Robust Nonnegative Mixed-Norm Algorithm With Weighted L(1)-Norm Regularization
CONFERENCE PROCEEDINGS OF 2019 IEEE INTERNATIONAL CONFERENCE ON SIGNAL PROCESSING, COMMUNICATIONS AND COMPUTING (IEEE ICSPCC 2019)(2019)
Abstract
Nonnegativity as a constraint has attracted much attention in recent years. The recently proposed sign-sign nonnegative least-mean-square (NNLMS) algorithm is robust against impulsive noise, but it suffers from slow convergence speed. In this paper we propose a new nonnegative mixednorm algorithm with faster convergence speed than sign-sign NNLMS. To further improve the convergence speed for estimating sparse systems with nonnegativity constrains, we apply the weighted l(1)-norm of the adaptive filter weight vector as a regularization term to the cost function of the estimation error. Simulation results are provided to illustrate the superior performance.
MoreTranslated text
Key words
adaptive filtering, robustness, weighted l(1)-norm, mixed-norm, nonnegative constraint
AI Read Science
Must-Reading Tree
Example
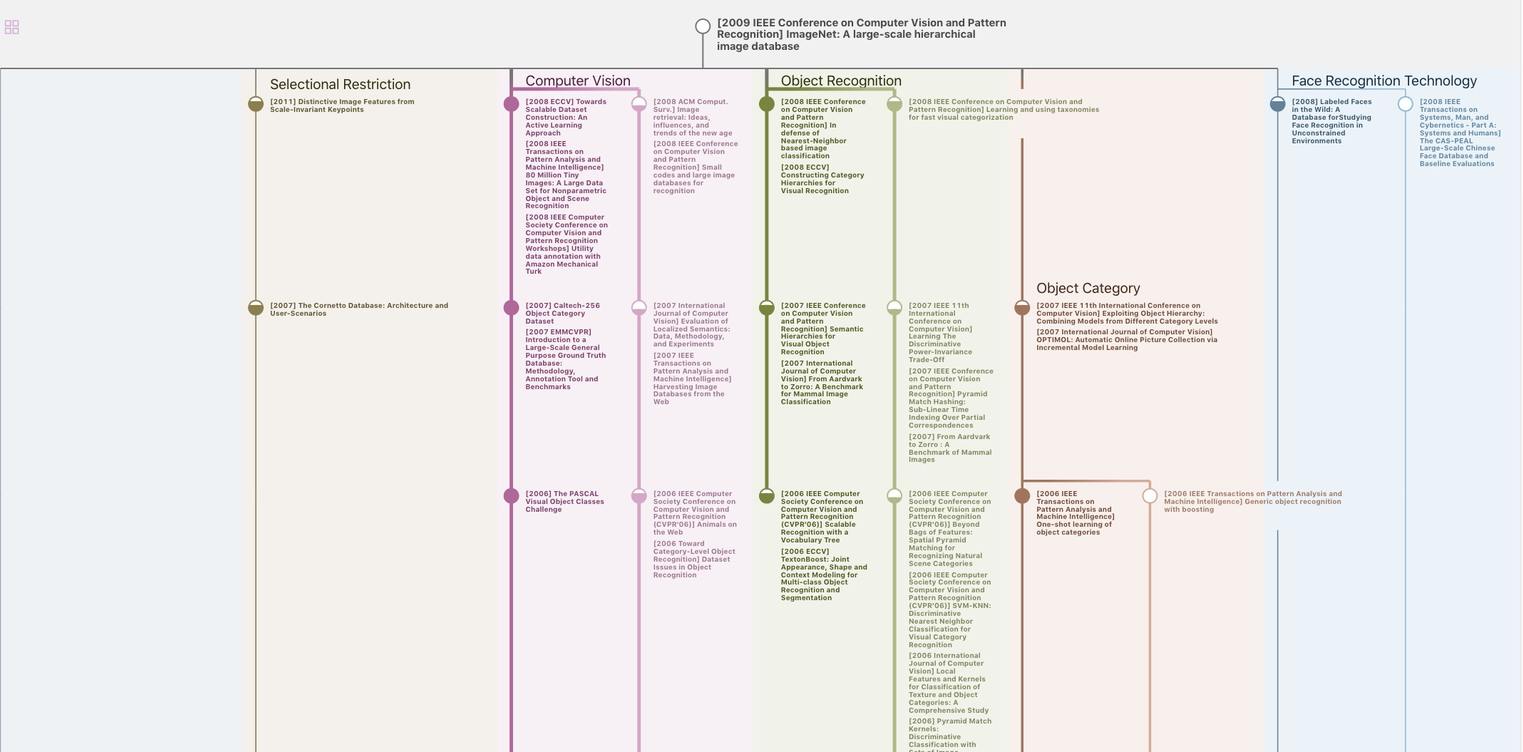
Generate MRT to find the research sequence of this paper
Chat Paper
Summary is being generated by the instructions you defined