Multi-weighted nuclear norm minimization for real world image denoising
Optik(2020)
摘要
The noise in real world images is much more complicated than additive Gaussian white noise that most existing denoising methods are designed for. The performances of the denoising methods aiming at additive Gaussian white noise on real world images are not satisfactory. A major feature of noise in real world images is that the noise levels vary with regions. We propose a denoising model named multi-weighted nuclear norm minimization according to the characteristic of the noise in real images which is the noise levels varying with regions. In our model, the objective function is divided into two parts: the data fidelity term and the regularization term. The regularization term is a weighted nuclear norm. We use two weight matrices on the data fidelity term to balance the data between channels and between regions, respectively. Since the objective function has no analytical solution, we use alternating direction method of multipliers to decompose the objective function into sub-problems with analytical solutions. We prove the effectiveness of the proposed model by experiments.
更多查看译文
关键词
Low-rank,Non-local self-similarity,Sparsity,Color image denoising
AI 理解论文
溯源树
样例
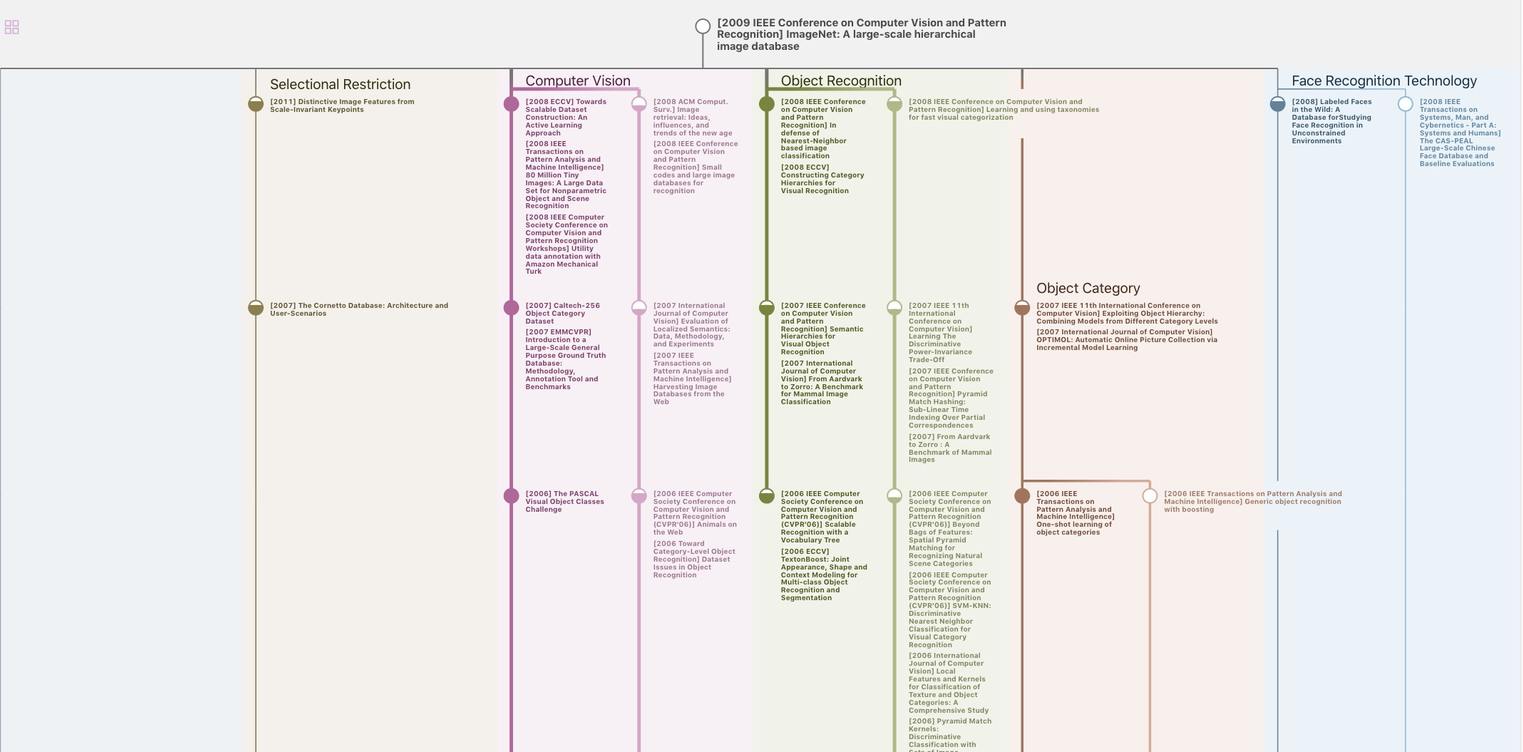
生成溯源树,研究论文发展脉络
Chat Paper
正在生成论文摘要