DEGAN: De-Expression Generative Adversarial Network for Expression-Invariant Face Recognition by Robot Vision
2019 WRC Symposium on Advanced Robotics and Automation (WRC SARA)(2019)
摘要
Face Recognition is an efficient biometric technique to recognize individuals from images or videos. Since the introduction of face recognition, remarkable achievements have been made in academic researches and commercial applications. However, facial expression variations is one of the remaining challenges for recognition. Because existing face databases almost only store neutral faces, the variations in facial expressions can substantially degrade the recognition performance. It is especially the case for robots since the collected facial images from objects are more likely to contain various expressions in contrast to scenarios such as security check at the airport. Thus, for robot applications, ensuring the performance of expression-invariant face recognition is quite important. The key to expression-invariant face recognition is to eliminate the interference of expression variations. Therefore, we propose a GAN-based method called De-Expression Generative Adversarial Network (DEGAN). DEGAN improves the recognition performance by transforming the facial image with expression to its corresponding neutral one. Detailed experiments are conducted to verify the effectiveness of our proposed method.
更多查看译文
关键词
recognition performance,expression-invariant face recognition,De-Expression Generative Adversarial Network,facial expression variations,face databases,neutral faces,robot vision,efficient biometric technique,collected facial images,GAN-based method,DEGAN
AI 理解论文
溯源树
样例
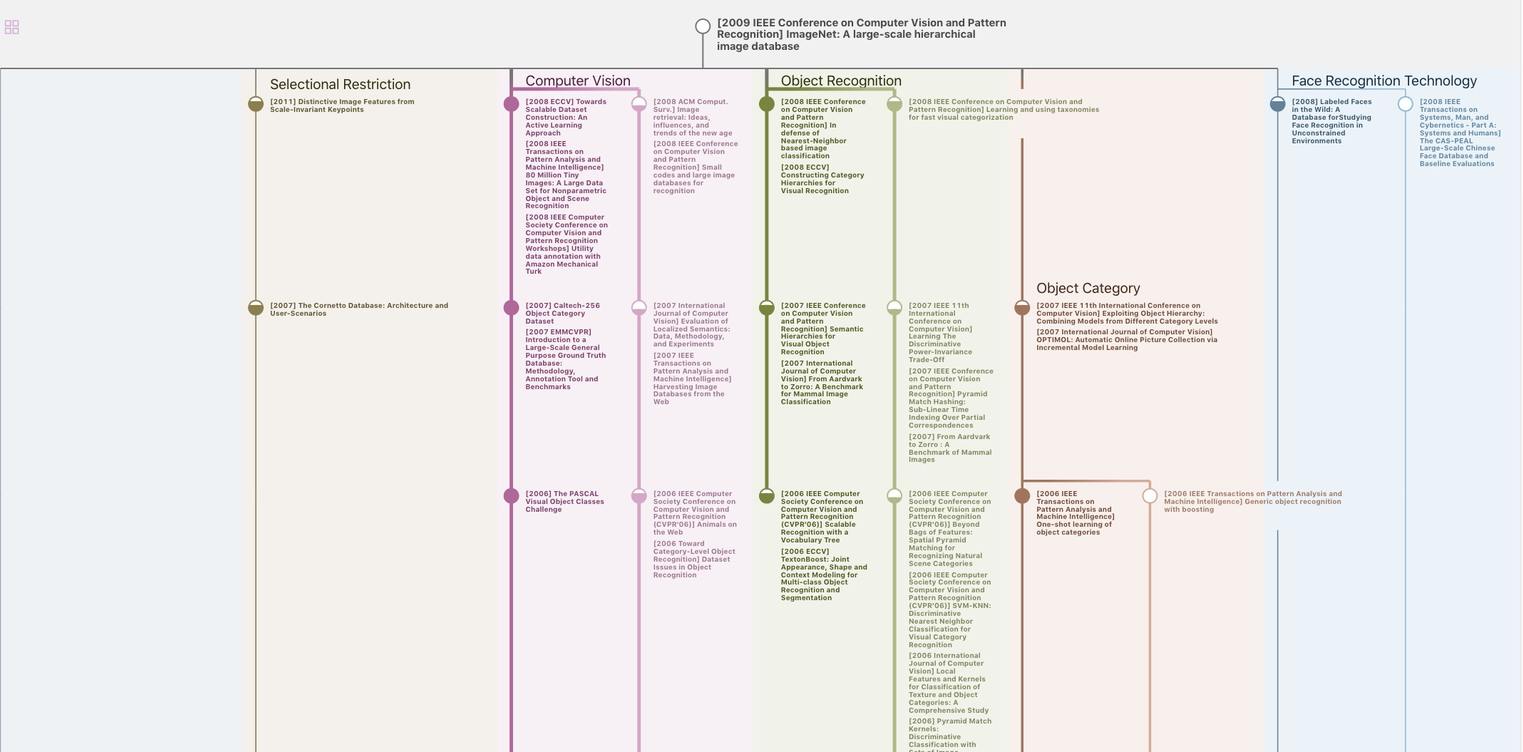
生成溯源树,研究论文发展脉络
Chat Paper
正在生成论文摘要