Non-Rigid Joint Registration for Multi-Contrast MR of Infant Brain Based on the Unsupervised Deep Regression Network
CISP-BMEI(2019)
摘要
At present, the non-rigid registration for adult brain Magnetic Resonance (MR) images is widely used, but it still faces challenges in the registration of infant brain MR images because of the low SNR. In this paper, we proposed a multi-input channel unsupervised deep regression network (DRN) to achieve non-rigid joint registration for multi-contrast MR, aiming to improve the registration accuracy of infant brain MR images. We firstly designed a U-Net-like network with multiple input channels, which can separately learn the information of T1- and T2- weighted MR images. The model can estimate one deformation field with information within both images to improve registration accuracy. For the multi-contrast MR, other registration algorithms produce two deformation fields but it is hard to evaluate which one is better. Secondly, the model can be trained in an unsupervised and auto-context manner with a multi-scale loss function. That allows the model to perform coarse registration with the large-scale loss function, and then fine registration with the small-scale loss function to achieve better results. Experimental results show that our algorithm reaches state-of-the-art accuracy and outperforms the others in some aspects for the registration of infant brain MR.
更多查看译文
关键词
Non-rigid Medical Image Registration,Multi-contrast MR,Infant Brain MR,Deep Regression Network
AI 理解论文
溯源树
样例
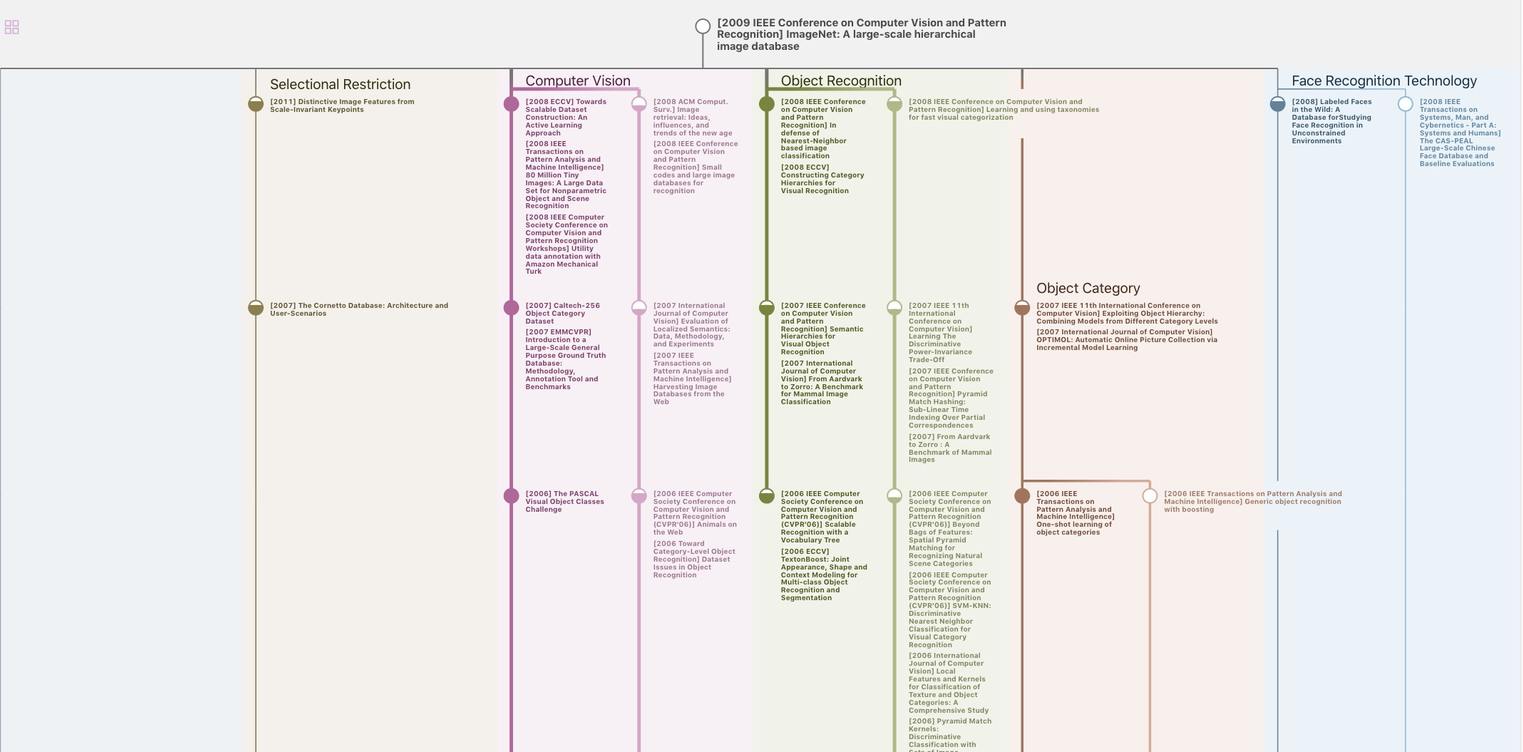
生成溯源树,研究论文发展脉络
Chat Paper
正在生成论文摘要