Robust tensor decomposition via orientation invariant tubal nuclear norms
THIRTY-FOURTH AAAI CONFERENCE ON ARTIFICIAL INTELLIGENCE, THE THIRTY-SECOND INNOVATIVE APPLICATIONS OF ARTIFICIAL INTELLIGENCE CONFERENCE AND THE TENTH AAAI SYMPOSIUM ON EDUCATIONAL ADVANCES IN ARTIFICIAL INTELLIGENCE(2022)
Abstract
Aiming at recovering an unknown tensor (i.e., multi-way array) corrupted by both sparse outliers and dense noises, robust tensor decomposition (RTD) serves as a powerful pre-processing tool for subsequent tasks like classification and target detection in many computer vision and machine learning applications. Recently, tubal nuclear norm (TNN) based optimization is proposed with superior performance as compared with other tensorial nuclear norms for tensor recovery. However, one major limitation is its orientation sensitivity due to low-rankness strictly defined along tubal orientation and it cannot simultaneously model spectral low-rankness in multiple orientations. To this end, we introduce two new tensor norms called OITNN-O and OITNN-L to exploit multi-orientational spectral low-rankness for an arbitrary K -way (K ≥ 3) tensors. We further formulate two RTD models via the proposed norms and develop two algorithms as the solutions. Theoretically, we establish non-asymptotic error bounds which can predict the scaling behavior of the estimation error. Experiments on real-world datasets demonstrate the superiority and effectiveness of the proposed norms.
MoreTranslated text
Key words
tensor recovery,t-SVD,estimation error,tensor completion
AI Read Science
Must-Reading Tree
Example
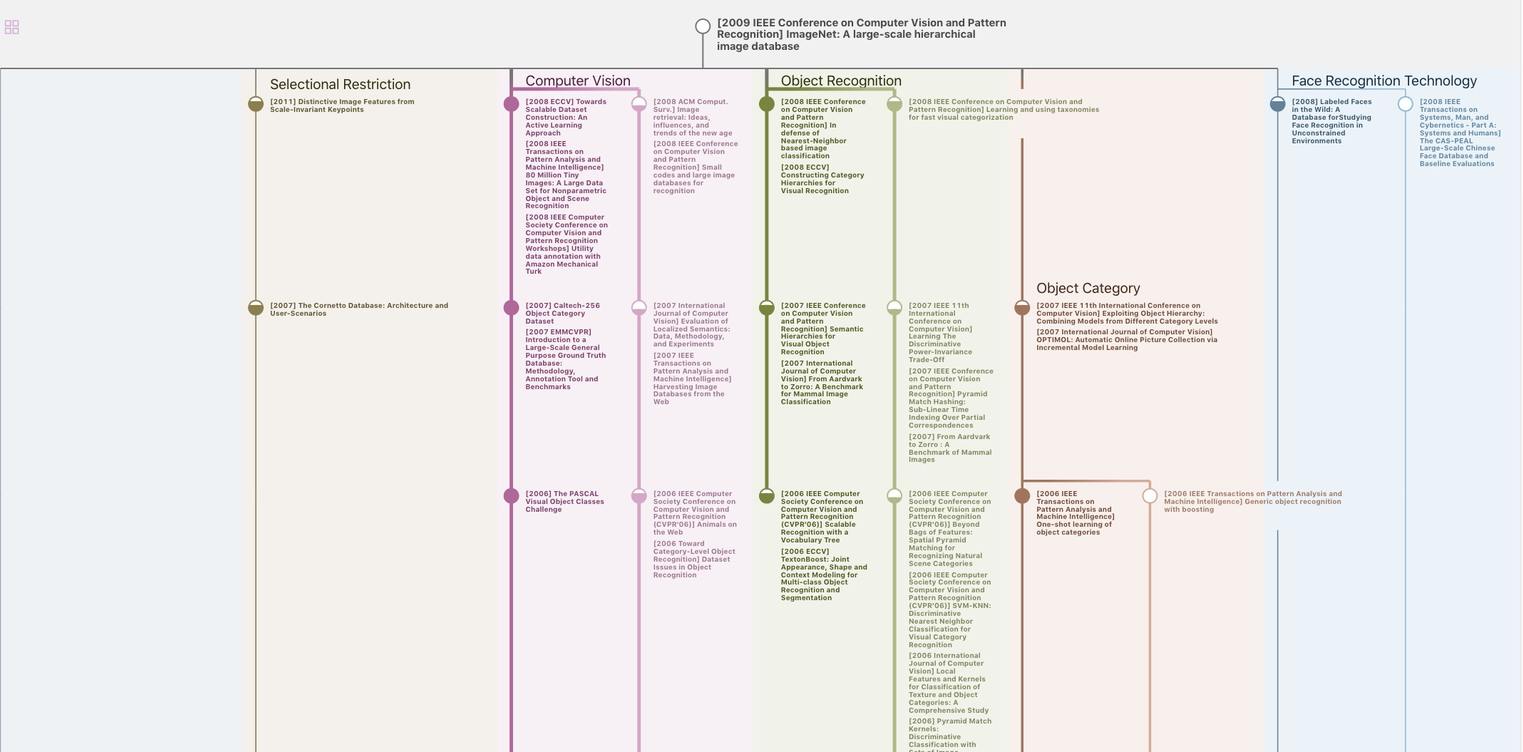
Generate MRT to find the research sequence of this paper
Chat Paper
Summary is being generated by the instructions you defined