Variational Autoencoder Based Latent Factor Decoding Of Multichannel Eeg For Emotion Recognition
2019 IEEE INTERNATIONAL CONFERENCE ON BIOINFORMATICS AND BIOMEDICINE (BIBM)(2019)
摘要
Robust cross-subject emotion recognition based on multichannel EEG has always been a hard work. In this work, we hypothesize there exists default brain variables across subjects in emotional processes. Hence, the states of the latent variables that related to emotional processing must contribute to building robust recognition models. We propose to utilize variational autoencoder (VAE) to determine the latent factors from the multi-channel EEG. Through sequence modeling method, we examine the emotion recognition performance based on the learnt latent factors. The performance of the proposed methodology is verified on two public datasets (DEAP and SEED), and compared with traditional matrix factorization based (ICA) and autoencoder based (AE) approaches. Experimental results demonstrate that neural network is suitable for unsupervised EEG modeling and our proposed emotion recognition framework achieves the state-of-the-art performance. As far as we know, it is the first work that introduces VAE into multichannel EEG decoding for emotion recognition.
更多查看译文
关键词
Affective Computing, Latent Factor Decoding, Emotion Recognition, EEG, Variational Autoencoder
AI 理解论文
溯源树
样例
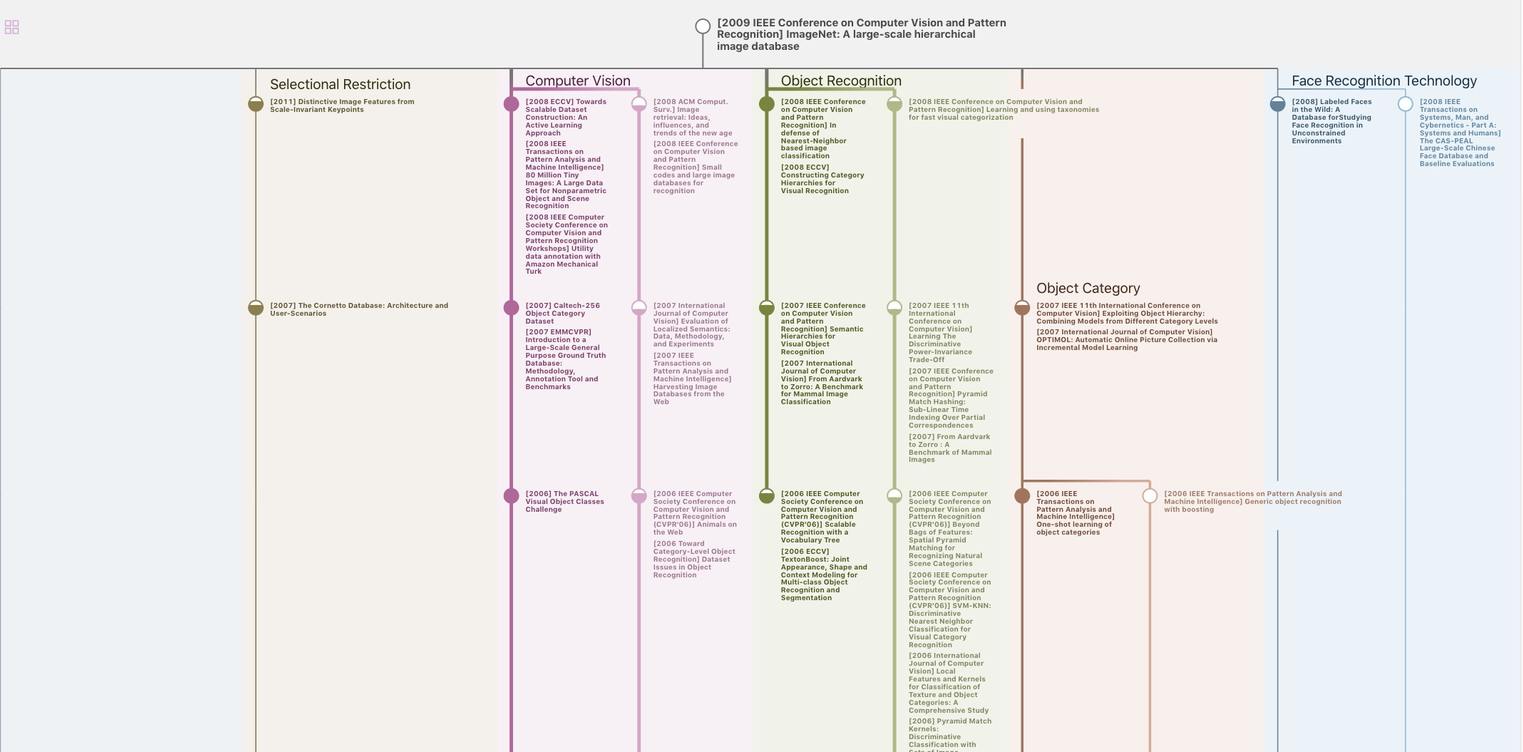
生成溯源树,研究论文发展脉络
Chat Paper
正在生成论文摘要