Cloud and Cloud Shadow Detection Based on Multiscale 3D-CNN for High Resolution Multispectral Imagery
IEEE ACCESS(2020)
摘要
Cloud and cloud shadow detection is one of the most important tasks for optical remote sensing image preprocessing. It is not an easy task due to the variety and complexity of underlying surfaces, such as the low-albedo objects (water and mountain shadow) and the high-albedo objects (snow and ice). In this study, an end-to-end multiscale 3D-CNN method is proposed for cloud and cloud shadow detection in high resolution multispectral imagery. Specifically, a multiscale learning module is designed to extract cloud and cloud shadow contextual information of different levels. In order to make full use of band information, four band-combination images are inputted into the multiscale 3D-CNN. A joint spectral-spatial information of 3D-convolution layer is developed to fully explore the joint spatial-spectral correlations feature in the input data. Overall, in the experiments undertaken in this paper, the proposed method achieved a mean overall accuracy of 97.27 & x0025; for cloud detection, with a mean precision of 96.02 & x0025; and a mean recall of 95.86 & x0025;. For cloud shadow detection, the proposed method achieved a mean precision of 95.92 & x0025; and a mean recall of 92.86 & x0025;. Experimental results on two validation datasets (GF-1 WFV validation data and ZY-3 validation data) show that the proposed multiscale-3D-CNN method achieved good performance with limited spectral ranges.
更多查看译文
关键词
Cloud detection,cloud shadow,convolution neural networks,multiscale 3D-CNN
AI 理解论文
溯源树
样例
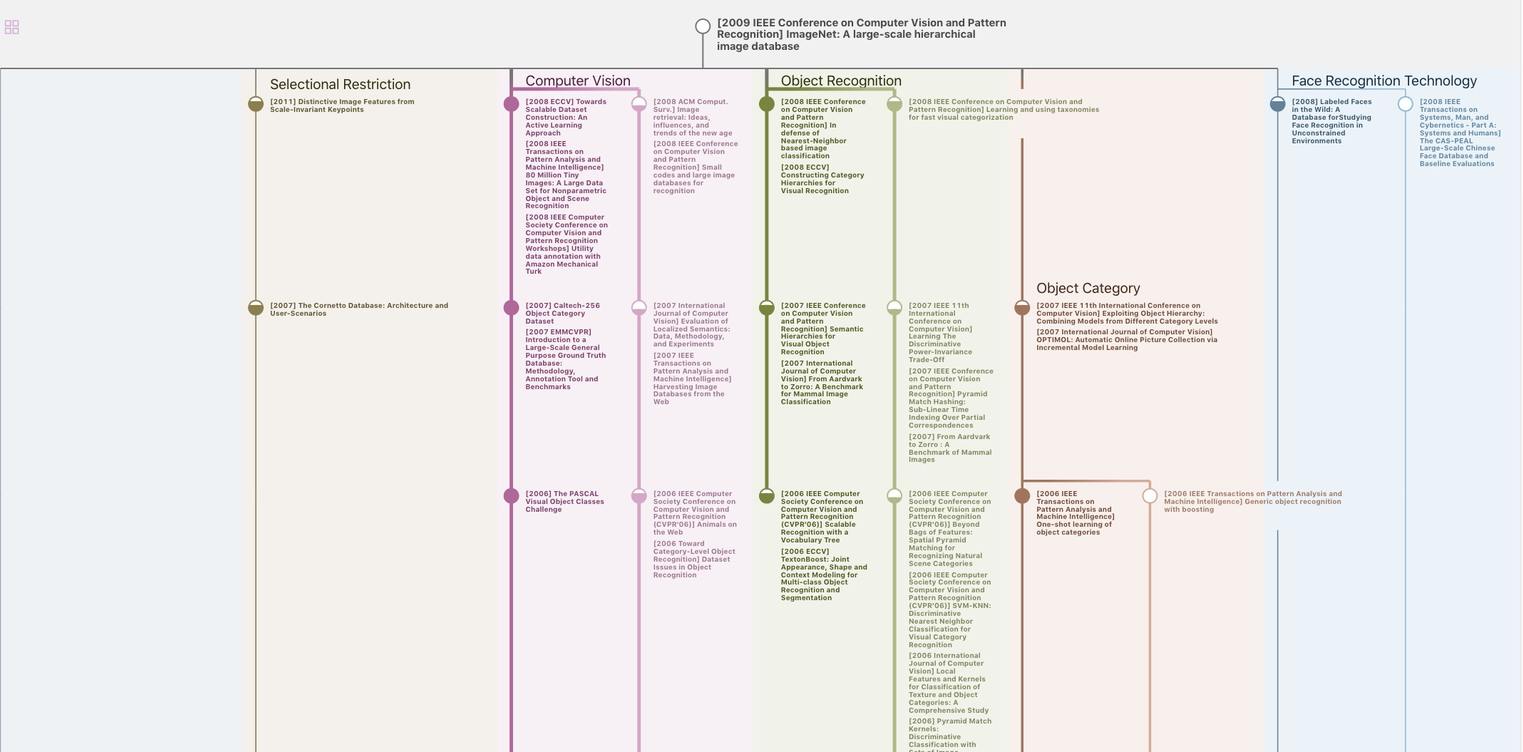
生成溯源树,研究论文发展脉络
Chat Paper
正在生成论文摘要