Pay Attention and Watch Temporal Correlation: A Novel 1-D Convolutional Neural Network for ECG Record Classification
CinC(2019)
Abstract
Currently, many researches focus on the classification of heart beats. It is rare to study the classification of the entire ECG records. In fact, these two issues are completely different, record level classification is more difficult than beat level classification. In this paper, we propose a record level ECG classification method by combining 1-D deep convolutional neural network (CNN) and long short-term memory network (LSTM). The proposed method utilizes CNN to automatic extract features of ECG patches without complex data pre-processing or artificial feature scheme, and then utilizes two layers LSTM to model the underlying temporal correlation relation among ECG patches. In order to alleviate vanishing gradient problem and enhance representation power of features, we combine residual connection and attention mechanisms to build a residual attention module. Stacked residual attention modules not only deepen the network, but also refine the feature maps from both spatial and channel dimensions. We validate the proposed method through the dataset provide by The China Physiological Signal Challenge 2018 (6,877 ECG records, 9 classes), the cross-validation result of F1 score is 0.78. Experiments on PhysioNet/CinC 2017 (8,528 ECG records, 4 classes) reveal that the F1 score is 0.809.
MoreTranslated text
Key words
1D convolutional neural network,ECG record classification,heart beats,entire ECG records,record level classification,beat level classification,record level ECG classification method,CNN,long short-term memory network,ECG patches,complex data preprocessing,artificial feature scheme,layers LSTM,residual attention module,stacked residual attention modules,feature maps,ECG records,temporal correlation relation
AI Read Science
Must-Reading Tree
Example
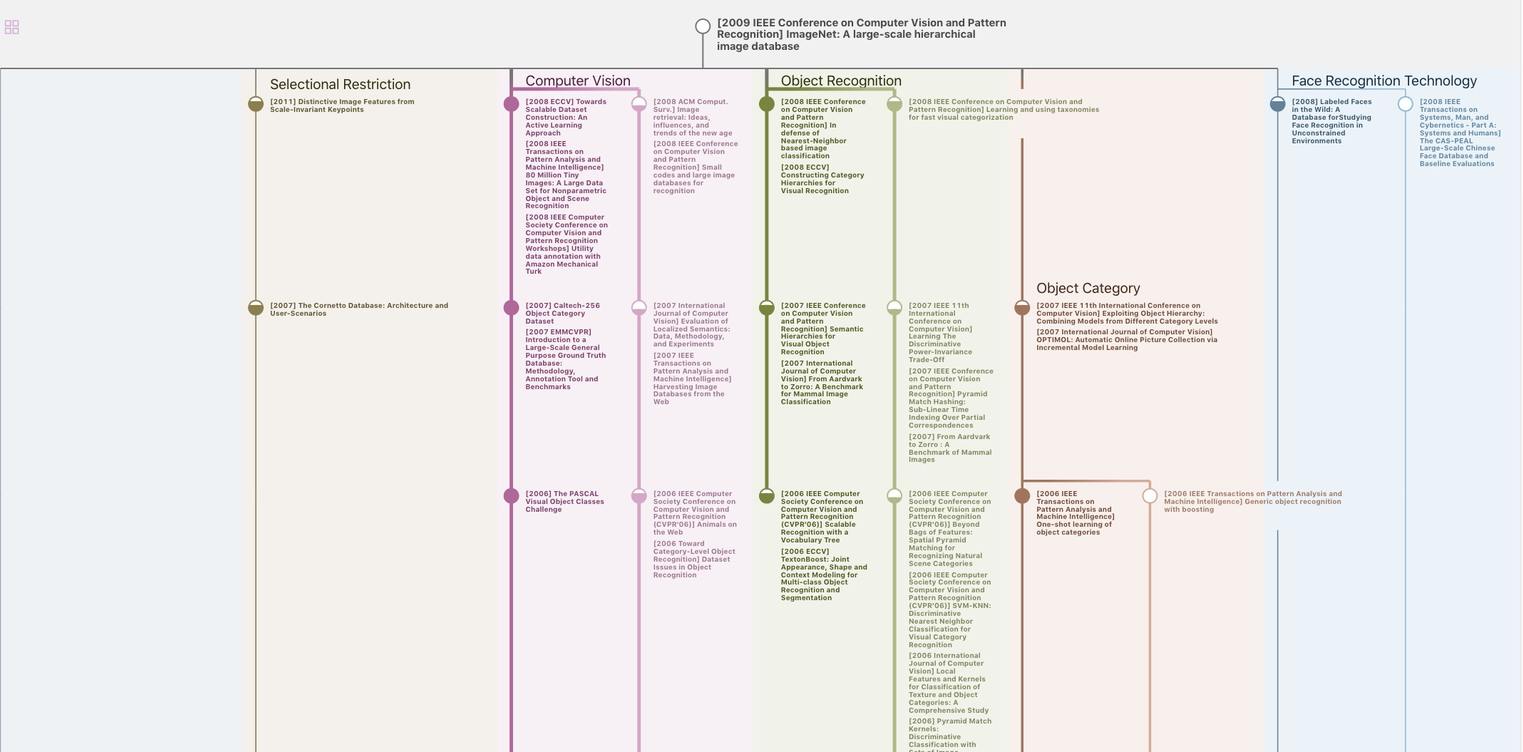
Generate MRT to find the research sequence of this paper
Chat Paper
Summary is being generated by the instructions you defined