Multi-Feature Probabilistic Detector Applied to Apnea/Hypopnea Monitoring
CinC(2019)
摘要
Robust, real-time apnea and hypopnea detection for monitoring patients suffering from sleep apnea syndrome (SAS) still represents an open problem due to the effect of noise artifacts, the complexity of respiratory patterns and inter-subject variability. We propose in this study the application of an original multi-feature probabilistic detector (MFPD) for SAS event detection during long-term monitoring recordings on three SAS patients. The nasal pressure signal is used as input to derive a set of respiratory features (variance, peak-to-peak amplitude and total respiration cycle) which are statistically characterized during time and used to provide a mono-feature detection probability in realtime. A centralized fusion approach based on the Kullback-Leibler divergence (KLD), optimally combines these mono-feature distributions in order to produce a final detection. While the optimal feature set selection lies beyond the scope of our study, we illustrate the ability to adapt each feature’s weight dynamically to make centralized fusion decisions. The method can be directly applied to data acquired from multiple sensors as long as features are synchronized. Our proposed fusion method achieves a very high sensitivity (94%) as compared with reference thresholding based methods in the literature.
更多查看译文
关键词
nasal pressure signal,respiratory features,peak-to-peak amplitude,total respiration cycle,centralized fusion approach,Kullback-Leibler divergence,optimal feature set selection,centralized fusion decisions,real-time apnea,hypopnea detection,monitoring patients,sleep apnea syndrome,noise artifacts,respiratory patterns,SAS event detection,long-term monitoring recordings,SAS patients,monofeature distributions,monofeature detection probability,multifeature probabilistic detector
AI 理解论文
溯源树
样例
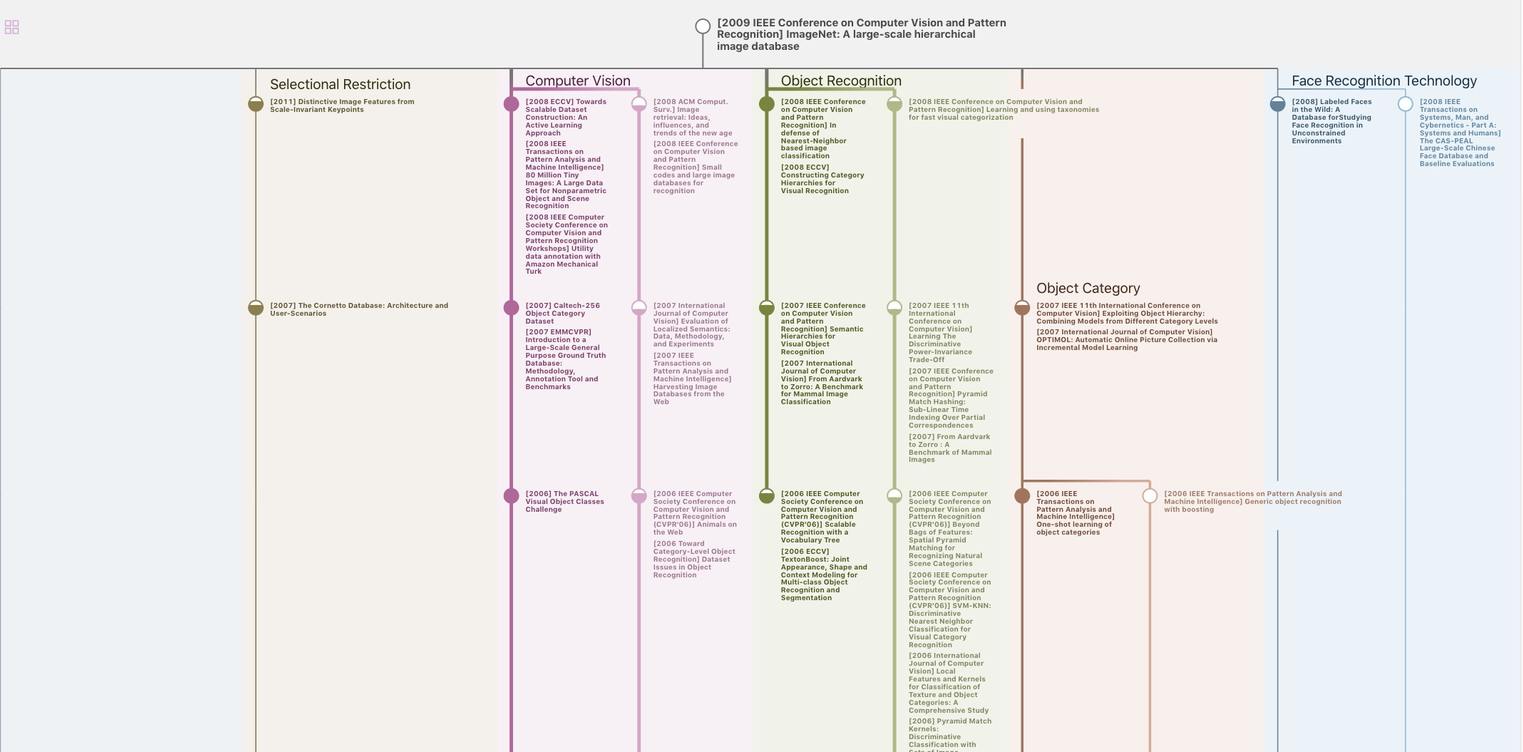
生成溯源树,研究论文发展脉络
Chat Paper
正在生成论文摘要