A Kernel -Means-Based Method and Attribute Selections for Diabetes Diagnosis.
JACIII(2020)
摘要
Diabetes diagnosis is important due to the high death rate and complication consequences caused by the disease. First, we propose a kernel k-means-based prediction method and explore attribute selections for effective and robust diabetes diagnosis. This method derives homogeneous sub-clusters in the high dimensional kernelized feature space to compute the distance of a new instance to those sub-clusters, and then apply the 1-nearest neighbor to classify it as positive or negative to the disease. Our experimental results could identify the best effective attribute group for each considered prediction method and show that the proposed method outperforms the existing ones for the task. Second, we introduce our developed diabetes visualization and decision support system, named DIAVIS, which is equipped with the proposed prediction method. This system can support doctors to diagnose diabetes and track patient health progress to prescribe proper medications in a treatment process.
更多查看译文
AI 理解论文
溯源树
样例
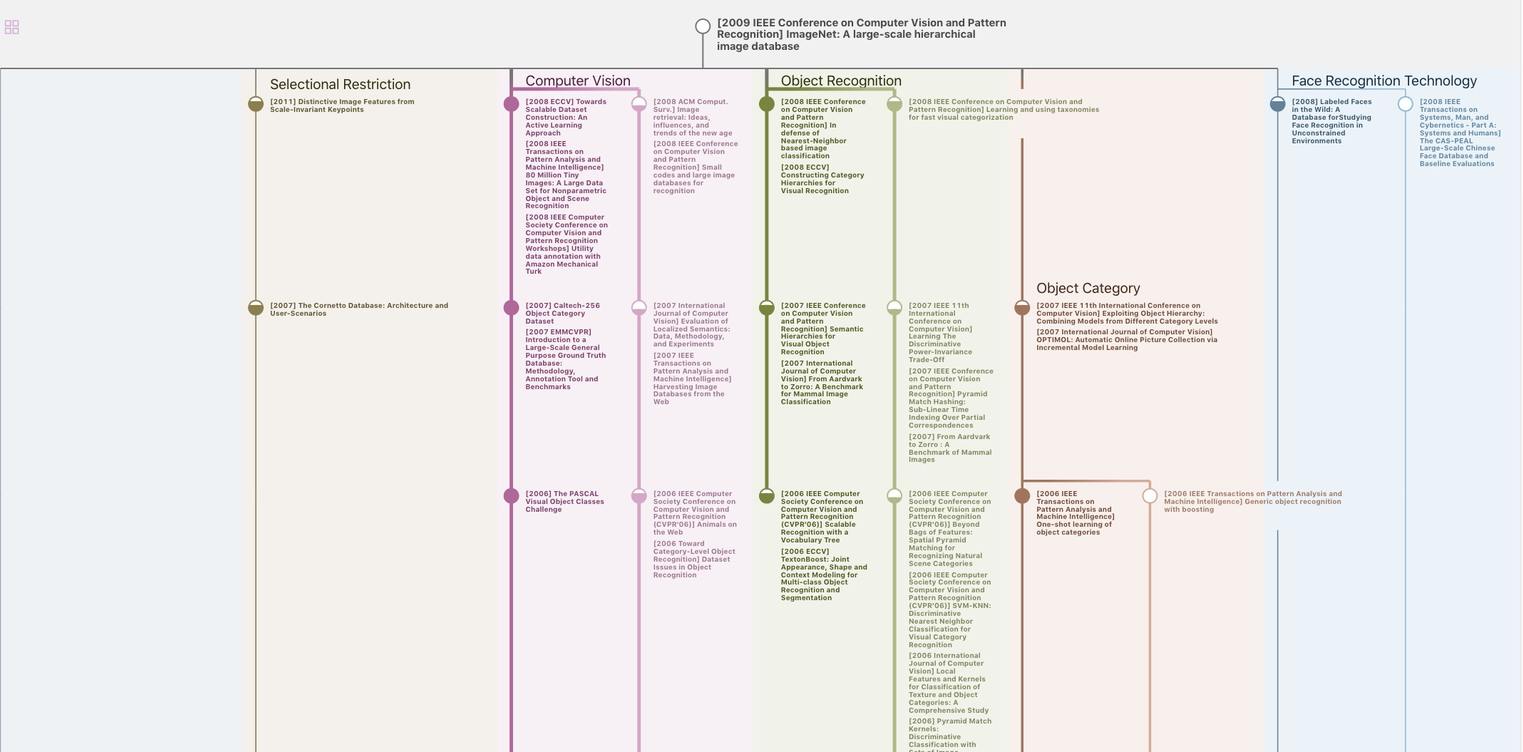
生成溯源树,研究论文发展脉络
Chat Paper
正在生成论文摘要