An Effective CNN Approach for Diabetic Retinopathy Stage Classification with Dual Inputs and Selective Data Sampling
ICMLA(2019)
摘要
Diabetic retinopathy (DR) is a vision-threatening complication among the diabetic population and a leading cause of blindness for working-age adults. Early detection and timely treatment can reduce the occurrence of blindness due to DR. Computer-aided diagnosis have great potential to significantly improve the accuracy and speed in DR detection over the traditional manual diagnosis process. In this paper, we present a deep convolutional neural network for DR stage classification, trained and evaluated on a large dataset. Our model uses high-resolution retinal fundus images of both the left and right eyes as inputs to take advantage of more detailed retinal lesion information in images and strong correlation between both eyes. Selective data sampling (SeS) is applied in the training process to mitigate the data imbalance problem. Experiments show that our model outperforms the fine-tuned Inception-v3 model by every measure, achieving an accuracy of 87.2% and a Kappa score of 0.806 on the Kaggle dataset.
更多查看译文
关键词
convolutional neural networks,image classification,deep learning
AI 理解论文
溯源树
样例
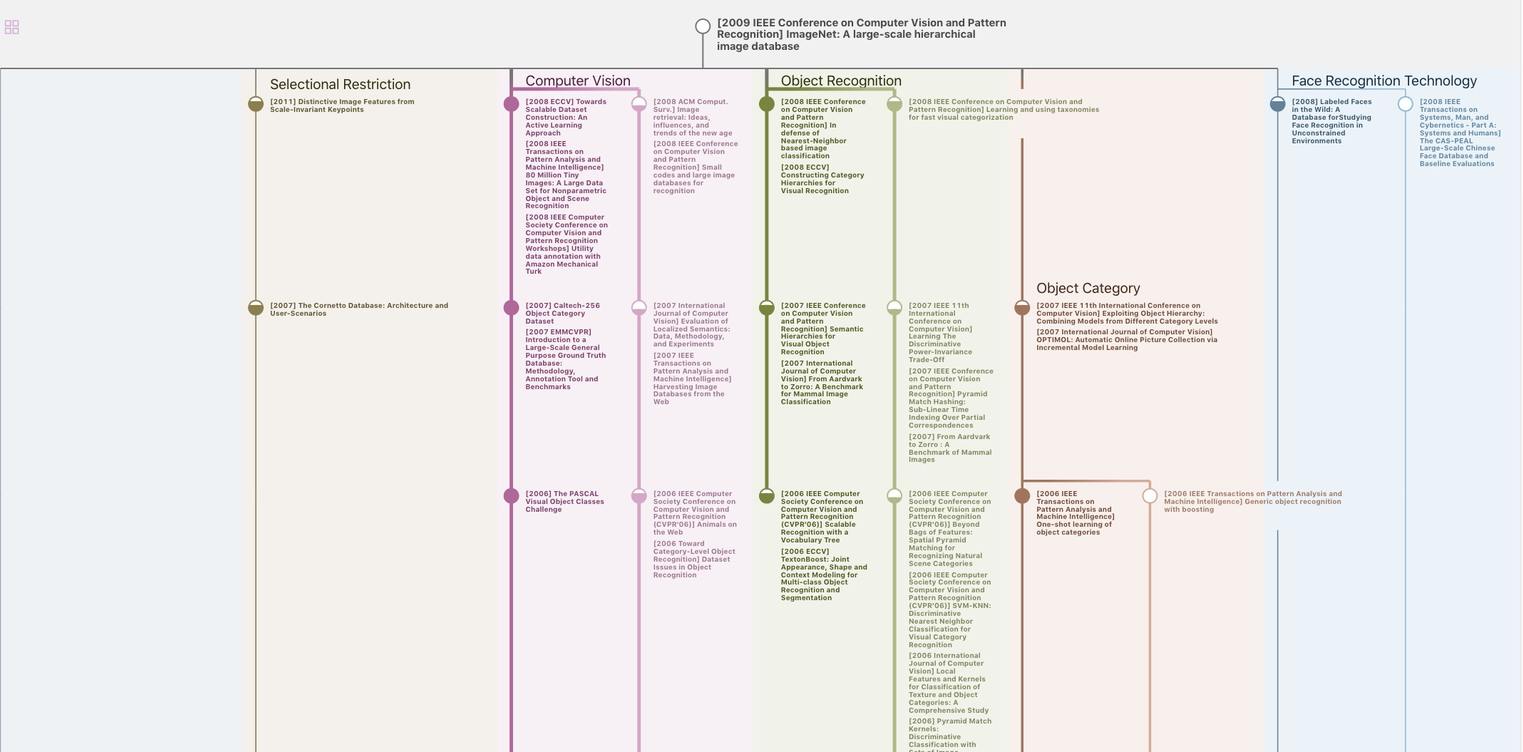
生成溯源树,研究论文发展脉络
Chat Paper
正在生成论文摘要