An Overhead-Free Max-Pooling Method for SNN
IEEE Embedded Systems Letters(2020)
摘要
Spiking neural networks (SNNs) have been shown to be accurate, fast, and efficient in classical machine vision tasks, such as object recognition or detection. It is typical to convert a pretrained deep neural network into an SNN since training SNN is not easy. The max-pooling (MP) function is widely adopted in most state-of-the-art deep neural networks. To maintain the accuracy of the SNN obtained through conversion, this function is an important element to be implemented. However, it is difficult due to the dynamic characteristics of spikes. As far as we know, existing solutions adopt additional technologies except the spiking neuron model to implement MP or approximate MP, which introduce overhead of memory storage and computation. In this letter, we propose a novel method that utilizes only the spiking neuron model to approximate MP. Our method does not incur any overhead. We validate our method with three datasets and six networks including three oxford visual geometry group-like networks. And the experimental results show that the performance (accuracy and convergence rate) of our method is as good as or even better than that of the existing method.
更多查看译文
关键词
Neurons,Microsoft Windows,Mathematical model,Training,Computational modeling,Biological neural networks,Task analysis
AI 理解论文
溯源树
样例
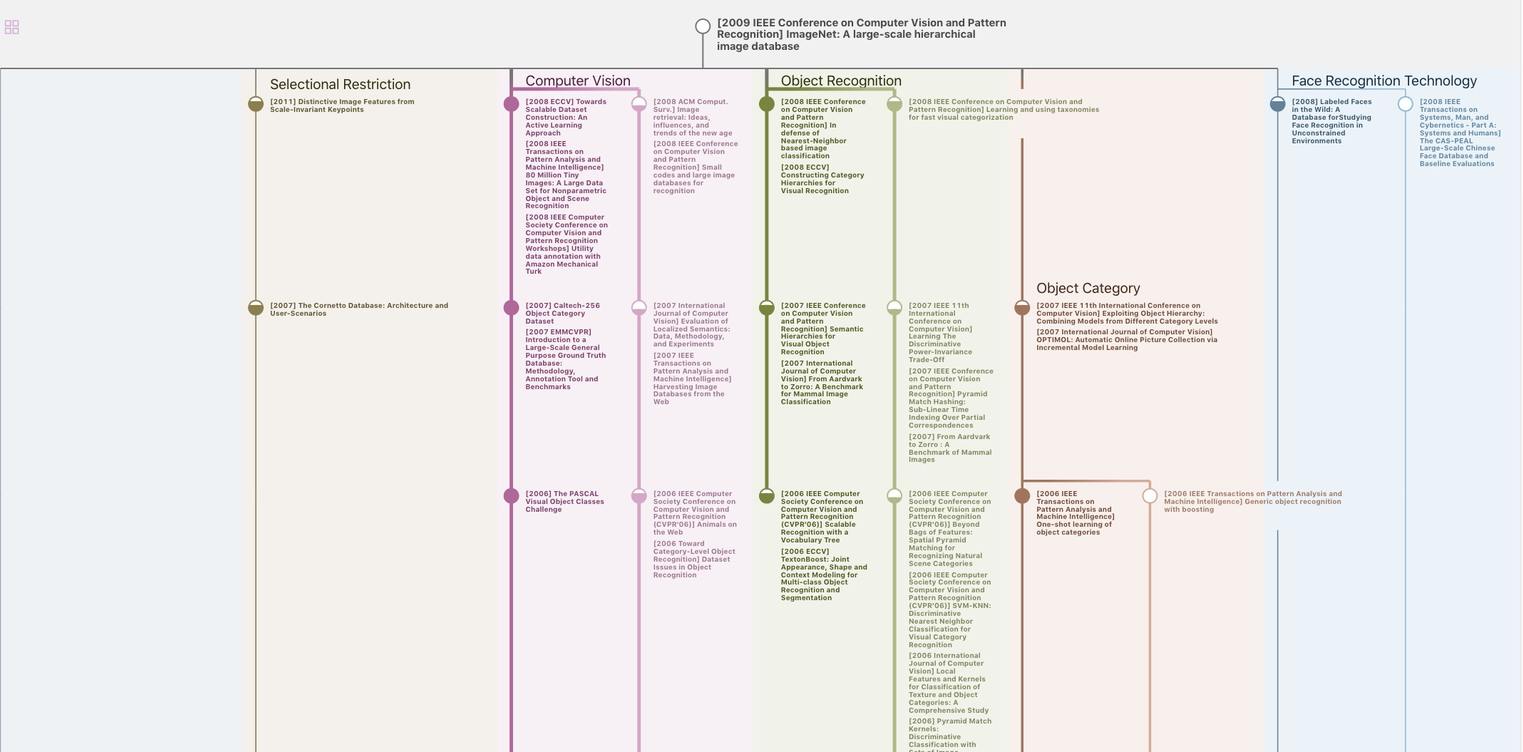
生成溯源树,研究论文发展脉络
Chat Paper
正在生成论文摘要