Learning Dense Visual Object Descriptors to Fold Two-Dimensional Deformable Fabrics
2023 IEEE 13th International Conference on CYBER Technology in Automation, Control, and Intelligent Systems (CYBER)(2023)
摘要
Manipulating two-dimensional fabrics is a significant research field in recent years. Fabric manipulation presents a formidable challenge due to the intricate dynamics and high-dimensional state space inherent in the process, prior research has predominantly relied on robot learning of task-specific strategies as a means to effectively accomplish the corresponding fabric manipulation tasks. In this work, we utilize dense visual object descriptors trained on synthetic RGB images to learn visual representations for two-dimensional fabrics. Based on the learned descriptors, the robot can learn the correspondences of similar fabrics in different configurations. We apply a novel Siamese network architecture to improve the quality of learned descriptors for three types of fabrics, including square fabrics, T-shirts and shorts. By utilizing the learned descriptors, the equivalent actions in an unknown configuration can be computed based on a fabric folding demonstration in an initial configuration. We perform a series of fabric folding tasks in different colors, sizes and shapes. The policy can achieve 87.7% average task success rate across 7 different folding tasks.
更多查看译文
关键词
7 different folding tasks,corresponding fabric manipulation tasks,dense visual object descriptors,fabric folding demonstration,fabric folding tasks,high-dimensional state space,learned descriptors,robot learning,significant research field,similar fabrics,square fabrics,task-specific strategies,two-dimensional deformable fabrics,two-dimensional fabrics,visual representations
AI 理解论文
溯源树
样例
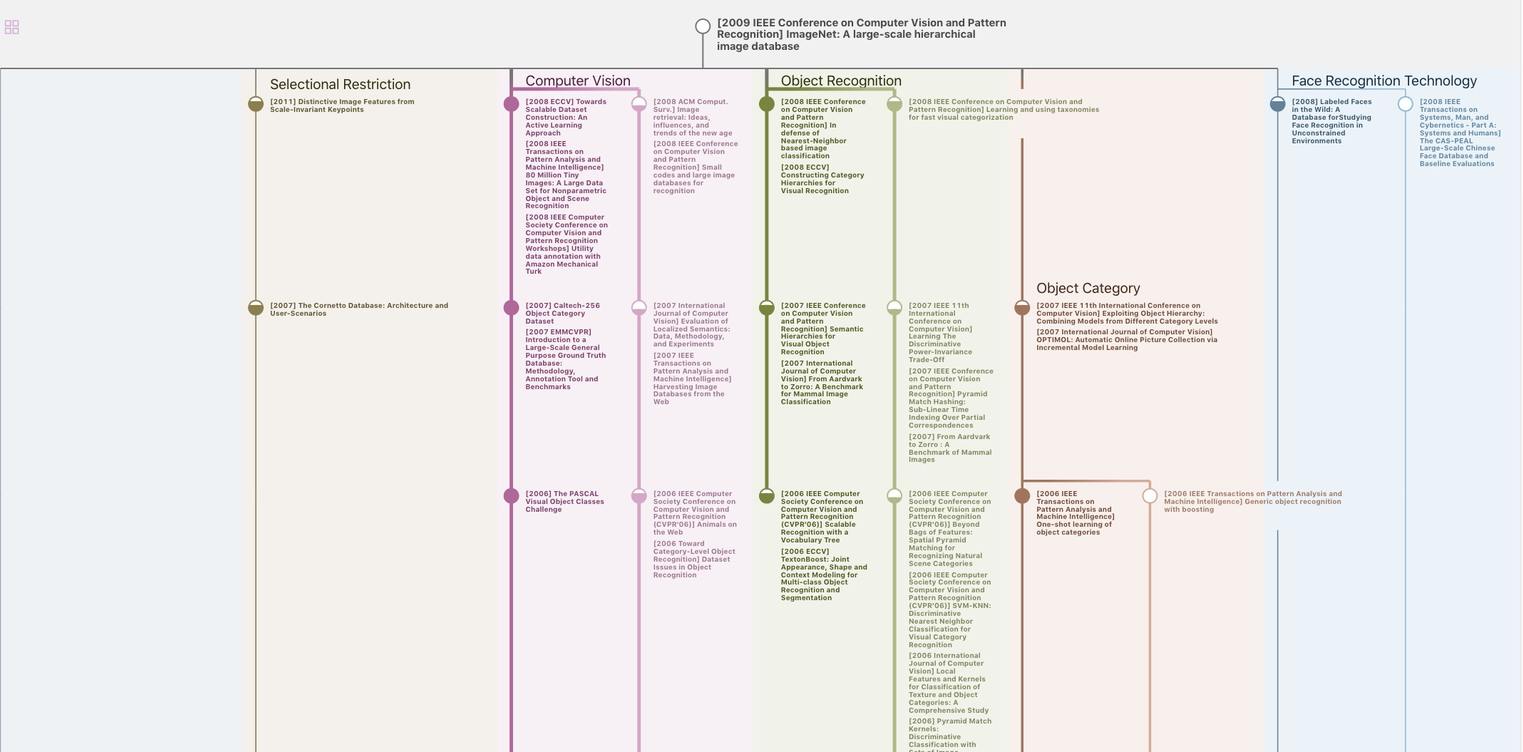
生成溯源树,研究论文发展脉络
Chat Paper
正在生成论文摘要