RNN-Based Subway Passenger Flow Rolling Prediction
IEEE ACCESS(2020)
摘要
The subway station passenger flow prediction model can forecast passenger volume in the future. This model helps to carry out safety warnings and evacuation of passenger flow in advance. Based on the data of the Shanghai traffic card, the passenger volume in all the time intervals is clustered into three different models for prediction. Taking the Nanjing East Road Station in Shanghai as an example, the time series of passenger volumes was combined with weather data to create several supervised sequences and was converted to supervised sequences according to different values of timestep. To accelerate convergence, two artificial features were added as input. The gated recurrent unit (GRU) network model achieves accurate rolling prediction from 15 minutes to 6 hours. Finally, comparing it with the long short-term memory (LSTM) network and the back-forward propagation network (BPN), it was confirmed that the GRU network with a timestep of 1.5 hours is the best model for the long-term (more than 3 hours) traffic flow rolling prediction, while GRU with a timestep of 45 minutes has the best result for short-term rolling prediction.
更多查看译文
关键词
Data analysis,time series analysis,predictive models,neural networks,LSTM,GRU
AI 理解论文
溯源树
样例
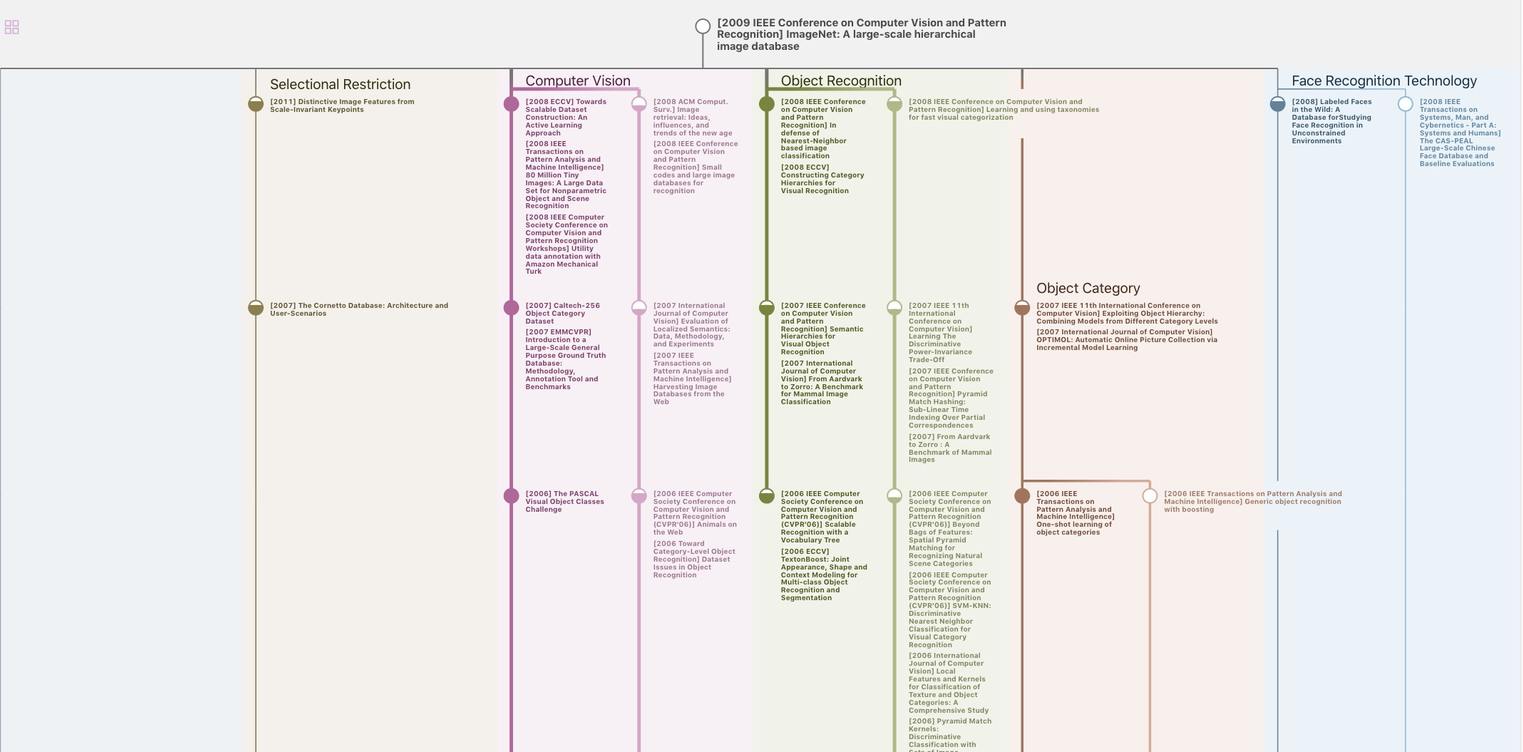
生成溯源树,研究论文发展脉络
Chat Paper
正在生成论文摘要