A Unified And Unsupervised Framework For Bilingual Phrase Alignment On Specialized Comparable Corpora
ECAI 2020: 24TH EUROPEAN CONFERENCE ON ARTIFICIAL INTELLIGENCE(2020)
摘要
Significant advances have been achieved in bilingual word-level alignment, yet the challenge remains for phrase-level alignment. Moreover, the need for parallel data is a critical drawback for the alignment task. In particular, this makes multi-word terms very difficult to align in specialized domains. This work proposes a system that alleviates these two problems: a unified phrase representation model using cross-lingual word embeddings as input, and an unsupervised training algorithm inspired by recent works on neural machine translation. The system consists of a sequence-to-sequence architecture where a short sequence encoder constructs cross-lingual representations of phrases of any length, then an LSTM network decodes them w.r.t their contexts. After training, our encoder provides cross-lingual phrase representations that can be compared without further transformation. Experiments on five specialized domain datasets show that our method obtains state-of-the-art results on the bilingual phrase alignment task, and improves the results of different length phrase alignment by a mean of 8.8 points in MAP.
更多查看译文
AI 理解论文
溯源树
样例
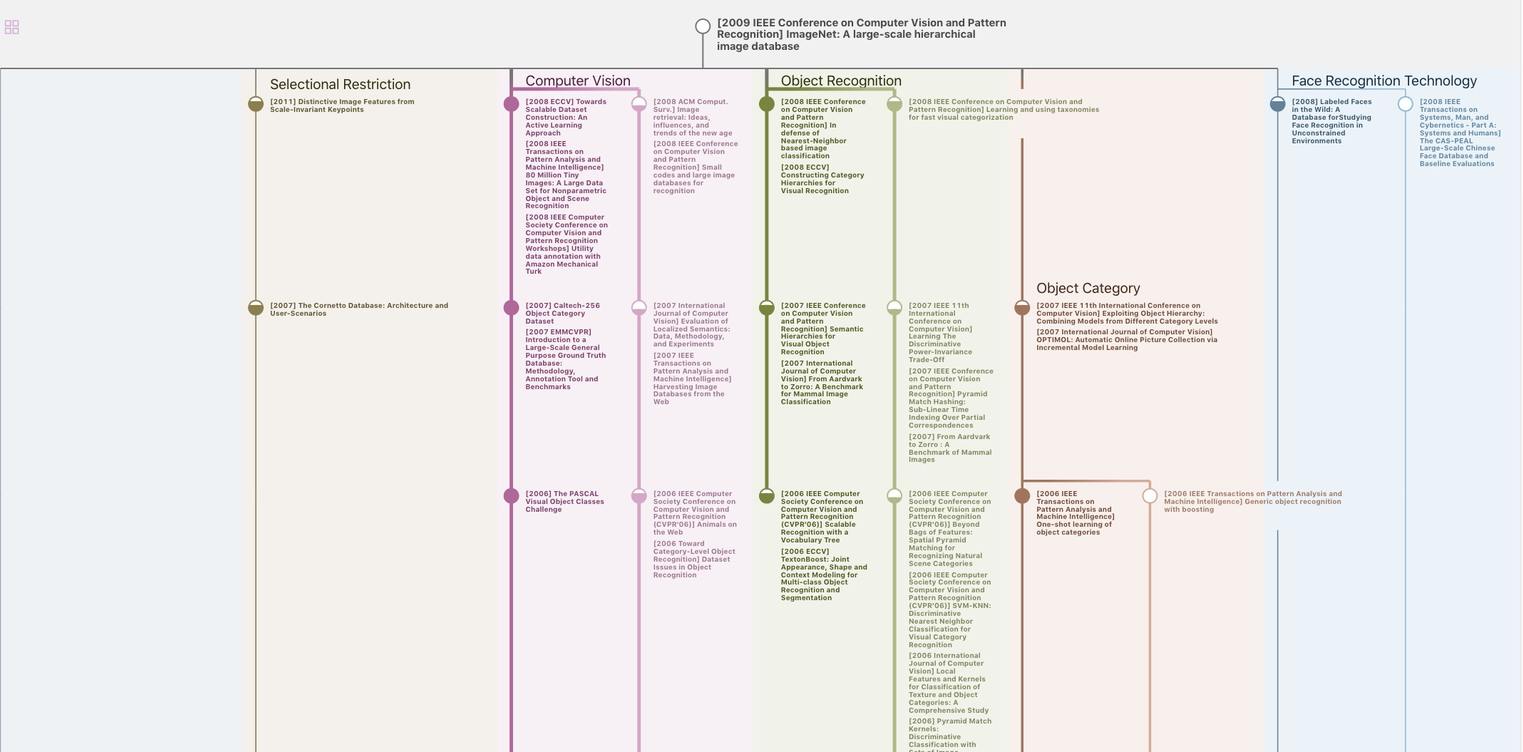
生成溯源树,研究论文发展脉络
Chat Paper
正在生成论文摘要