Networking Self-Organising Maps And Similarity Weight Associations
NEURAL INFORMATION PROCESSING, ICONIP 2019, PT V(2019)
摘要
Using a Self-Organising Map (SOM), the structure of a data set can be explored when analysing patterns between data that are multivariate, nonlinear and unlabelled in nature. As a SOM alone cannot be used to explore patterns between different data sets, a similarity weighting scheme was previously introduced to associate different SOMs in a network fashion and approximate output patterns for given inputs. This approach uses a global weight association method on the combination of all SOMs specified for a network. However, there has been a difficulty in defining the association when changing the SOM network structure. Furthermore, it has always produced the same output weight distribution for different input data that have the same best matching unit. In an attempt to overcome the issues, we propose a new approach in this paper for locally associating a pair of SOMs as a basic network building block and approximating individually associated weight distribution. The experiments using ecological data demonstrate that the proposed approach effectively associates a pair of input and output SOMs for structural flexibility of the SOM network with better approximation of output weight distributions for individual input data.
更多查看译文
关键词
Self-Organizing Map, Network, Input and output, Pattern analysis, Weight association, Similarity weight measure
AI 理解论文
溯源树
样例
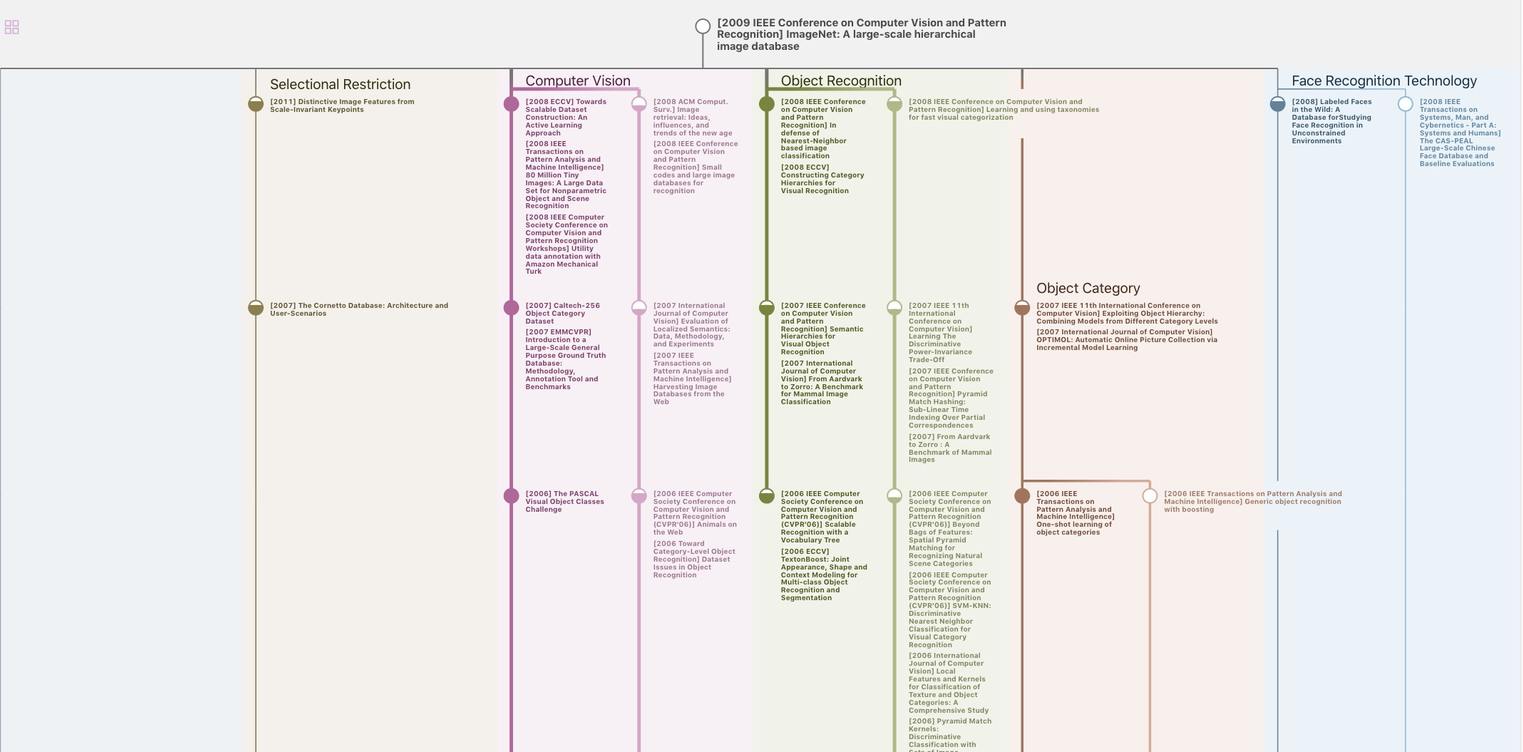
生成溯源树,研究论文发展脉络
Chat Paper
正在生成论文摘要