Verifying Conformance of Neural Network Models: Invited Paper
2019 IEEE/ACM International Conference on Computer-Aided Design (ICCAD)(2019)
摘要
Neural networks are increasingly used as data-driven models for a wide variety of physical systems such as ground vehicles, airplanes, human physiology and automobile engines. These models are in-turn used for designing and verifying autonomous systems. The advantages of using neural networks include the ability to capture characteristics of particular systems using the available data. This is particularly advantageous for medical systems, wherein the data collected from individuals can be used to design devices that are well-adapted to a particular individual's unique physiological characteristics. At the same time, neural network models remain opaque: their structure makes them hard to understand and interpret by human developers. One key challenge lies in checking that neural network models of processes are “conformant” to the well established scientific (physical, chemical and biological) laws that underlie these models. In this paper, we will show how conformance often fails in models that are otherwise accurate and trained using the best practices in machine learning, with potentially serious consequences. We motivate the need for learning and verifying key conformance properties in data-driven models of the human insulin-glucose system and data-driven automobile models. We survey verification approaches for neural networks that can hold the key to learning and verifying conformance.
更多查看译文
关键词
machine learning,airplanes,ground vehicles,data-driven automobile models,human insulin-glucose system,medical systems,autonomous systems,automobile engines,human physiology,physical systems,neural network models
AI 理解论文
溯源树
样例
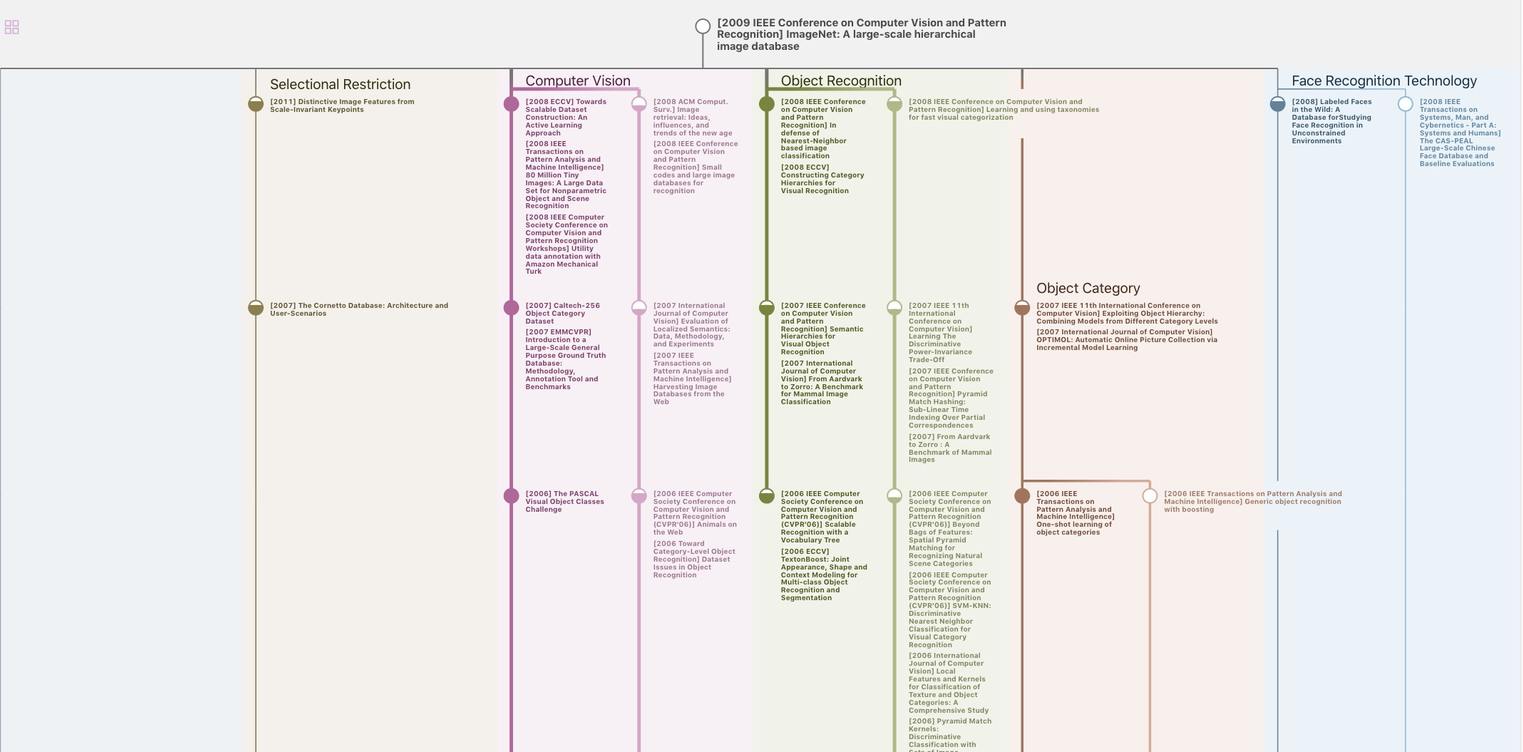
生成溯源树,研究论文发展脉络
Chat Paper
正在生成论文摘要