Heterogeneous Transfer Learning With Weighted Instance-Correspondence Data
THIRTY-FOURTH AAAI CONFERENCE ON ARTIFICIAL INTELLIGENCE, THE THIRTY-SECOND INNOVATIVE APPLICATIONS OF ARTIFICIAL INTELLIGENCE CONFERENCE AND THE TENTH AAAI SYMPOSIUM ON EDUCATIONAL ADVANCES IN ARTIFICIAL INTELLIGENCE(2020)
Abstract
Instance-correspondence (IC) data are potent resources for heterogeneous transfer learning (Hell) due to the capability of bridging the source and the target domains at the instance-level. To this end, people tend to use machine-generated IC data, because manually establishing IC data is expensive and primitive. However, existing IC data machine generators are not perfect and always produce the data that are not of high quality, thus hampering the performance of domain adaption. In this paper, instead of improving the IC data generator, which might not be an optimal way. we accept the fact that data quality variation does exist but find a better way to use the data. Specifically, we propose a novel heterogeneous transfer learning method named Transfer Learning with Weighted Correspondence (TLWC), which utilizes IC data to adapt the source domain to the target domain. Rather than treating IC data equally, TLWC can assign solid weights to each IC data pair depending on the quality of the data. We conduct extensive experiments on He datasets and the state-of-the-art results verify the effectiveness of TLWC.
MoreTranslated text
Key words
Transfer Learning,Regression,Incremental Learning,Representation Learning,Meta-Learning
AI Read Science
Must-Reading Tree
Example
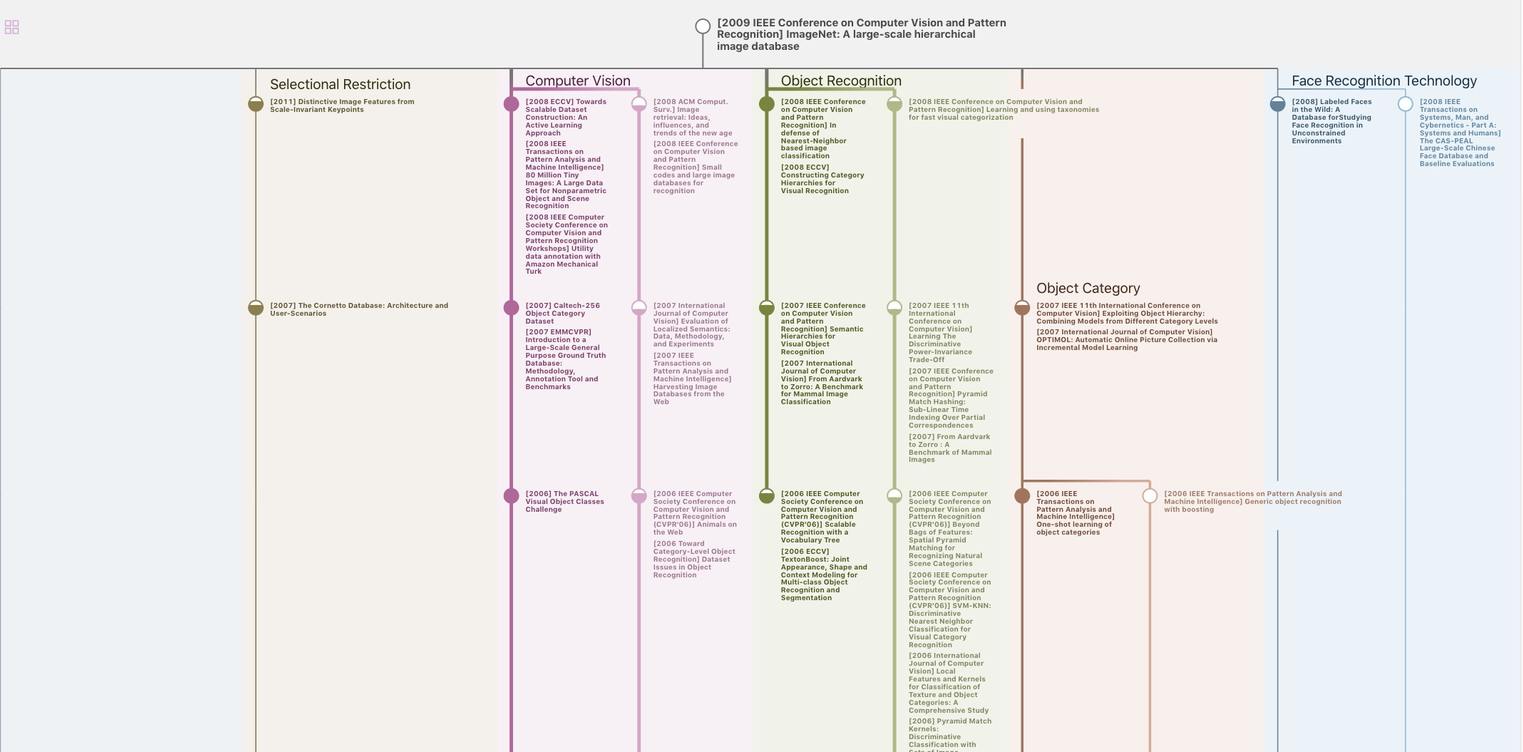
Generate MRT to find the research sequence of this paper
Chat Paper
Summary is being generated by the instructions you defined