3d Skeletal Gesture Recognition Via Hidden States Exploration
IEEE TRANSACTIONS ON IMAGE PROCESSING(2020)
摘要
Temporal dynamics is an open issue for modeling human body gestures. A solution is resorting to the generative models, such as the hidden Markov model (HMM). Nevertheless, most of the work assumes fixed anchors for each hidden state, which make it hard to describe the explicit temporal structure of gestures. Based on the observation that a gesture is a time series with distinctly defined phases, we propose a new formulation to build temporal compositions of gestures by the low-rank matrix decomposition. The only assumption is that the gesture's "hold" phases with static poses are linearly correlated among each other. As such, a gesture sequence could be segmented into temporal states with semantically meaningful and discriminative concepts. Furthermore, different to traditional HMMs which tend to use specific distance metric for clustering and ignore the temporal contextual information when estimating the emission probability, we utilize the long short-term memory to learn probability distributions over states of HMM. The proposed method is validated on multiple challenging datasets. Experiments demonstrate that our approach can effectively work on a wide range of gestures, and achieve state-of-the-art performance.
更多查看译文
关键词
Hidden Markov models, Three-dimensional displays, Gesture recognition, Matrix decomposition, Electronic mail, Manifolds, Robustness, Gesture recognition, hidden Markov model, deep neural networks, matrix decomposition
AI 理解论文
溯源树
样例
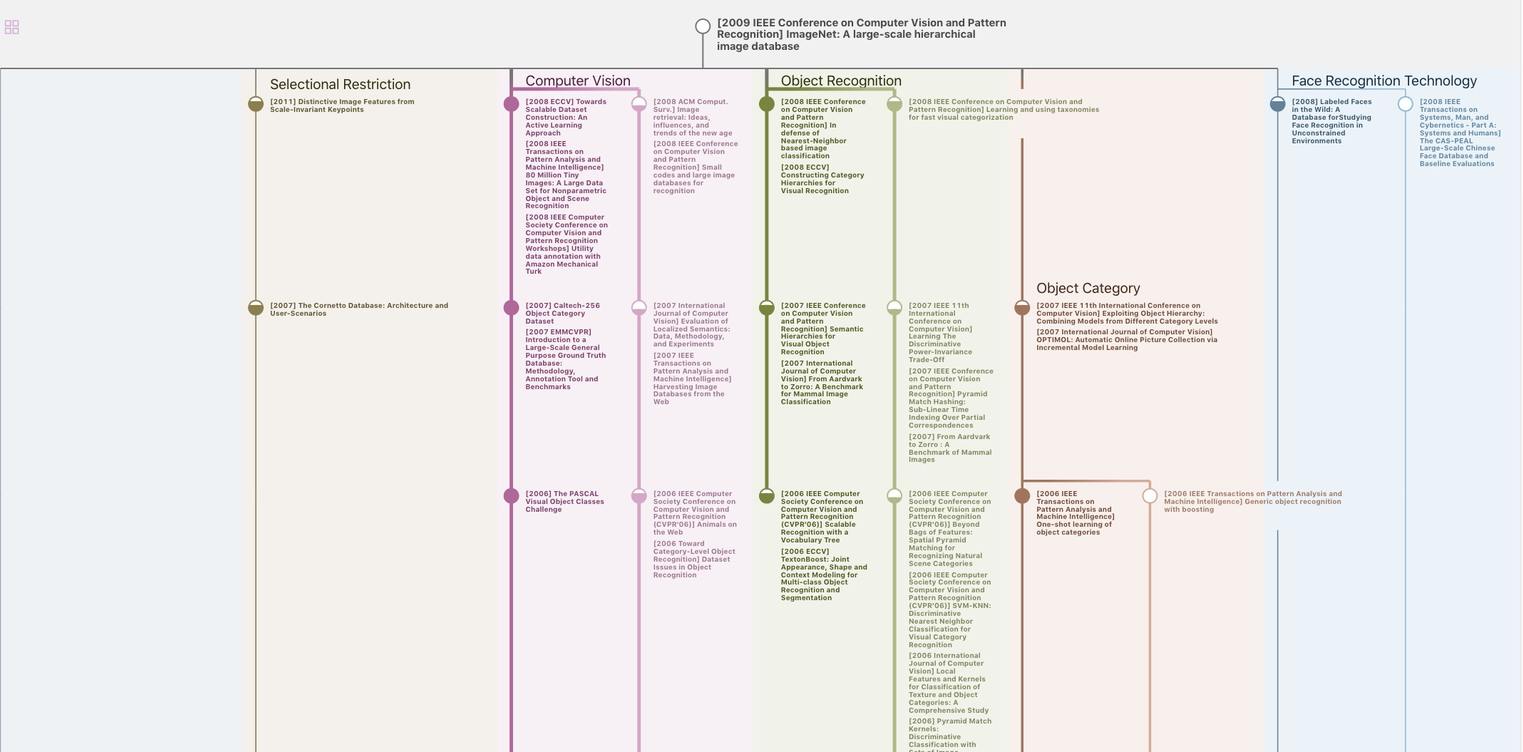
生成溯源树,研究论文发展脉络
Chat Paper
正在生成论文摘要