Flue gas monitoring system with empirically-trained dictionary
IEEE-CAA JOURNAL OF AUTOMATICA SINICA(2020)
Abstract
The monitoring of flue gas of the thermal power plants is of great significance in energy conservation and environmental protection. Spectral technique has been widely used in the gas monitoring system for predicting the concentrations of specific gas components. This paper proposes flue gas monitoring system with empirically-trained dictionary (ETD) to deal with the complexity and biases brought by the uninformative spectral data. Firstly, ETD is extracted from the raw spectral data by an alternative optimization between the sparse coding stage and the dictionary update stage to minimize the error of sparse representation. D1, D2 and D3 are three types of ETD obtained by different methods. Then, the predictive model of component concentration is constructed on the ETD. In the experiments, two real flue gas spectral datasets are collected and the proposed method combined with the partial least squares, the background propagation neural network and the support vector machines are performed. Moreover, the optimal parameters are chosen according to the 10-fold root-mean-square error of cross validation. The experimental results demonstrate that the proposed method can be used for quantitative analysis effectively and ETD can be applied to the gas monitoring systems.
MoreTranslated text
Key words
Dictionary learning,empirically-trained dictionaty (ETD),flue gas monitoring system,quantitative analysis
AI Read Science
Must-Reading Tree
Example
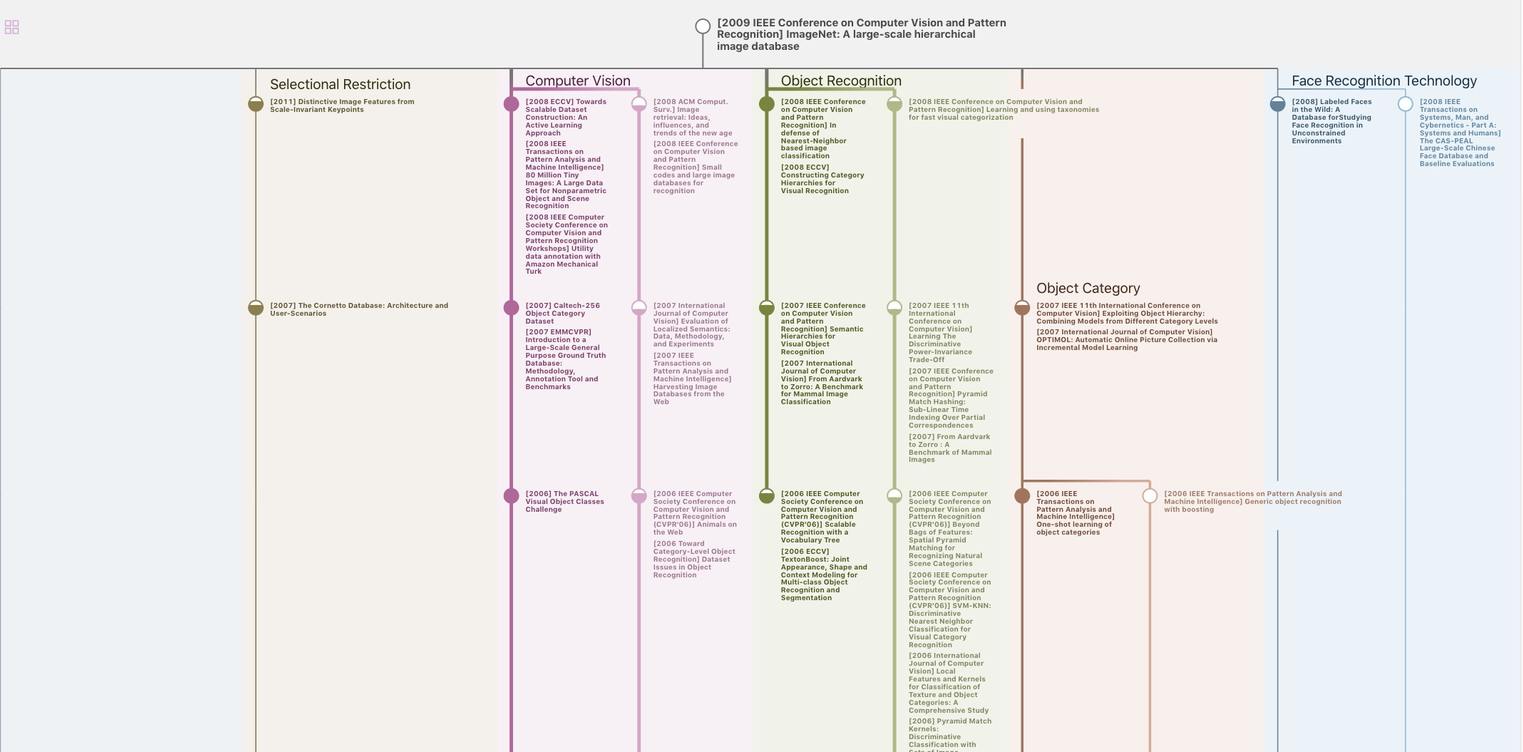
Generate MRT to find the research sequence of this paper
Chat Paper
Summary is being generated by the instructions you defined