Small-Footprint Open-Vocabulary Keyword Spotting with Quantized LSTM Networks
CoRR(2020)
Abstract
We explore a keyword-based spoken language understanding system, in which the intent of the user can directly be derived from the detection of a sequence of keywords in the query. In this paper, we focus on an open-vocabulary keyword spotting method, allowing the user to define their own keywords without having to retrain the whole model. We describe the different design choices leading to a fast and small-footprint system, able to run on tiny devices, for any arbitrary set of user-defined keywords, without training data specific to those keywords. The model, based on a quantized long short-term memory (LSTM) neural network, trained with connectionist temporal classification (CTC), weighs less than 500KB. Our approach takes advantage of some properties of the predictions of CTC-trained networks to calibrate the confidence scores and implement a fast detection algorithm. The proposed system outperforms a standard keyword-filler model approach.
MoreTranslated text
Key words
quantized lstm networks,small-footprint,open-vocabulary
AI Read Science
Must-Reading Tree
Example
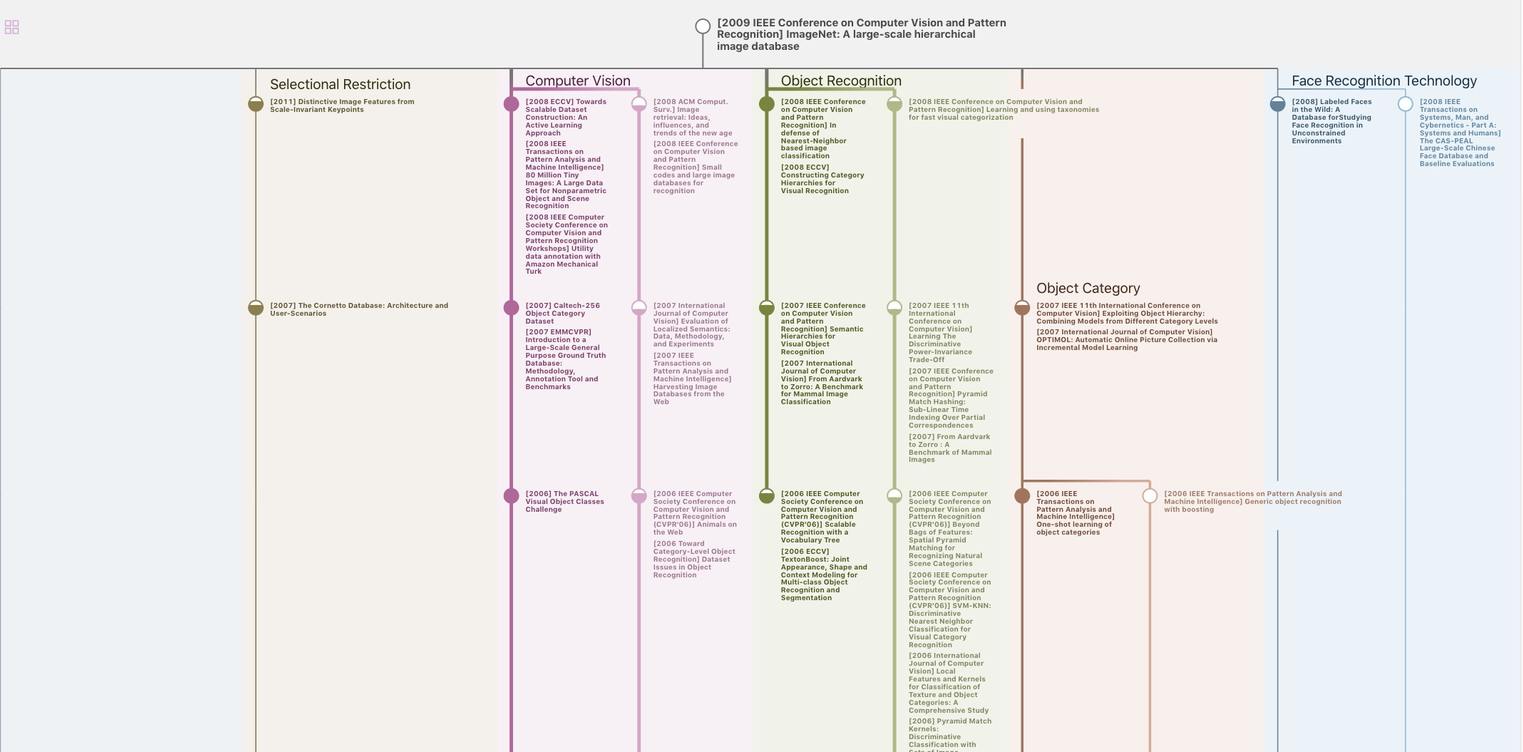
Generate MRT to find the research sequence of this paper
Chat Paper
Summary is being generated by the instructions you defined