Bilingual Auto-Categorization Comparison of Two LSTM Text Classifiers.
IIAI-AAI(2019)
摘要
Multi linguistic problems such as auto-categorization is not an easy task. It is possible to train different models for each language, another way to do auto-categorization is to build the model in one base language and use automatic translation from other languages to that base language. Different languages have a bias to a language specific grammar and syntax and will therefore pose problems to be expressed in other languages. Translating from one language into a non-verbal language could potentially have a positive impact of the categorization results. A non-verbal language could for example be pure information in form of a knowledge graph relation extraction from the text. In this article a comparison is conducted between Chinese and Swedish languages. Two categorization models are developed and validated on each dataset. The purpose is to make an auto-categorization model that works for nu0027importe quel langage. One model is built upon LSTM and optimized for Swedish and the other is an improved Bidirectional-LSTM Convolution model optimized for Chinese. The improved algorithm is trained on both languages and compared with the LSTM algorithm. The Bidirectional-LSTM algorithm performs approximately 20% units better than the LSTM algorithm, which is significant.
更多查看译文
关键词
neural network,chinese dataset,memory cell,convolutional neural network,feature map,chinese language,swedish dataset,auto categorization,information system,natural language processing,news article
AI 理解论文
溯源树
样例
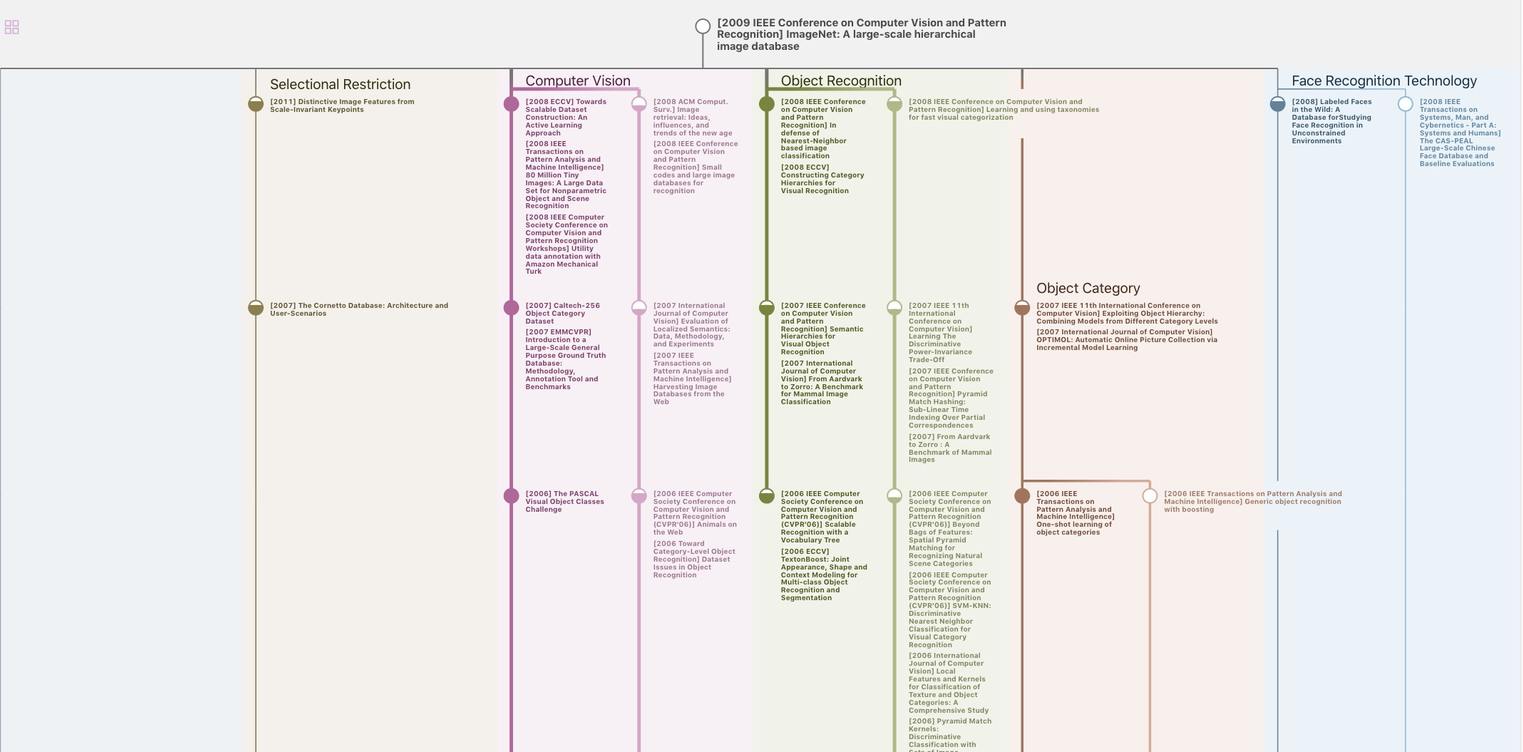
生成溯源树,研究论文发展脉络
Chat Paper
正在生成论文摘要