Per-Host DDoS Mitigation by Direct-Control Reinforcement Learning
IEEE transactions on network and service management/IEEE eTransactions on network and service management(2020)
Abstract
DDoS attacks plague the availability of online services today, yet like many cybersecurity problems are evolving and non-stationary. Normal and attack patterns shift as new protocols and applications are introduced, further compounded by burstiness and seasonal variation. Accordingly, it is difficult to apply machine learning-based techniques and defences in practice. Reinforcement learning (RL) may overcome this detection problem for DDoS attacks by managing and monitoring consequences; an agent’s role is to learn to optimise performance criteria (which are always available) in an online manner. We advance the state-of-the-art in RL-based DDoS mitigation by introducing two agent classes designed to act on a per-flow basis, in a protocol-agnostic manner for any network topology. This is supported by an in-depth investigation of feature suitability and empirical evaluation. Our results show the existence of flow features with high predictive power for different traffic classes, when used as a basis for feedback-loop-like control. We show that the new RL agent models can offer a significant increase in goodput of legitimate TCP traffic for many choices of host density.
MoreTranslated text
Key words
Security services,distributed denial-of-service,software-defined networking,machine learning,reinforcement learning
AI Read Science
Must-Reading Tree
Example
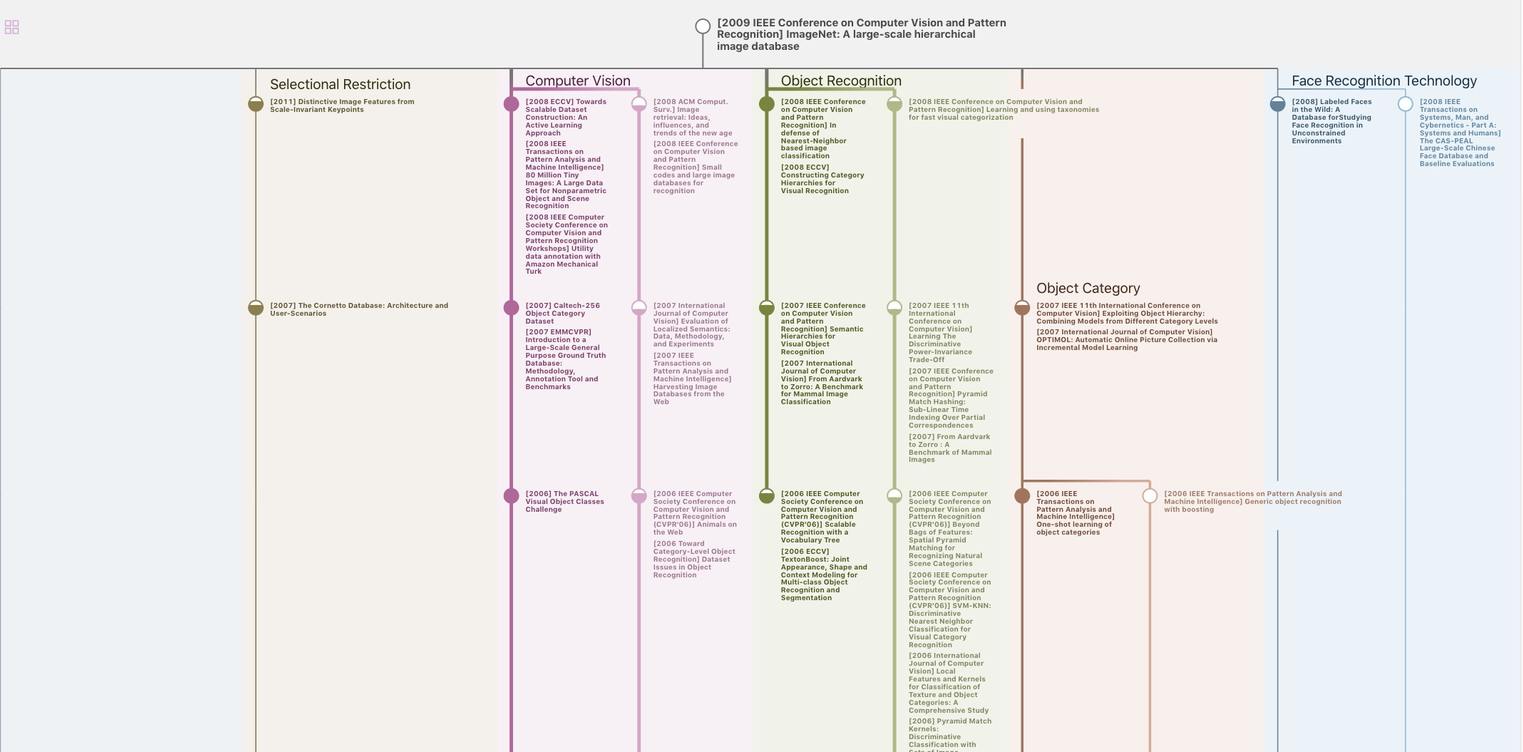
Generate MRT to find the research sequence of this paper
Chat Paper
Summary is being generated by the instructions you defined