Time series segmentation for state-model generation of autonomous aquatic drones: A systematic framework
Engineering Applications of Artificial Intelligence(2020)
摘要
Autonomous surface vessels are becoming increasingly important for water monitoring. Their aim is to navigate rivers and lakes with limited intervention of human operators, to collect real-time data about water parameters. To reach this goal, these intelligent systems must interact with the environment and act according to the situations they face. In this work we propose a framework based on the integration of recent time-series clustering/segmentation methods and cluster validity indices, for detecting, modeling and evaluating aquatic drone states. The approach is completely data-driven and unsupervised. It takes unlabeled multivariate time series of sensor traces and returns both a set of statistically significant state-models (generated by different mathematical approaches) and a related segmentation of the dataset. We test the approach on a real dataset containing data of six campaigns, two in rivers and four in lakes, in different countries for about 5.6 h of navigation. Results show that the methodology is able to recognize known states and to discover unknown states, enabling novelty detection. The approach is therefore an easy-to-use tool for discovering and interpreting significant states in sensor data, that enables improved data analysis and drone autonomy.
更多查看译文
关键词
Time series segmentation,Situation assessment,State-model generation,Autonomous surface vessels,Activity recognition,Water monitoring,Model interpretation/explanation,Sensor data analysis
AI 理解论文
溯源树
样例
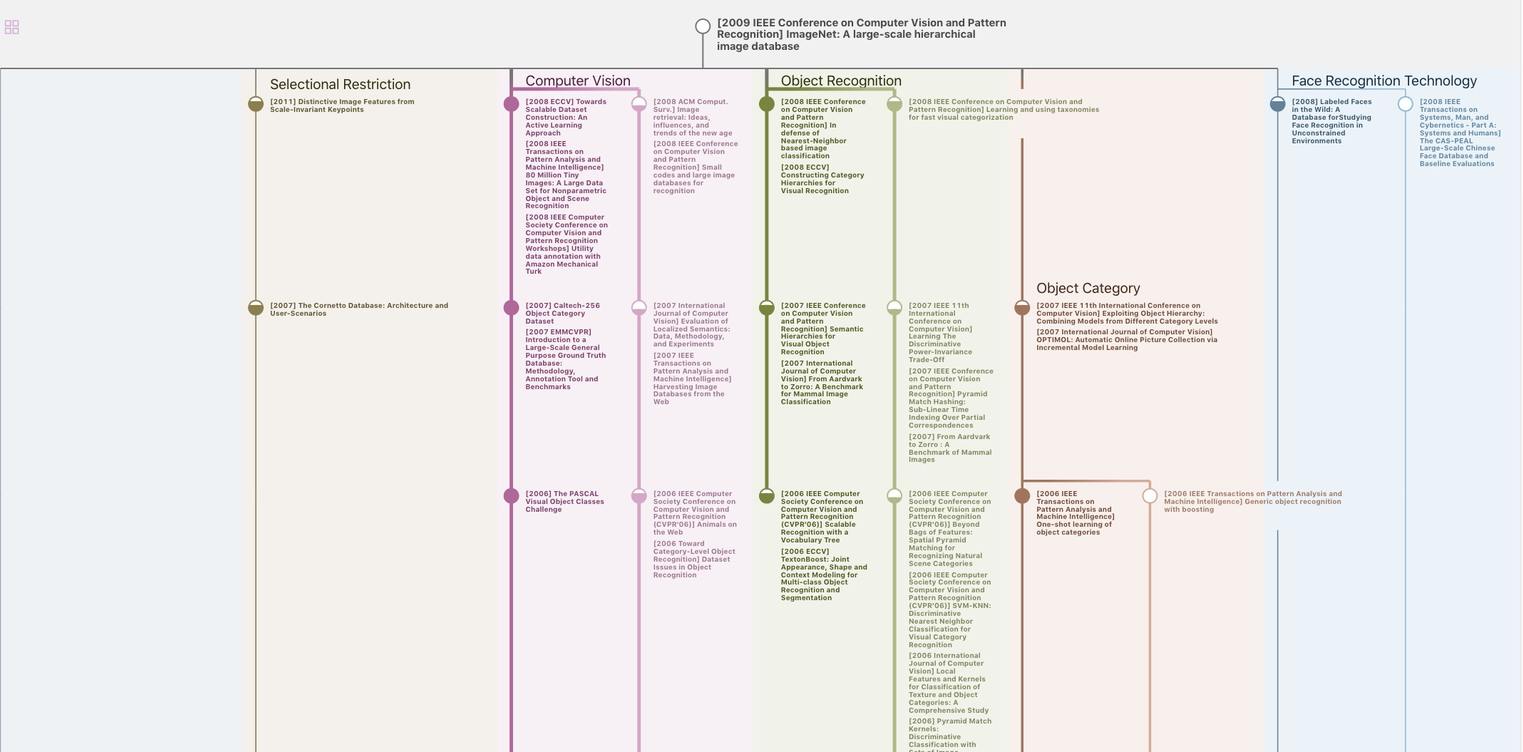
生成溯源树,研究论文发展脉络
Chat Paper
正在生成论文摘要