Integrated organic inference (IOI): A reconciliation of statistical paradigms
arXiv (Cornell University)(2020)
摘要
It is recognised that the Bayesian approach to inference can not adequately cope with all the types of pre-data beliefs about population quantities of interest that are commonly held in practice. In particular, it generally encounters difficulty when there is a lack of such beliefs over some or all the parameters of a model, or within certain partitions of the parameter space concerned. To address this issue, a fairly comprehensive theory of inference is put forward called integrated organic inference that is based on a fusion of Fisherian and Bayesian reasoning. Depending on the pre-data knowledge that is held about any given model parameter, inferences are made about the parameter conditional on all other parameters using one of three methods of inference, namely organic fiducial inference, bispatial inference and Bayesian inference. The full conditional post-data densities that result from doing this are then combined using a framework that allows a joint post-data density for all the parameters to be sensibly formed without requiring these full conditional densities to be compatible. Various examples of the application of this theory are presented. Finally, the theory is defended against possible criticisms partially in terms of what was previously defined as generalised subjective probability.
更多查看译文
关键词
integrated organic inference,ioi,statistical paradigms
AI 理解论文
溯源树
样例
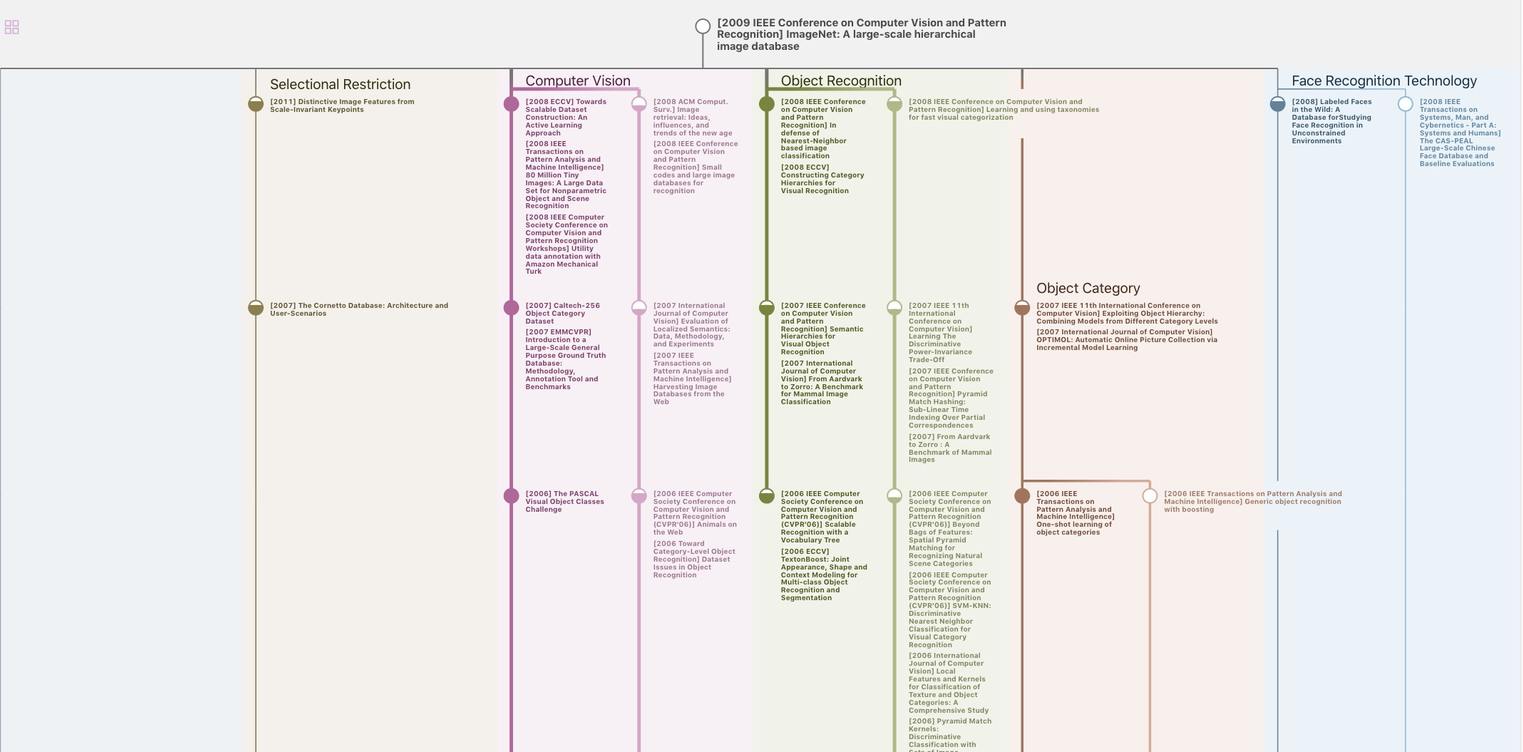
生成溯源树,研究论文发展脉络
Chat Paper
正在生成论文摘要