Feature Fusion Based Deep Spatiotemporal Model For Violence Detection In Videos
NEURAL INFORMATION PROCESSING (ICONIP 2019), PT I(2019)
摘要
It is essential for public monitoring and security to detect violent behavior in surveillance videos. However, it requires constant human observation and attention, which is a challenging task. Autonomous detection of violent activities is essential for continuous, uninterrupted video surveillance systems. This paper proposed a novel method to detect violent activities in videos, using fused spatial feature maps, based on Convolutional Neural Networks (CNN) and Long Short-Term Memory (LSTM) units. The spatial features are extracted through CNN, and multi-level spatial features fusion method is used to combine the spatial features maps from two equally spaced sequential input video frames to incorporate motion characteristics. The additional residual layer blocks are used to further learn these fused spatial features to increase the classification accuracy of the network. The combined spatial features of input frames are then fed to LSTM units to learn the global temporal information. The output of this network classifies the violent or non-violent category present in the input video frame. Experimental results on three different standard benchmark datasets: Hockey Fight, Crowd Violence and BEHAVE show that the proposed algorithm provides better ability to recognize violent actions in different scenarios and results in improved performance compared to the state-of-the-art methods.
更多查看译文
关键词
Violence detection, CNN, LSTM, Autonomous video, Surveillance spatiotemporal features
AI 理解论文
溯源树
样例
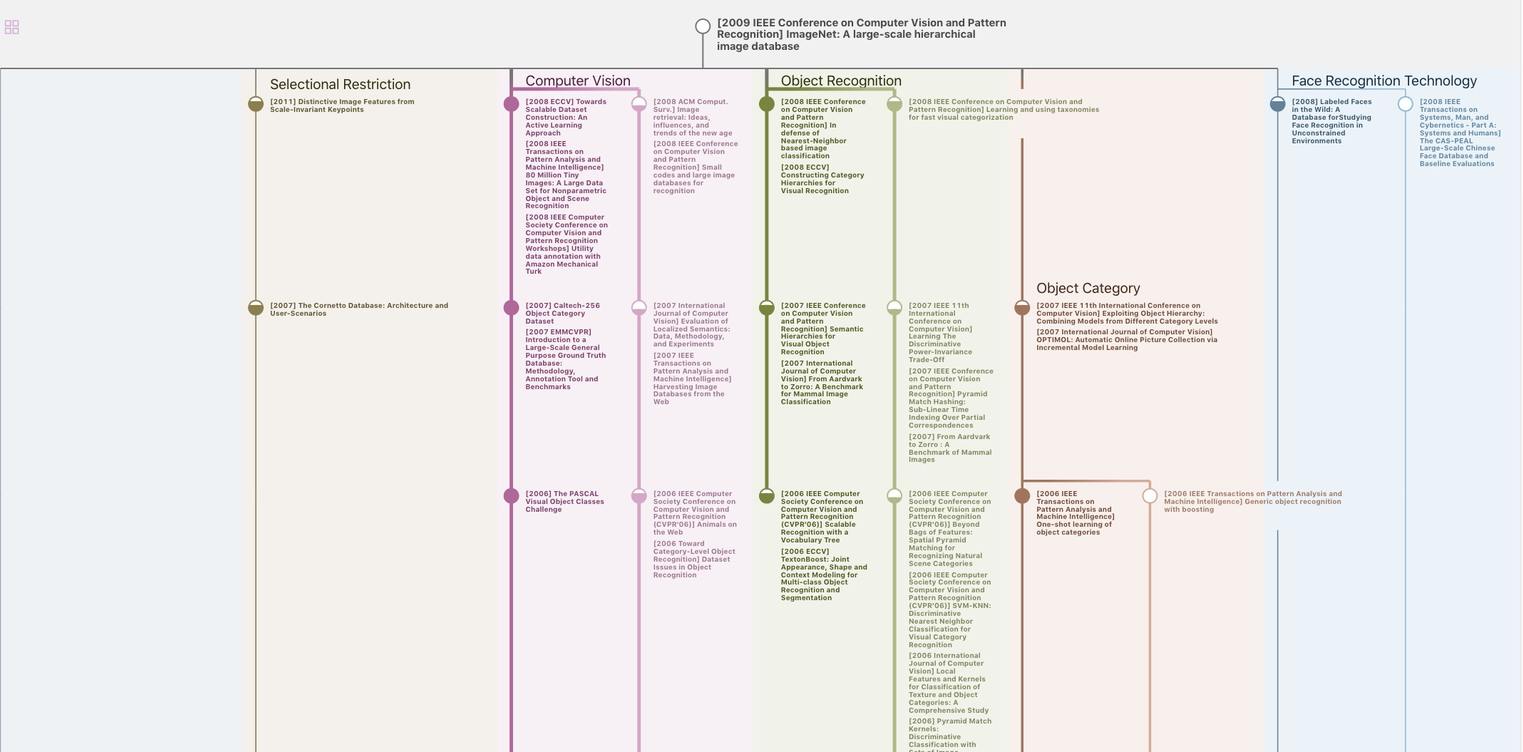
生成溯源树,研究论文发展脉络
Chat Paper
正在生成论文摘要