A Case Study On A Sustainable Framework For Ethically Aware Predictive Modeling
2019 IEEE INTERNATIONAL SYMPOSIUM ON TECHNOLOGY AND SOCIETY (ISTAS)(2019)
摘要
Large volumes of data allow for modern application of statistical and mathematical models to practical social issues. Many applications of predictive models like criminal activity heat mapping, recidivism estimation, and child safety scoring rely on data that may be incomplete, incorrect, or biased. Many sensitive social and historical issues can unintentionally be incorporated into predictions causing ethical mistreatment. This work proposes a mechanism for continuously mitigating model bias by using algorithms that produce predictions from reasonably small subsets of data, allowing a human-in-the-loop approach to model application. The benefits offered by this framework are twofold: (1) bias can be identified either statistically or by human users on a per-prediction basis; (2) data can be cleaned for bias on a per-prediction basis. A modeling and data management methodology similar to that presented here could strengthen the ethical application of data science and make the process of cleaning and validating data manageable in the long term.
更多查看译文
关键词
ethics, predictive models, multi-layer perceptron, decision tree, nearest neighbor, framework, human-in-the-loop
AI 理解论文
溯源树
样例
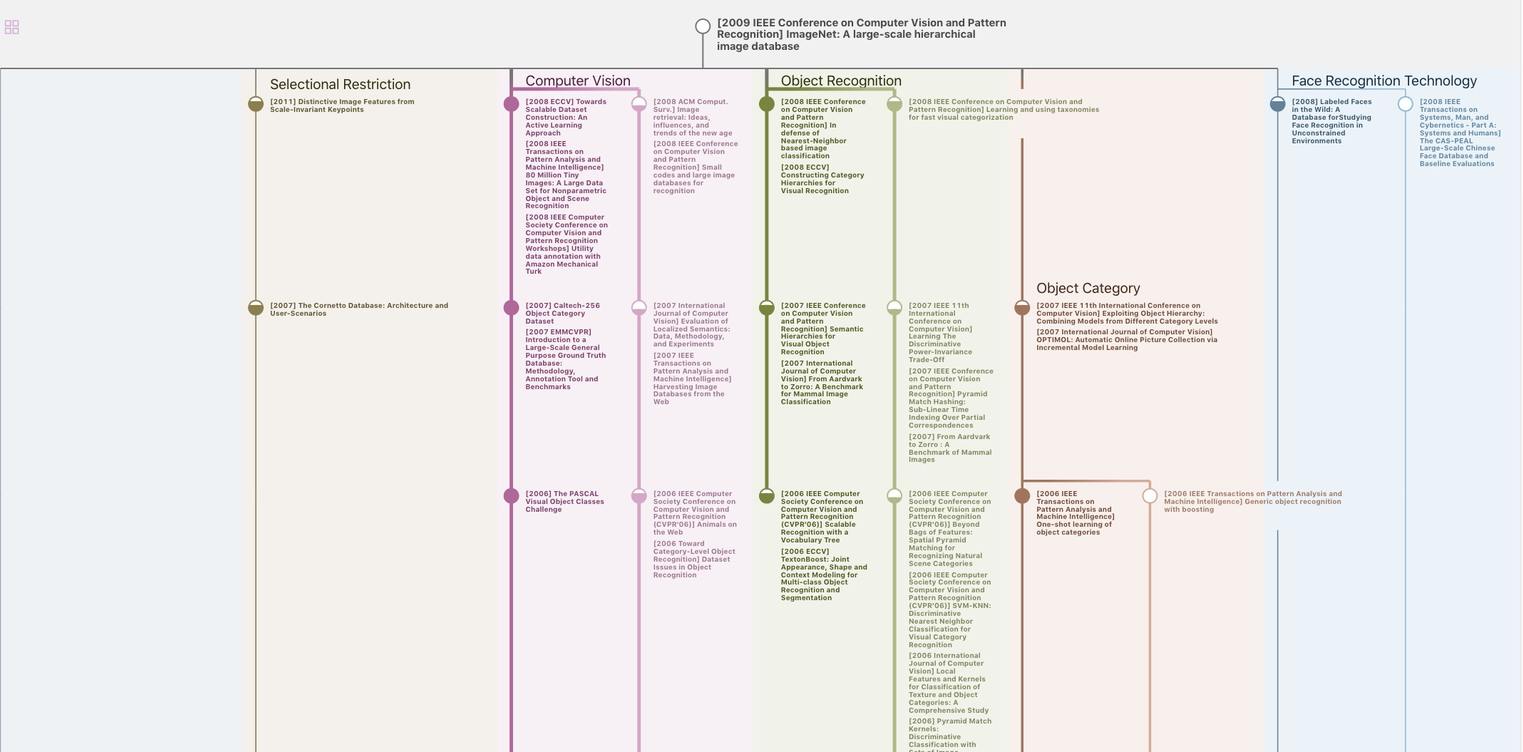
生成溯源树,研究论文发展脉络
Chat Paper
正在生成论文摘要