Learning Deep Spatiotemporal Feature for Engagement Recognition of Online Courses
2019 IEEE Symposium Series on Computational Intelligence (SSCI)(2019)
Abstract
This paper focuses on the study of engagement recognition of online courses from students' appearance and behavioral information using deep learning methods. Automatic engagement recognition can be applied to developing effective online instructional and assessment strategies for promoting learning. In this paper, we make two contributions. First, we propose a Convolutional 3D (C3D) neural networks-based approach to automatic engagement recognition, which models both the appearance and motion information in videos and recognize student engagement automatically. Second, we introduce the Focal Loss to address the class-imbalanced data distribution problem in engagement recognition by adaptively decreasing the weight of high engagement samples while increasing the weight of low engagement samples in deep spatiotemporal feature learning. Experiments on the DAiSEE dataset show the effectiveness of our method in comparison with the state-of-the-art automatic engagement recognition methods.
MoreTranslated text
Key words
engagement recognition,spatiotemporal features,Convolutional 3D,class-imbalanced,Focal Loss
AI Read Science
Must-Reading Tree
Example
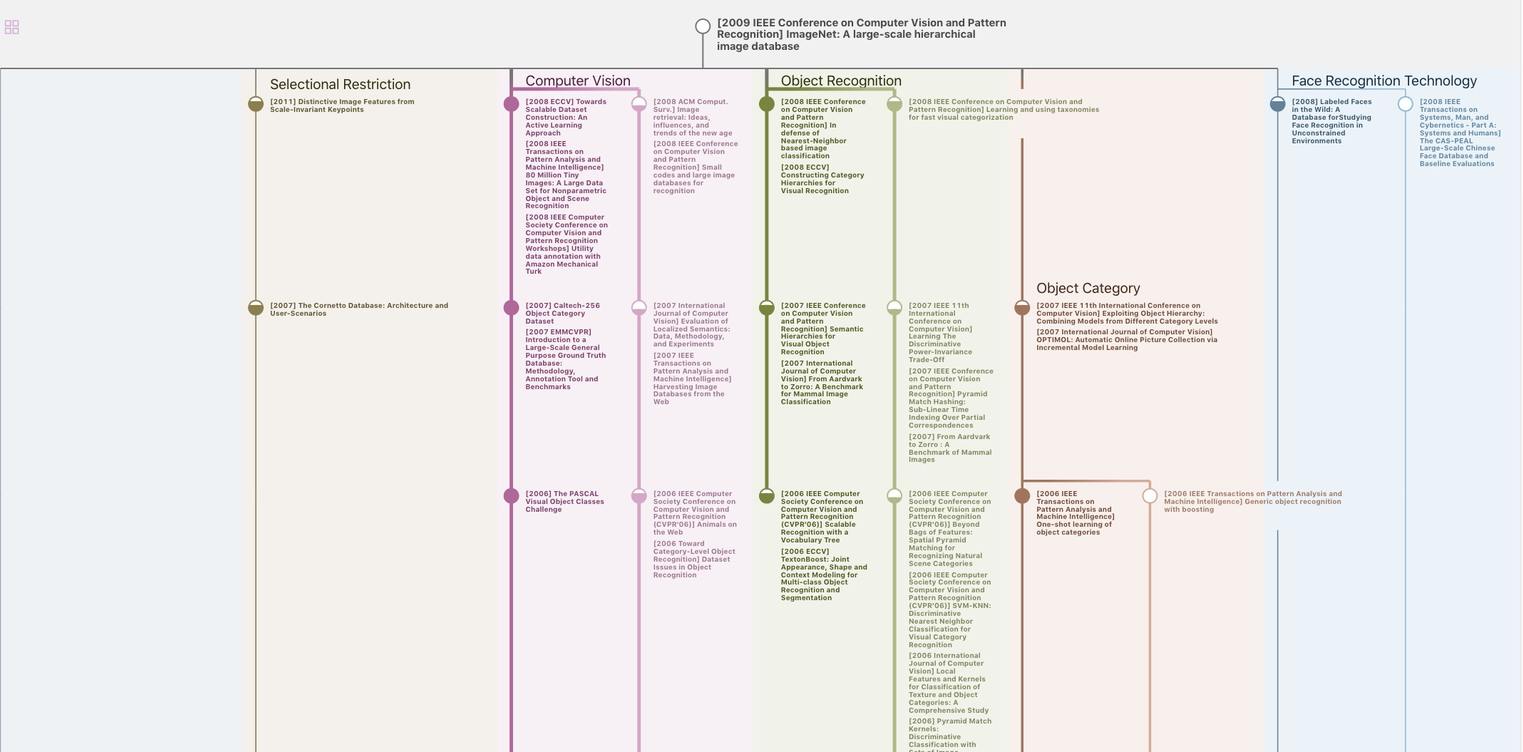
Generate MRT to find the research sequence of this paper
Chat Paper
Summary is being generated by the instructions you defined