Machine Learning Perspective Gene Optimization for Efficient Induction Machine Design
JOURNAL OF ELECTRICAL ENGINEERING & TECHNOLOGY(2018)
摘要
In this paper, induction machine operation efficiency and torque is improved using Machine Learning based Gene Optimization (ML-GO) Technique is introduced. Optimized Genetic Algorithm (OGA) is used to select the optimal induction machine data. In OGA, selection, crossover and mutation process is carried out to find the optimal electrical machine data for induction machine design. Initially, many number of induction machine data are given as input for OGA. Then, fitness value is calculated for all induction machine data to find whether the criterion is satisfied or not through fitness function (i.e., objective function such as starting to full load torque ratio, rotor current, power factor and maximum flux density of stator and rotor teeth). When the criterion is not satisfied, annealed selection approach in OGA is used to move the selection criteria from exploration to exploitation to attain the optimal solution (i.e., efficient machine data). After the selection process, two point crossovers is carried out to select two crossover points within a chromosomes (i.e., design variables) and then swaps two parent's chromosomes for producing two new offspring. Finally, Adaptive Levy Mutation is used in OGA to select any value in random manner and gets mutated to obtain the optimal value. This process gets iterated till finding the optimal value for induction machine design. Experimental evaluation of ML-GO technique is carried out with performance metrics such as torque, rotor current, induction machine operation efficiency and rotor power factor compared to the state-of-the-art works.
更多查看译文
关键词
Induction motor,annealed selection,Adaptive Levy Mutation,two point crossovers,fitness function,optimized genetic algorithm
AI 理解论文
溯源树
样例
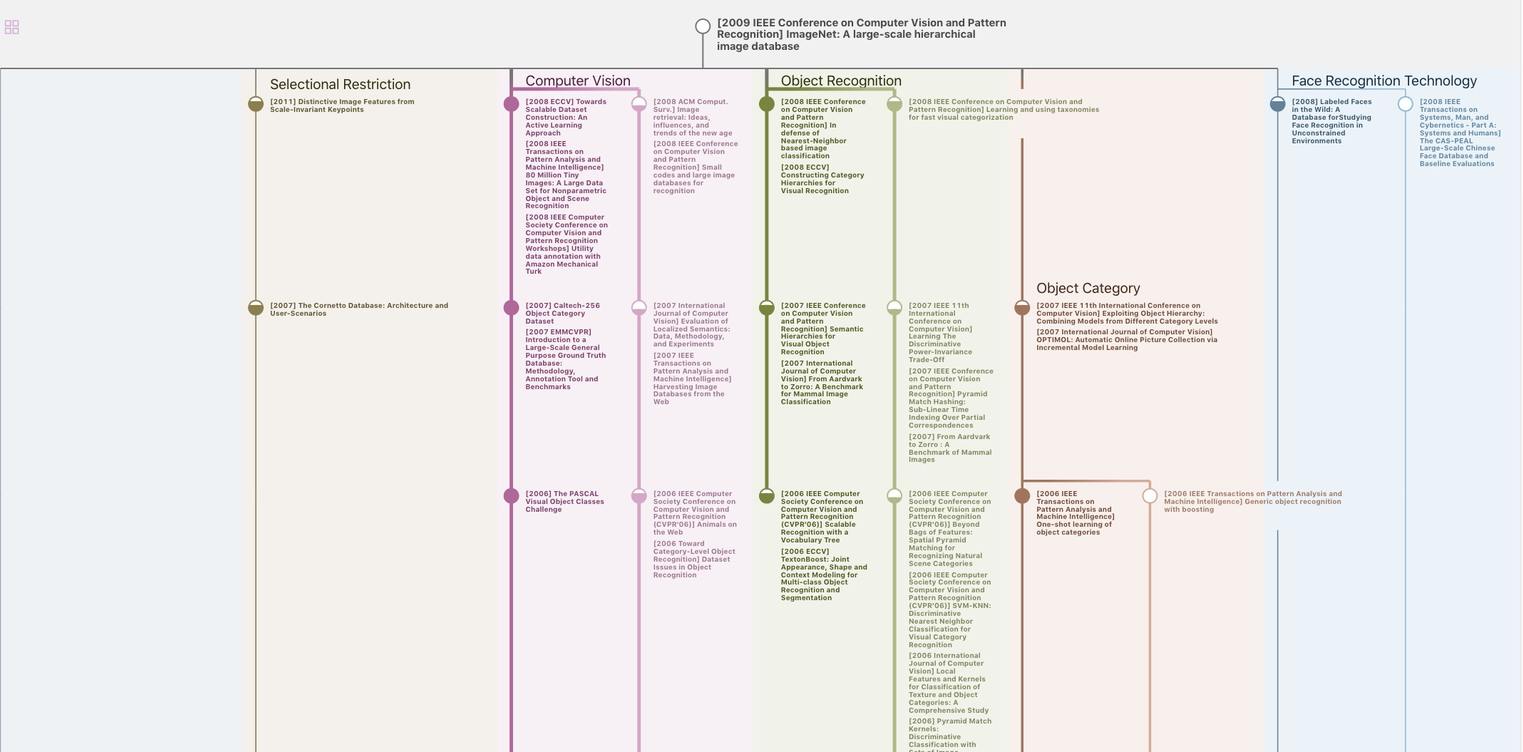
生成溯源树,研究论文发展脉络
Chat Paper
正在生成论文摘要