Analysis of non-iterative phase retrieval based on machine learning
Optical Review(2020)
摘要
In this paper, we analyze a machine-learning-based non-iterative phase retrieval method. Phase retrieval and its applications have been attractive research topics in optics and photonics, for example, in biomedical imaging, astronomical imaging, and so on. Most conventional phase retrieval methods have used iterative processes to recover phase information; however, the calculation speed and convergence with these methods are serious issues in real-time monitoring applications. Machine-learning-based methods are promising for addressing these issues. Here, we numerically compare conventional methods and a machine-learning-based method in which a convolutional neural network is employed. Simulations with several conditions show that the machine-learning-based method realizes fast and robust phase recovery compared with the conventional methods. We also numerically demonstrate machine-learning-based phase retrieval from noisy measurements with a noisy training data set for improving the noise robustness. The machine-learning-based approach used in this study may increase the impact of phase retrieval, which is useful in various fields, where phase retrieval has been used as a fundamental tool.
更多查看译文
关键词
Phase retrieval, Machine learning, Deep learning, Convolutional neural network, Inverse problem
AI 理解论文
溯源树
样例
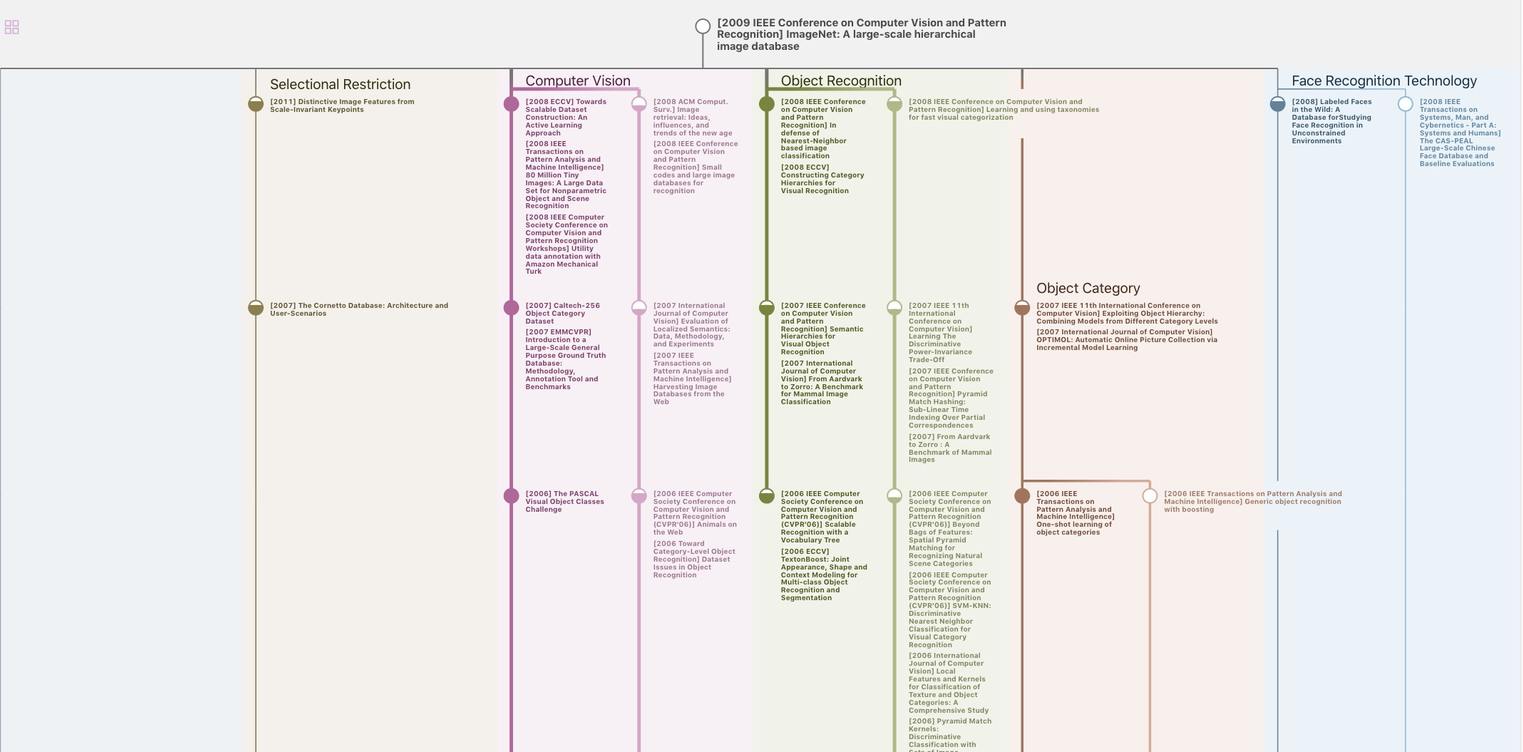
生成溯源树,研究论文发展脉络
Chat Paper
正在生成论文摘要