Restricted Structural Random Matrix For Compressive Sensing
SIGNAL PROCESSING-IMAGE COMMUNICATION(2021)
摘要
Compressive sensing (CS) is well-known for its unique functionalities of sensing, compressing, and security (i.e. equal importance of CS measurements). However, there is a tradeoff. Improving sensing and compressing efficiency with prior signal information tends to favour particular measurements, thus decreasing security. This work aimed to improve the sensing and compressing efficiency without compromising security with a novel sampling matrix, named Restricted Structural Random Matrix (RSRM). RSRM unified the advantages of frame-based and block-based sensing together with the global smoothness prior (i.e. low-resolution signals are highly correlated). RSRM acquired compressive measurements with random projection of multiple randomly sub-sampled signals, which was restricted to low-resolution signals (equal in energy), thereby its observations are equally important. RSRM was proven to satisfy the Restricted Isometry Property and showed comparable reconstruction performance with recent state-of-the-art compressive sensing and deep learning-based methods.
更多查看译文
关键词
Compressive sensing, Structural sparse matrix, Restricted isometry property, Security, Kronecker compressive sensing
AI 理解论文
溯源树
样例
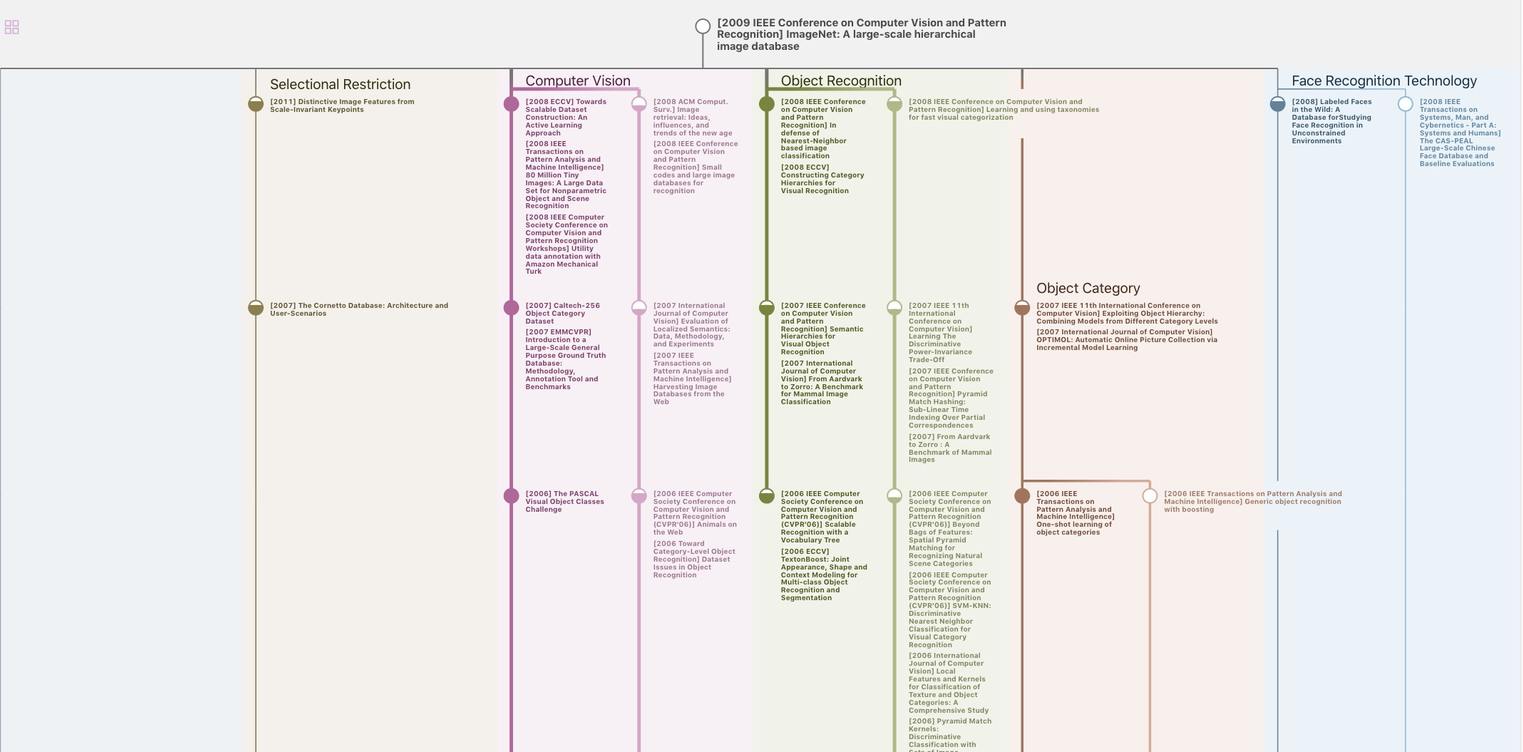
生成溯源树,研究论文发展脉络
Chat Paper
正在生成论文摘要