A CNN-Based Multi-scale Super-Resolution Architecture on FPGA for 4K/8K UHD Applications
MULTIMEDIA MODELING (MMM 2020), PT II(2020)
摘要
In this paper, based on our previous work, we present a multi-scale super-resolution (SR) hardware (HW) architecture using a convolutional neural network (CNN), where the up-scaling factors of 2, 3 and 4 are supported. In our dedicated multi-scale CNN-based SR HW, low-resolution (LR) input frames are processed line-by-line, and the number of convolutional filter parameters is significantly reduced by incorporating depth-wise separable convolutions with residual connections. As for 3× and 4× up-scaling, the number of channels for point-wise convolution layer before a pixel-shuffle layer is set to 9 and 16, respectively. Additionally, we propose an integrated timing generator that supports 3× and 4× up-scaling. For efficient HW implementation, we use a simple and effective quantization method with a minimal peak signal-to-noise ratio (PSNR) degradation. Also, we propose a compression method to efficiently store intermediate feature map data to reduce the number of line memories used in HW. Our CNN-based SR HW implementation on the FPGA can generate 4K ultra high-definition (UHD) frames of higher PSNR at 60 fps, which have higher visual quality compared to conventional CNN-based SR methods that were trained and tested in software. The resources in our CNN-based SR HW can be shared for multi-scale upscaling factors of 2, 3 and 4 so that can be implemented to generate 8K UHD frames from 2K FHD input frames.
更多查看译文
关键词
Super-resolution,Multi-scale,4 K UHD,Deep learning,CNN,Real-time,Hardware,FPGA
AI 理解论文
溯源树
样例
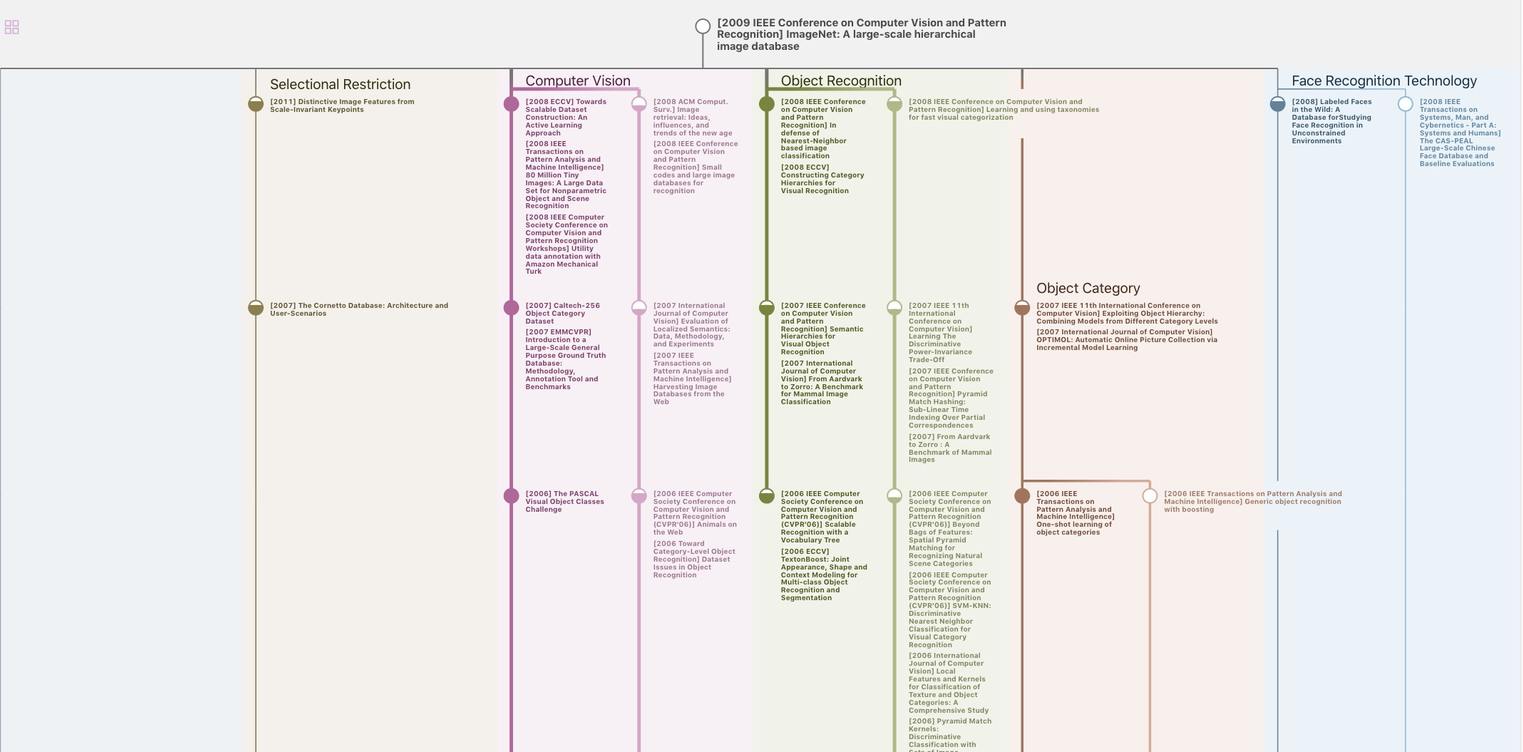
生成溯源树,研究论文发展脉络
Chat Paper
正在生成论文摘要