Role of trochoidal machining process parameter and chip morphology studies during end milling of AISI D3 steel
JOURNAL OF INTELLIGENT MANUFACTURING(2019)
摘要
This study aims at discovering the effect of the trochoidal loop spacing parameter on Surface Roughness (SR), Specific Cutting Energy (SCE) and Temperature (T) during flat end milling operations. Twenty experimental runs were conducted based on the face centered central composite design (CCD) of response surface methodology (RSM). Artificial Neural Network (ANN) prediction modelling was created using four learning algorithms such as Batch Back Propagation Algorithm (BBPA), Quick Propagation Algorithm (QPA), Incremental Back Propagation Algorithm (IBPA) and Legvenberg–Marquardt back propagation Algorithm (LMBPA). The results were compared based on the value of Root mean square (RMSE) obtained for each learning algorithm and it was identified that LMBPA model produced least RMSE value. The predictive LMBPA neural network model was found to be capable of better predictions of surface roughness, temperature and specific cutting energy within the trained range. The Genetic algorithm(GA) gives the optimum parameters for conformation test and they are cutting speed of 41 m/min, feed rate of 136 mm/min and trochoidal loop spacing of 1.3 mm and error percentage between experimental and GA predicted values is 3.60% for surface roughness, 3.15% for specific cutting energy and 3.89% for temperature was found to be minimal. Scratches and serrated boundaries at both side of the chips were observed and laces, chip adhesion and side flow marks were found on machined surface.
更多查看译文
关键词
End milling, Trochoidal loop spacing, Response surface methodology, Artificial Neural Network, Specific cutting energy, Temperature, Surface roughness, Genetic algorithm
AI 理解论文
溯源树
样例
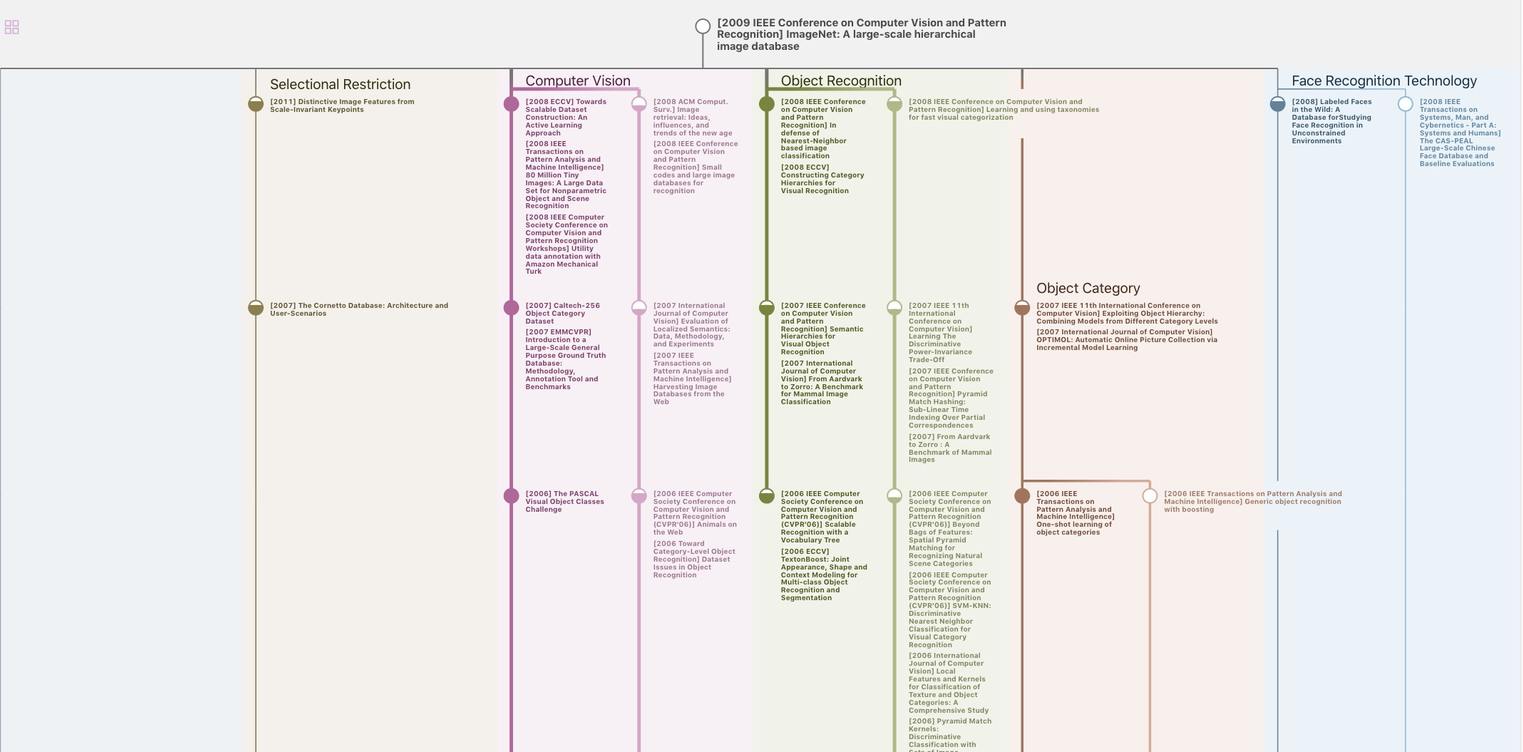
生成溯源树,研究论文发展脉络
Chat Paper
正在生成论文摘要