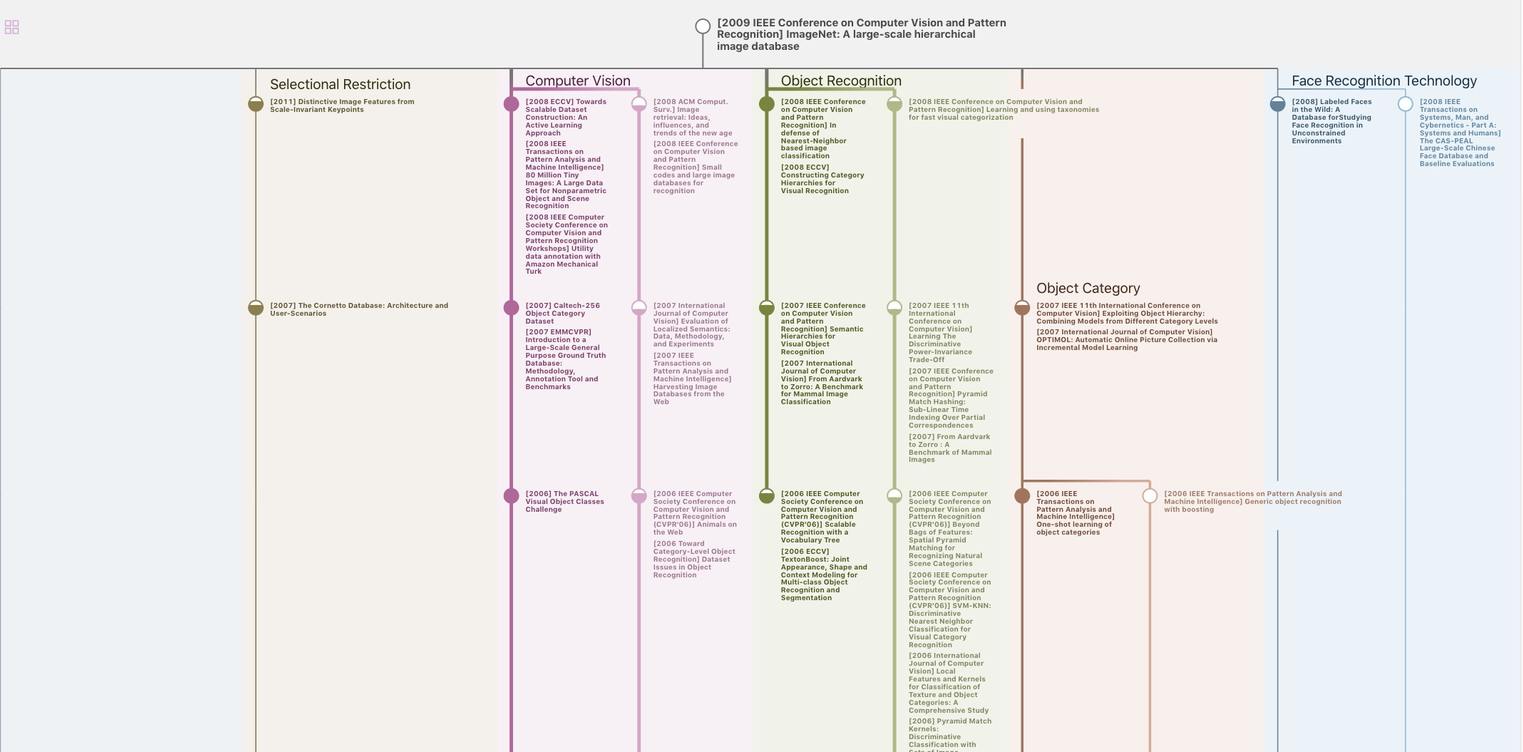
生成溯源树,研究论文发展脉络
Chat Paper
正在生成论文摘要