Syllable-Dependent Discriminative Learning for Small Footprint Text-Dependent Speaker Verification
2019 IEEE Automatic Speech Recognition and Understanding Workshop (ASRU)(2019)
摘要
This study proposes a novel scheme of syllable-dependent discriminative speaker embedding learning for small footprint text-dependent speaker verification systems. To suppress undesired syllable variation and enhance the power of discrimination inherited in the frame-level features, we design a novel syllable-dependent clustering loss to optimize the network. Specifically, this loss function utilizes syllable labels as auxiliary supervision information to explicitly maximize inter-syllable divisibility and intra-syllable compactness between the learned frame-level features. Successively, we propose two syllable-dependent pooling mechanisms to aggregate the frame-level features to several syllable-level features by averaging those features corresponding to each syllable. The utterance-level speaker embeddings with powerful discrimination are then obtained by concatenating the syllable-level features. Experimental results on Tencent voice wake-up dataset show that our proposed scheme can accelerate the network convergence and achieve significant performance improvement against the state-of-the-art methods.
更多查看译文
关键词
Text-dependent speaker verification,pooling mechanism,syllable-dependent,discriminative speaker embedding
AI 理解论文
溯源树
样例
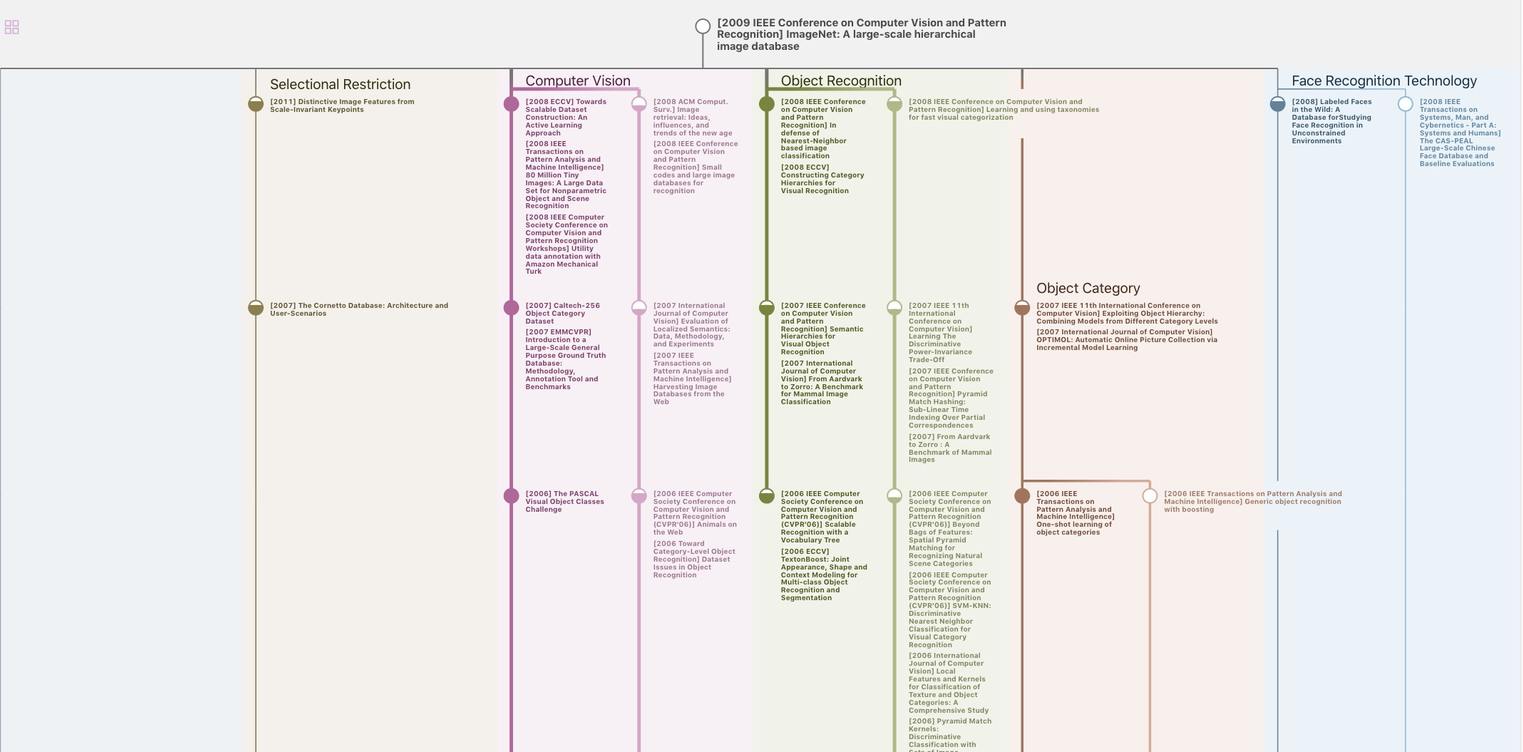
生成溯源树,研究论文发展脉络
Chat Paper
正在生成论文摘要