Predictive models to determine fine soil fractions and organic matter from readily available soil and terrain data of soils under boreal forest
GEODERMA REGIONAL(2020)
Abstract
Knowledge about the trafficability of the terrain is crucial for forest management andmechanised forest harvesting. Small-scale soil and terrain data is needed for improved trafficability estimations. This study formed predictive models for soil factors that in turn influence the soil strength and terrain trafficability in Finnish upland forests using readily available vegetation, terrain and soil data. The response soil variables represented soil texture, stoniness, organic matter content and depth of the humus layer. Explanatory variables in the models included site type, vegetation and tree coverage, climatic and topographic parameters and airborne gamma-ray radiation (K%) from the ground. The coefficients for determination in the general linear models for predicting the fine fraction content, organic matter content or depth of humus layer varied from 28 to 39%, which shows that a moderate part of the overall variation remained unexplained by the explanatory variables. The stoniness (vol% in 0-30 cm depth) could only be predicted relatively weakly (R-2 = 17%). However, the classification efficiencies of the logistic models for predicting the soil clay contents to be lower or higher than 10% were good (ROC= 0.76-0.89), which indicates significant potential to distinguish and classify the upland soils with potentialweak trafficability. Further means to improve trafficability estimations of uplands by usingmore detailed soil and regional phytogeomorphological data are discussed. (C) 2019 Elsevier B.V. All rights reserved.
MoreTranslated text
Key words
Arenosols,Cambisols,Clay,Humus layer,Leptosols,Particle size,Podzols,Site quality,Trafficability,Vegetation
AI Read Science
Must-Reading Tree
Example
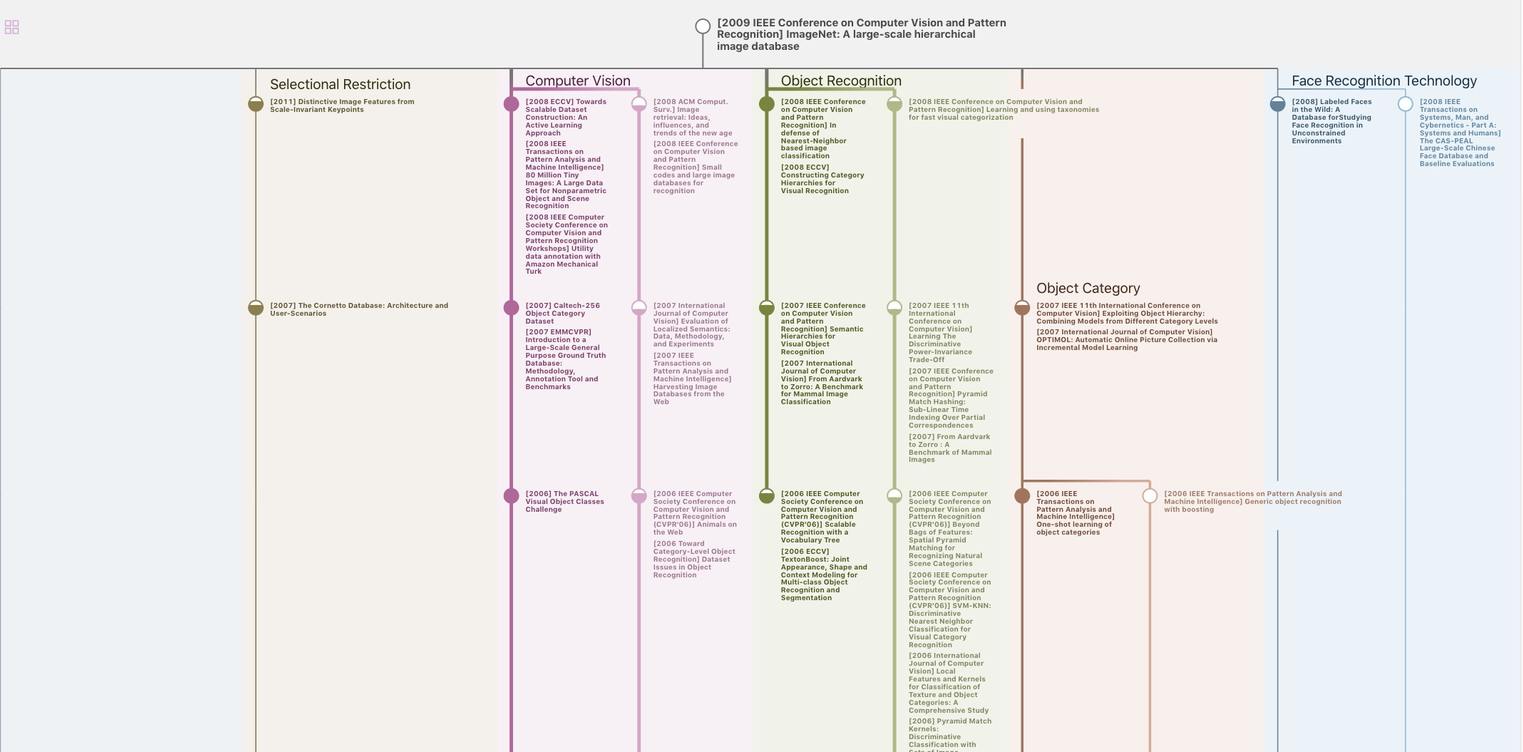
Generate MRT to find the research sequence of this paper
Chat Paper
Summary is being generated by the instructions you defined