Using machine learning to separate hadronic and electromagnetic interactions in the GlueX forward calorimeter
JOURNAL OF INSTRUMENTATION(2020)
摘要
The GlueX forward calorimeter is an array of 2800 lead glass modules that was constructed to detect photons produced in the decays of hadrons. A background to this process originates from hadronic interactions in the calorimeter, which, in some instances, can be difficult to distinguish from low energy photon interactions. Machine learning techniques were applied to the classification of particle interactions in the GlueX forward calorimeter. The algorithms were trained on data using decays of the omega meson, which contain both true photons and charged particles that interact with the calorimeter. Algorithms were evaluated on efficiency, rate of false positives, run time, and implementation complexity. An algorithm that utilizes a multi-layer perceptron neural net was deployed in the GlueX software stack and provides a signal efficiency of 85% with a background rejection of 60% for an inclusive pi(0) data sample for an intermediate quality constraint.
更多查看译文
关键词
Analysis and statistical methods,Pattern recognition,cluster finding,calibration and fitting methods
AI 理解论文
溯源树
样例
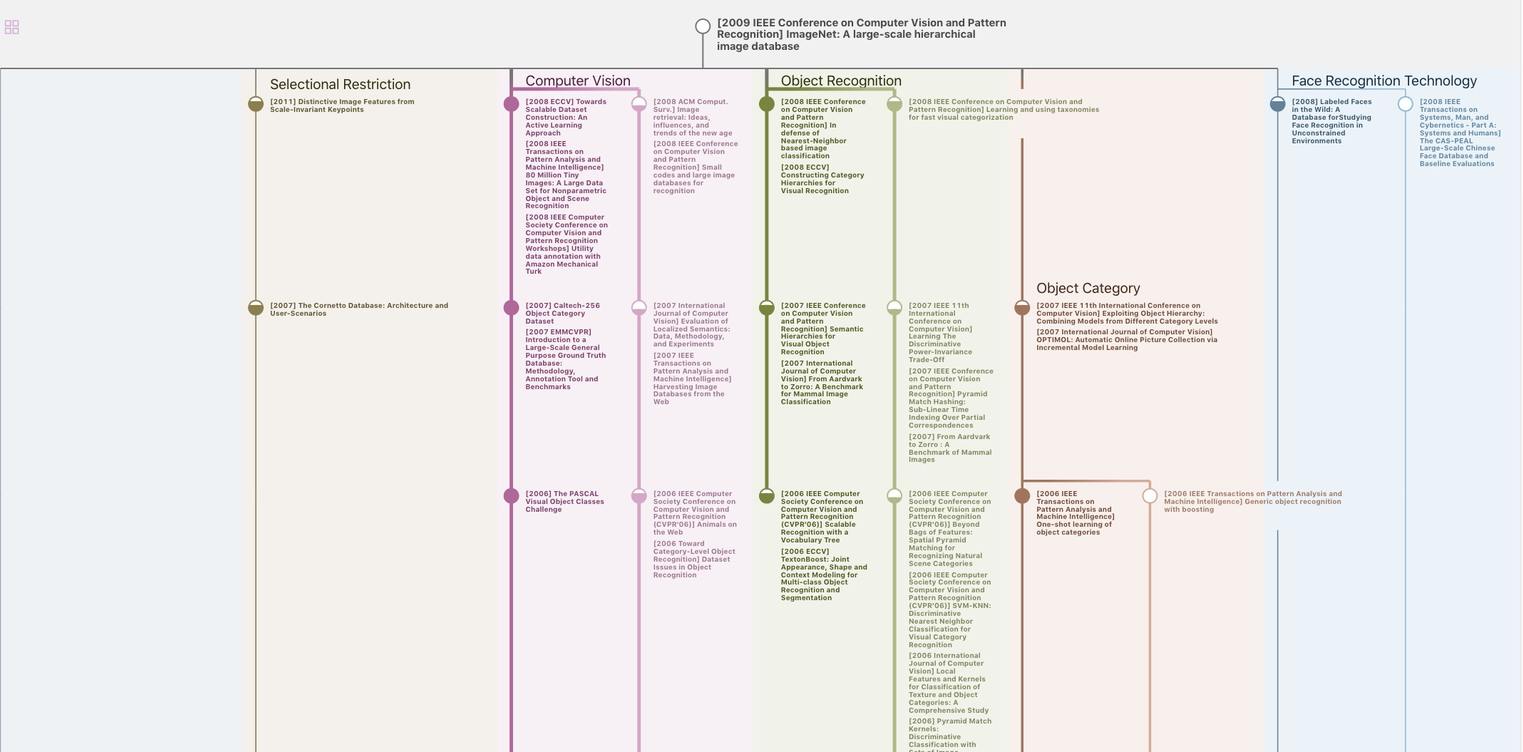
生成溯源树,研究论文发展脉络
Chat Paper
正在生成论文摘要