Learning To Select Bi-Aspect Information For Document-Scale Text Content Manipulation
THIRTY-FOURTH AAAI CONFERENCE ON ARTIFICIAL INTELLIGENCE, THE THIRTY-SECOND INNOVATIVE APPLICATIONS OF ARTIFICIAL INTELLIGENCE CONFERENCE AND THE TENTH AAAI SYMPOSIUM ON EDUCATIONAL ADVANCES IN ARTIFICIAL INTELLIGENCE(2020)
摘要
In this paper, we focus on a new practical task, document-scale text content manipulation, which is the opposite of text style transfer and aims to preserve text styles while altering the content. In detail, the input is a set of structured records and a reference text for describing another recordset. The output is a summary that accurately describes the partial content in the source recordset with the same writing style of the reference. The task is unsupervised due to lack of parallel data, and is challenging to select suitable records and style words from bi-aspect inputs respectively and generate a high-fidelity long document. To tackle those problems, we first build a dataset based on a basketball game report corpus as our testbed, and present an unsupervised neural model with interactive attention mechanism, which is used for learning the semantic relationship between records and reference texts to achieve better content transfer and better style preservation. In addition, we also explore the effectiveness of the back-translation in our task for constructing some pseudotraining pairs. Empirical results show superiority of our approaches over competitive methods, and the models also yield a new state-of-the-art result on a sentence-level dataset.(1)
更多查看译文
关键词
text,content
AI 理解论文
溯源树
样例
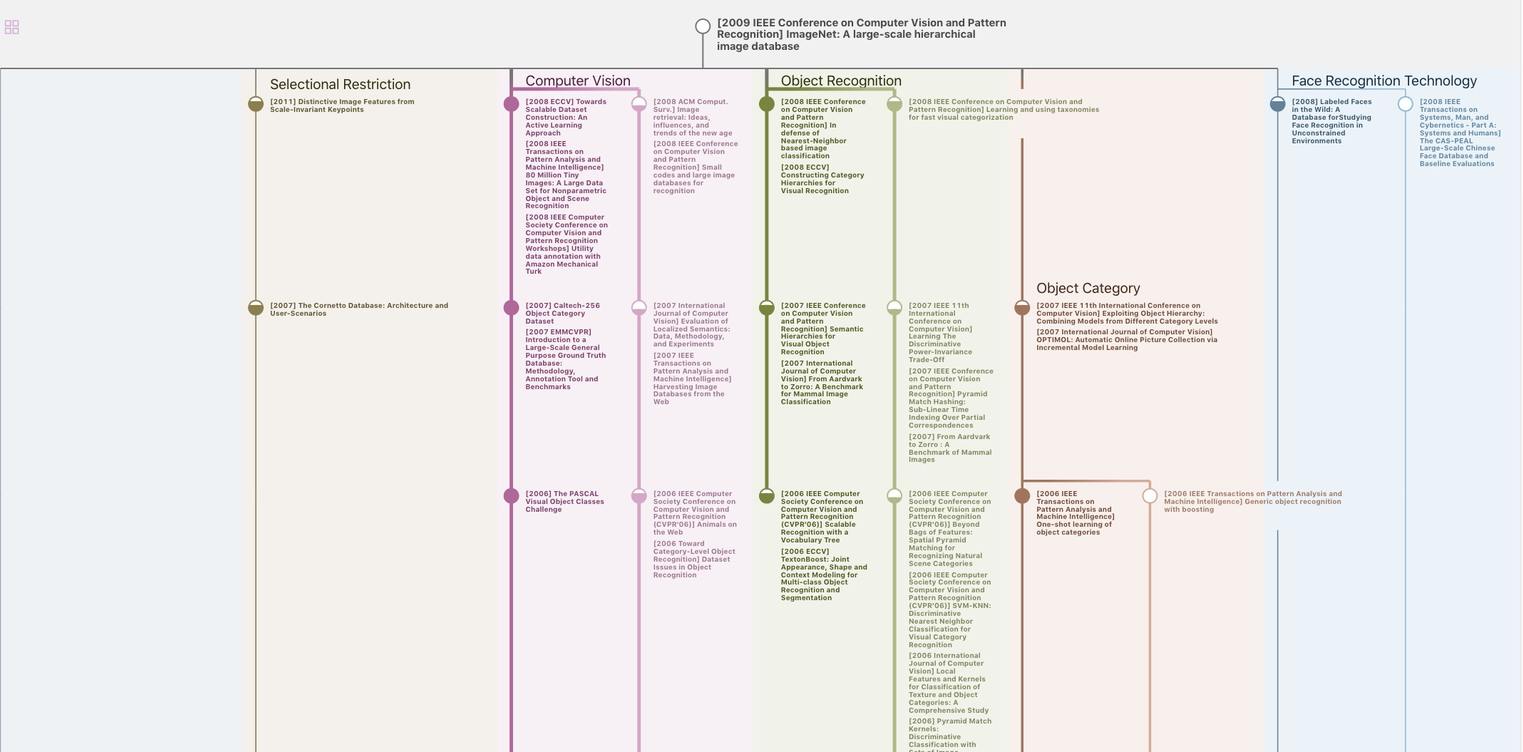
生成溯源树,研究论文发展脉络
Chat Paper
正在生成论文摘要