Molecule Attention Transformer
arxiv(2020)
摘要
Designing a single neural network architecture that performs competitively across a range of molecule property prediction tasks remains largely an open challenge, and its solution may unlock a widespread use of deep learning in the drug discovery industry. To move towards this goal, we propose Molecule Attention Transformer (MAT). Our key innovation is to augment the attention mechanism in Transformer using inter-atomic distances and the molecular graph structure. Experiments show that MAT performs competitively on a diverse set of molecular prediction tasks. Most importantly, with a simple self-supervised pretraining, MAT requires tuning of only a few hyperparameter values to achieve state-of-the-art performance on downstream tasks. Finally, we show that attention weights learned by MAT are interpretable from the chemical point of view.
更多查看译文
关键词
molecule attention transformer
AI 理解论文
溯源树
样例
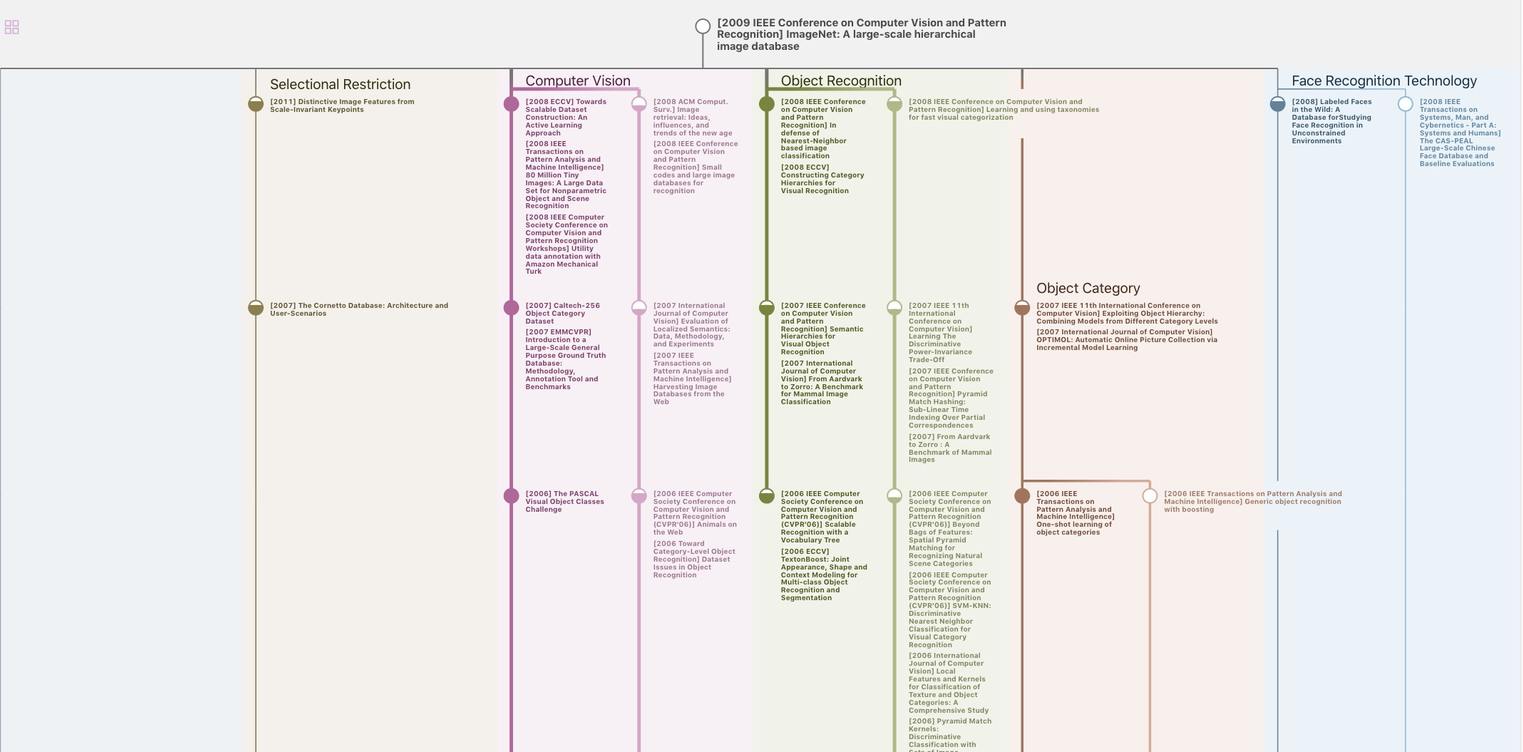
生成溯源树,研究论文发展脉络
Chat Paper
正在生成论文摘要