Small energy masking for improved neural network training for end-to-end speech recognition
2020 IEEE INTERNATIONAL CONFERENCE ON ACOUSTICS, SPEECH, AND SIGNAL PROCESSING(2020)
Abstract
In this paper, we present a Small Energy Masking (SEM) algorithm, which masks inputs having values below a certain threshold. More specifically, a time-frequency bin is masked if the filterbank energy in this bin is less than a certain energy threshold. A uniform distribution is employed to randomly generate the ratio of this energy threshold to the peak filterbank energy of each utterance in decibels. The unmasked feature elements are scaled so that the total sum of the feature values remain the same through this masking procedure. This very simple algorithm shows relatively 11.2 % and 13.5 % Word Error Rate (WER) improvements on the standard LibriSpeech test-clean and test-other sets over the baseline end-to-end speech recognition system. Additionally, compared to the input dropout algorithm, SEM algorithm shows relatively 7.7 % and 11.6 % improvements on the same LibriSpeech test-clean and test-other sets. With a modified shallow-fusion technique with a Transformer LM, we obtained a 2.62 % WER on the LibriSpeech test-clean set and a 7.87 % WER on the LibriSpeech test-other set.
MoreTranslated text
Key words
neural network, speech recognition, regularization, masking, dropout
AI Read Science
Must-Reading Tree
Example
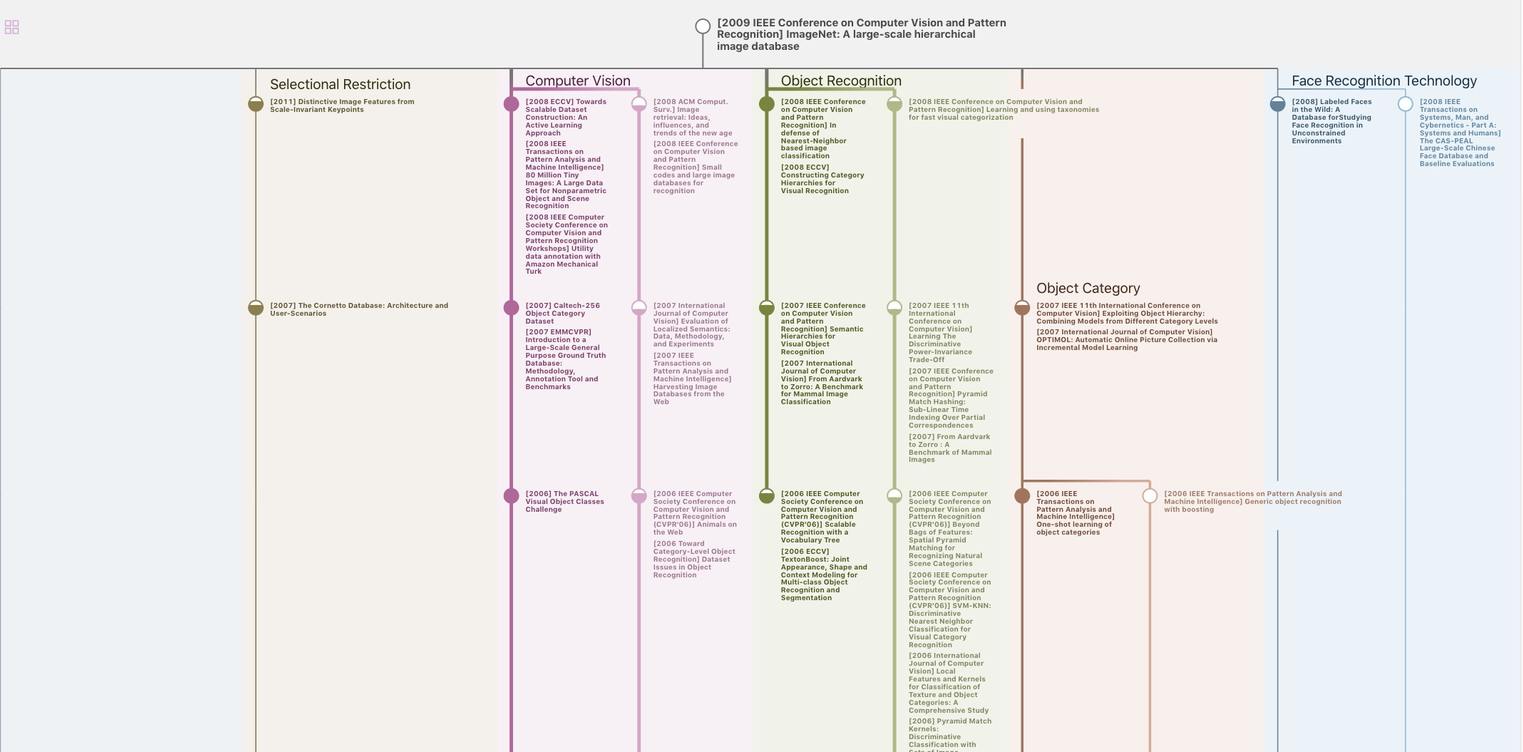
Generate MRT to find the research sequence of this paper
Chat Paper
Summary is being generated by the instructions you defined