A Critical View of the Structural Causal Model
CoRR(2020)
摘要
In the univariate case, we show that by comparing the individual complexities of univariate cause and effect, one can identify the cause and the effect, without considering their interaction at all. In our framework, complexities are captured by the reconstruction error of an autoencoder that operates on the quantiles of the distribution. Comparing the reconstruction errors of the two autoencoders, one for each variable, is shown to perform surprisingly well on the accepted causality directionality benchmarks. Hence, the decision as to which of the two is the cause and which is the effect may not be based on causality but on complexity. In the multivariate case, where one can ensure that the complexities of the cause and effect are balanced, we propose a new adversarial training method that mimics the disentangled structure of the causal model. We prove that in the multidimensional case, such modeling is likely to fit the data only in the direction of causality. Furthermore, a uniqueness result shows that the learned model is able to identify the underlying causal and residual (noise) components. Our multidimensional method outperforms the literature methods on both synthetic and real world datasets.
更多查看译文
关键词
critical view,model
AI 理解论文
溯源树
样例
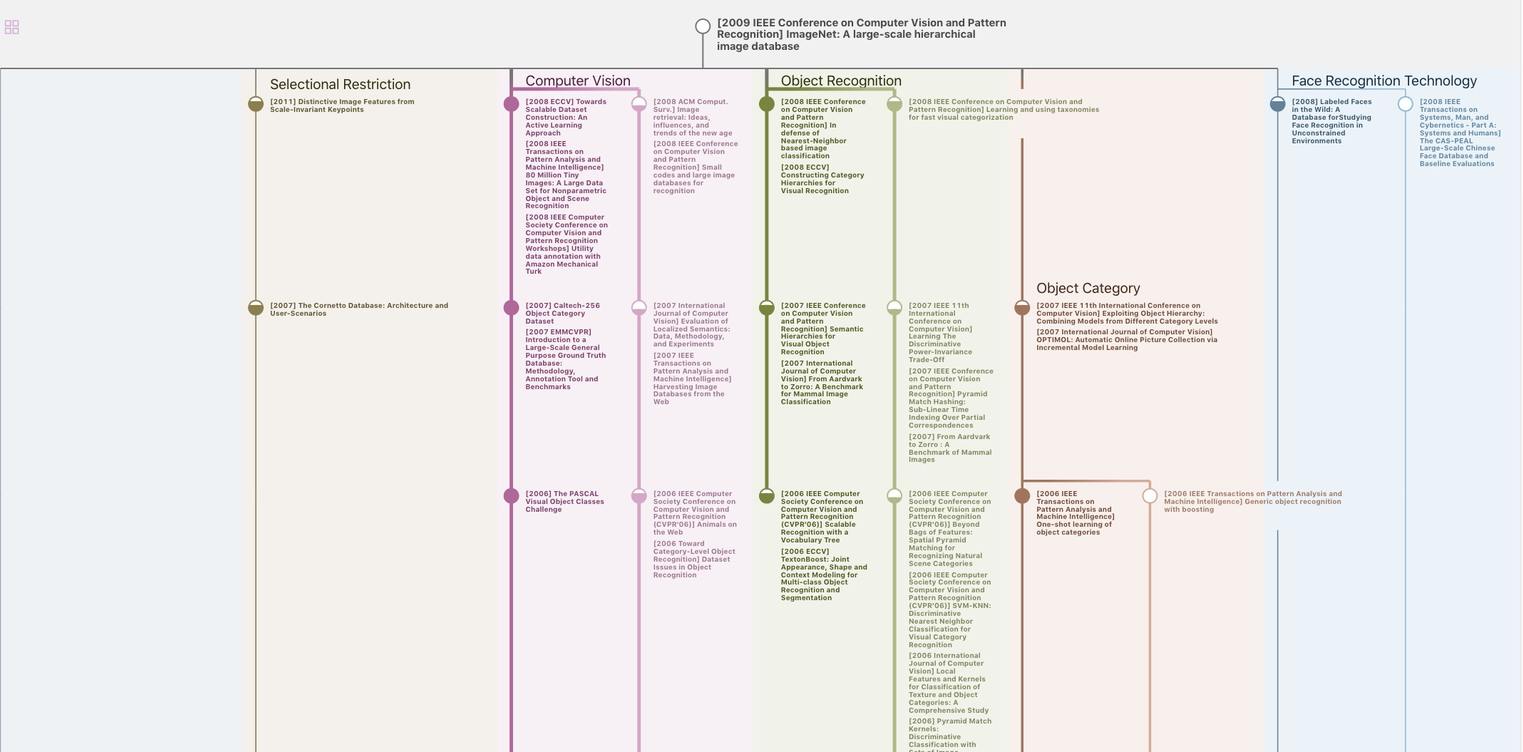
生成溯源树,研究论文发展脉络
Chat Paper
正在生成论文摘要