A novel density-based clustering algorithm using nearest neighbor graph
PATTERN RECOGNITION(2020)
Abstract
Density-based clustering has several desirable properties, such as the abilities to handle and identify noise samples, discover clusters of arbitrary shapes, and automatically discover of the number of clusters. Identifying the core samples within the dense regions of a dataset is a significant step of the density-based clustering algorithm. Unlike many other algorithms that estimate the density of each samples using different kinds of density estimators and then choose core samples based on a threshold, in this paper, we present a novel approach for identifying local high-density samples utilizing the inherent properties of the nearest neighbor graph (NNG). After using the density estimator to filter noise samples, the proposed algorithm ADBSCAN in which "A" stands for "Adaptive" performs a DBSCAN-like clustering process. The experimental results on artificial and real-world datasets have demonstrated the significant performance improvement over existing density-based clustering algorithms. (C) 2020 Elsevier Ltd. All rights reserved.
MoreTranslated text
Key words
Density-based clustering,Nearest neighbor graph,DBSCAN
AI Read Science
Must-Reading Tree
Example
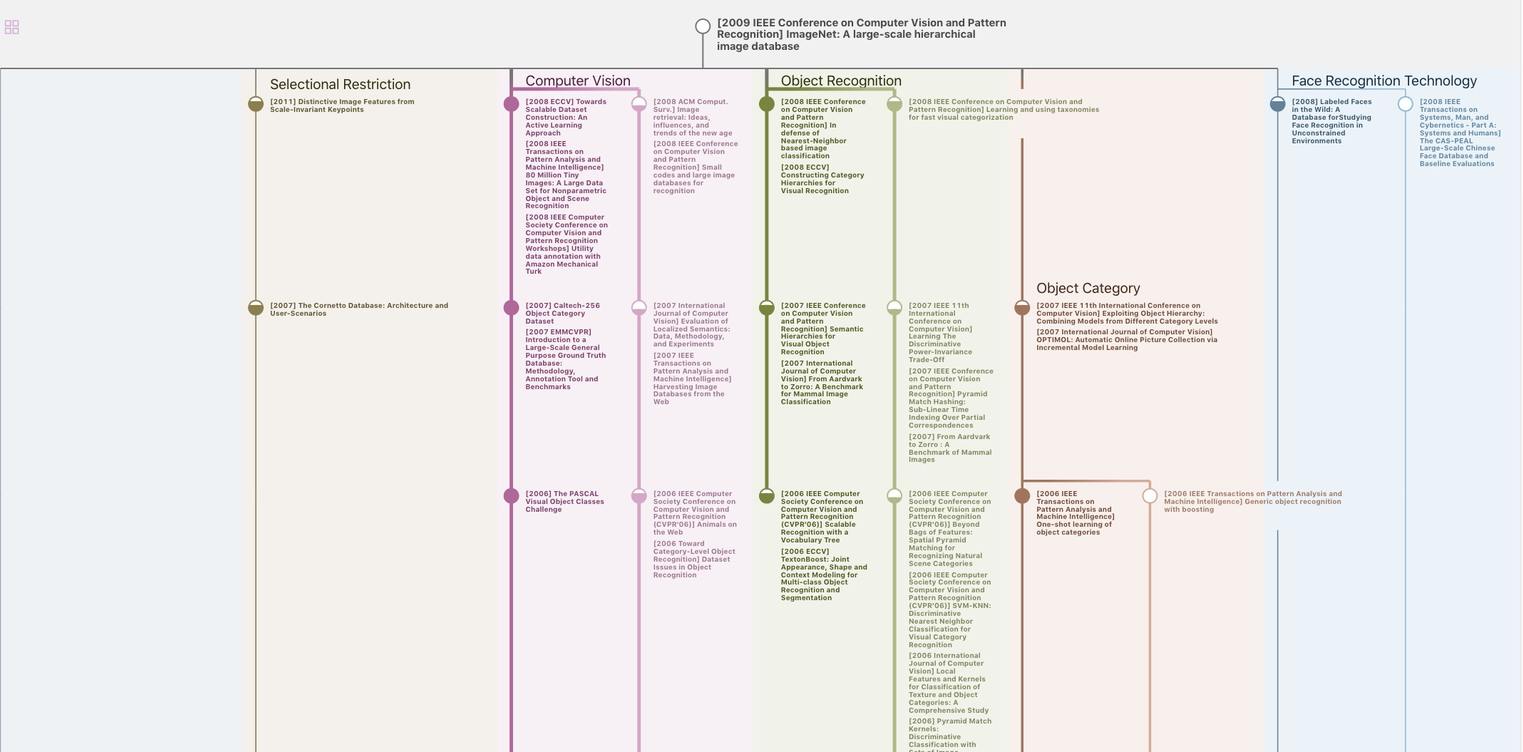
Generate MRT to find the research sequence of this paper
Chat Paper
Summary is being generated by the instructions you defined