An Iterative Learning Control Algorithm With Gain Adaptation for Stochastic Systems
IEEE Transactions on Automatic Control(2020)
摘要
This paper proposes an iterative learning control (ILC) algorithm with gain adaptation for discrete-time stochastic systems. The algorithm is based on Kesten's accelerated stochastic approximation (SA) algorithm. The gain adaptation uses only tracking error information, and, hence, is a data-driven adaptation approach. If stochastic noises account for a small proportion of the tracking error, the learning gain matrix remains constant with a high probability. If stochastic noises dominate the tracking error, the learning gain matrix is decreasing. Therefore, the new ILC algorithm converges more quickly than existing SA-based algorithms. In addition, the classic P-type ILC law for noise-free systems is a special case of the new ILC algorithm. The behaviors of the proposed ILC algorithm are demonstrated through examples.
更多查看译文
关键词
Convergence,Approximation algorithms,Stochastic systems,Acceleration,Covariance matrices,Iterative learning control,Indexes
AI 理解论文
溯源树
样例
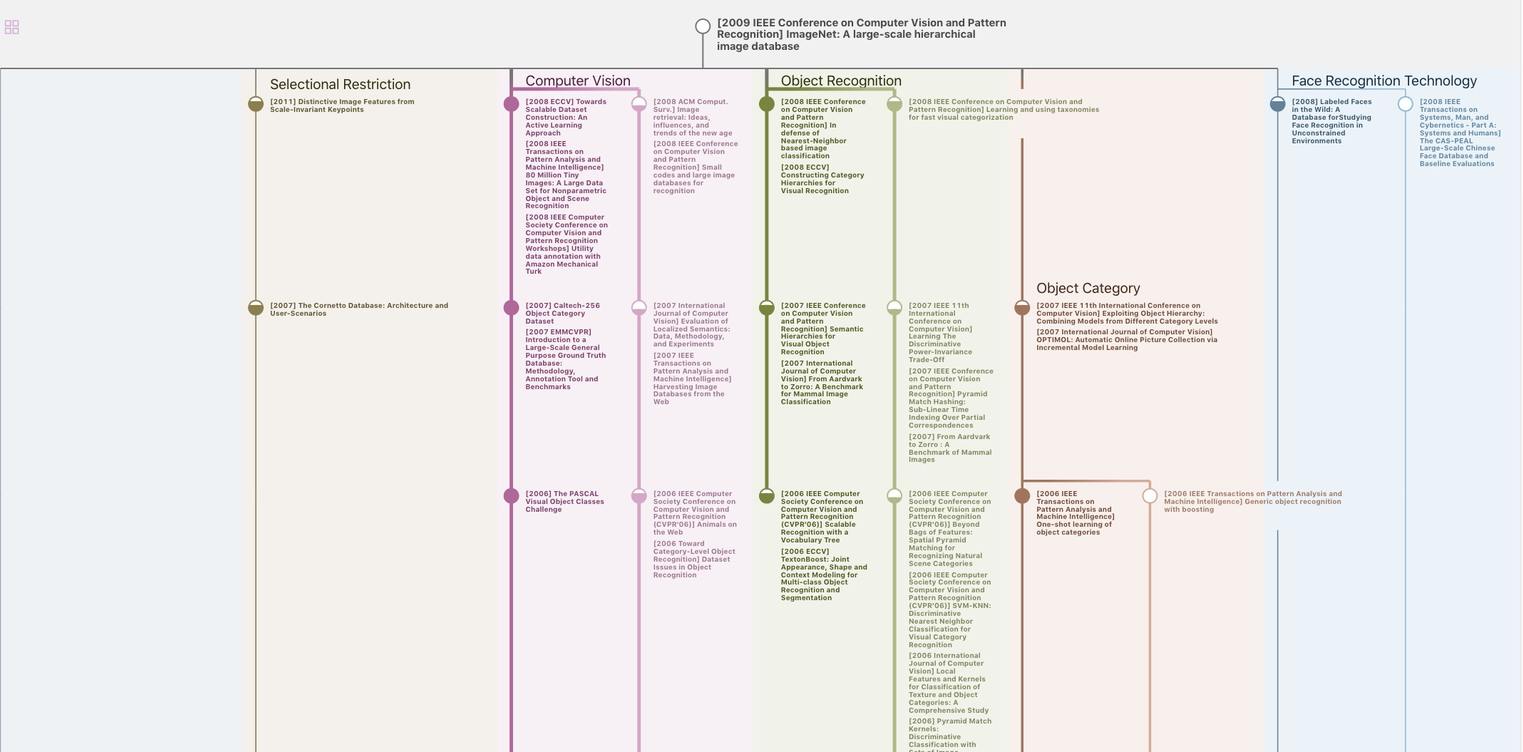
生成溯源树,研究论文发展脉络
Chat Paper
正在生成论文摘要