Multitask Learning with Capsule Networks for Speech-to-Intent Applications
2020 IEEE INTERNATIONAL CONFERENCE ON ACOUSTICS, SPEECH, AND SIGNAL PROCESSING(2020)
摘要
Voice controlled applications can be a great aid to society, especially for physically challenged people. However this requires robustness to all kinds of variations in speech. A spoken language understanding system that learns from interaction with and demonstrations from the user, allows the use of such a system in different settings and for different types of speech, even for deviant or impaired speech, while also allowing the user to choose a phrasing. The user gives a command and enters its intent through an interface, after which the model learns to map the speech directly to the right action. Since the effort of the user should be as low as possible, capsule networks have drawn interest due to potentially needing little training data compared to deeper neural networks. In this paper, we show how capsules can incorporate multitask learning, which often can improve the performance of a model when the task is difficult. The basic capsule network will be expanded with a regularisation to create more structure in its output: it learns to identify the speaker of the utterance by forcing the required information into the capsule vectors. To this end we move from a speaker dependent to a speaker independent setting.
更多查看译文
关键词
Spoken Language Understanding, Capsule Networks, Multitask Learning, End-to-end, Speaker Identification
AI 理解论文
溯源树
样例
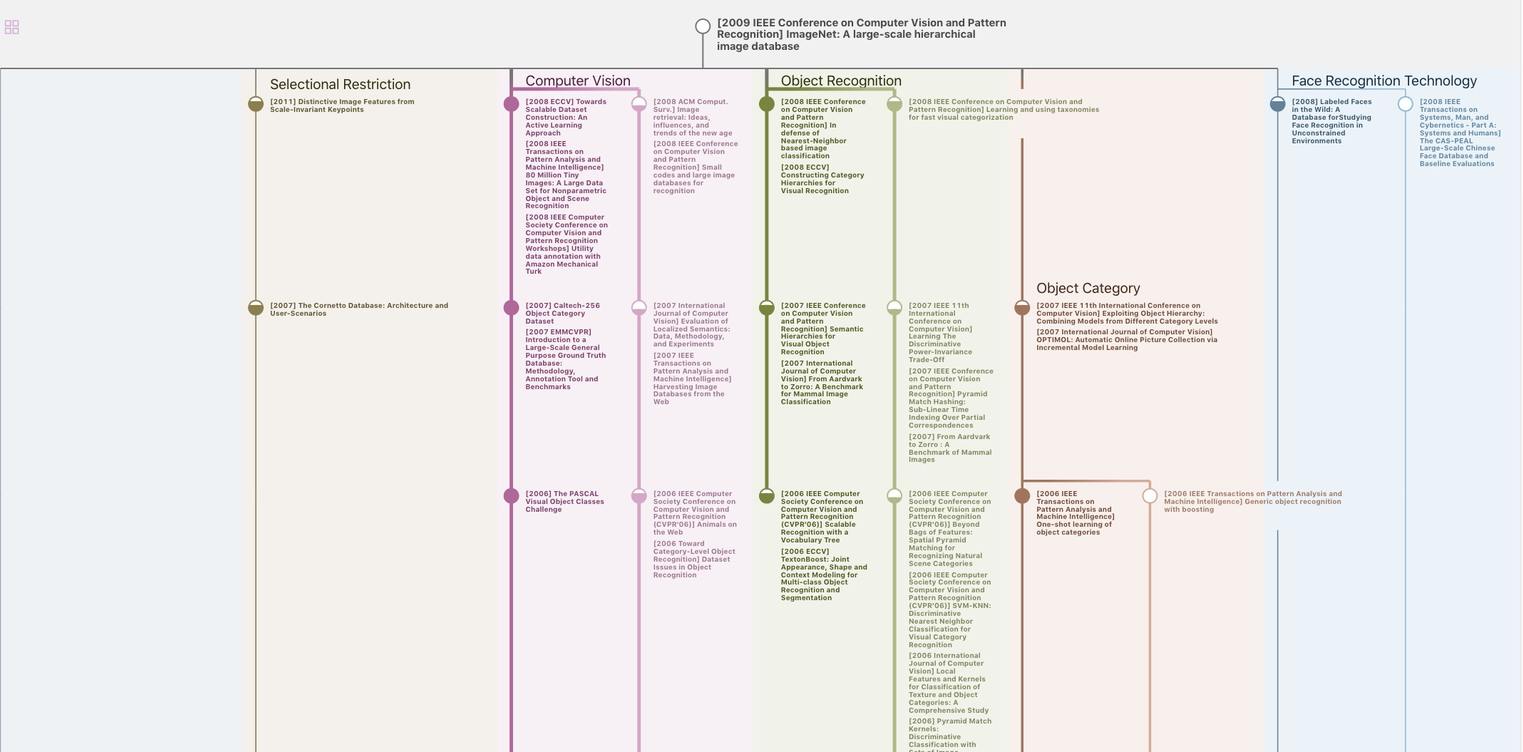
生成溯源树,研究论文发展脉络
Chat Paper
正在生成论文摘要