On the combination of kernel principal component analysis and neural networks for process indirect control
MATHEMATICAL AND COMPUTER MODELLING OF DYNAMICAL SYSTEMS(2020)
摘要
A new adaptive kernel principal component analysis (KPCA) for non-linear discrete system control is proposed. The proposed approach can be treated as a new proposition for data pre-processing techniques. Indeed, the input vector of neural network controller is pre-processed by the KPCA method. Then, the obtained reduced neural network controller is applied in the indirect adaptive control. The influence of the input data pre-processing on the accuracy of neural network controller results is discussed by using numerical examples of the cases of time-varying parameters of single-input single-output non-linear discrete system and multi-input multi-output system. It is concluded that, using the KPCA method, a significant reduction in the control error and the identification error is obtained. The lowest mean squared error and mean absolute error are shown that the KPCA neural network with the sigmoid kernel function is the best.
更多查看译文
关键词
Neural networks,modelling,indirect control,KPCA,reduction,non-linear system
AI 理解论文
溯源树
样例
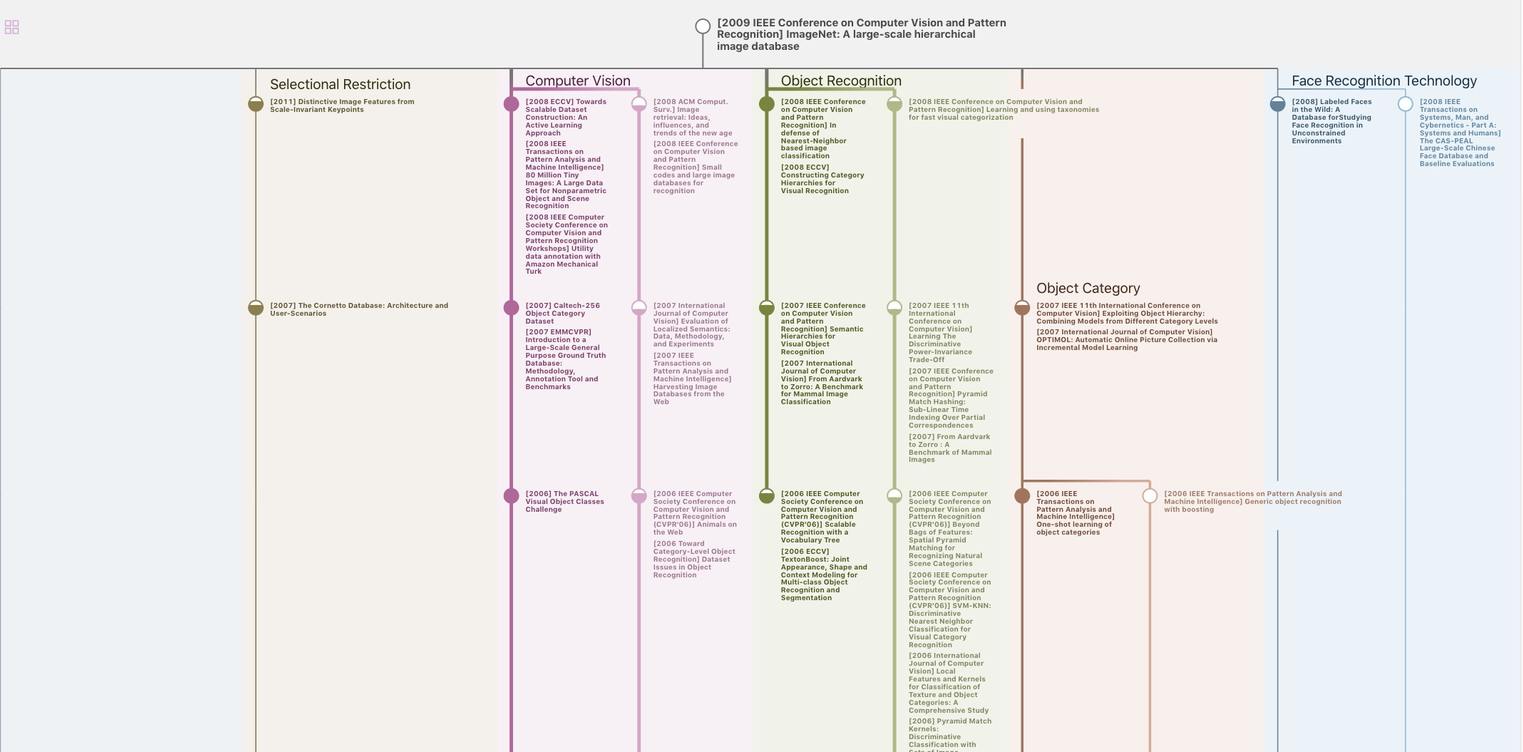
生成溯源树,研究论文发展脉络
Chat Paper
正在生成论文摘要