Understanding The Effects Of Dichotomization Of Continuous Outcomes On Geostatistical Inference
SPATIAL STATISTICS(2021)
摘要
Diagnosis is often based on the exceedance or not of continuous health indicators of a predefined cut-off value, so as to classify patients into positives and negatives for the disease under investigation. In this paper, we investigate the effects of dichotomization of spatially-referenced continuous outcome variables on geostatistical inference. Although this issue has been extensively studied in other fields, dichotomization is still a common practice in epidemiological studies. Furthermore, the effects of this practice in the context of prevalence mapping have not been fully understood. Here, we demonstrate how spatial correlation affects the loss of information due to dichotomization, how linear geostatistical models can be used to map disease prevalence and thus avoid dichotomization, and finally, how dichotomization affects our predictive inference on prevalence. To pursue these objectives, we develop a metric, based on the composite likelihood, which can be used to quantify the potential loss of information after dichotomization without requiring the fitting of Binomial geostatistical models. Through a simulation study and two applications on disease mapping in Africa, we show that, as thresholds used for dichotomization move further away from the mean of the underlying process, the performance of binomial geostatistical models deteriorates substantially. We also find that dichotomization can lead to the loss of fine scale features of disease prevalence and increased uncertainty in the parameter estimates, especially in the presence of a large noise to signal ratio. These findings strongly support the conclusions from previous studies that dichotomization should be always avoided whenever feasible. (C) 2020 Elsevier B.V. All rights reserved.
更多查看译文
关键词
Binary data, Dichotomization, Disease mapping, Linear geostatistical model, Model-based geostatistics, Prevalence
AI 理解论文
溯源树
样例
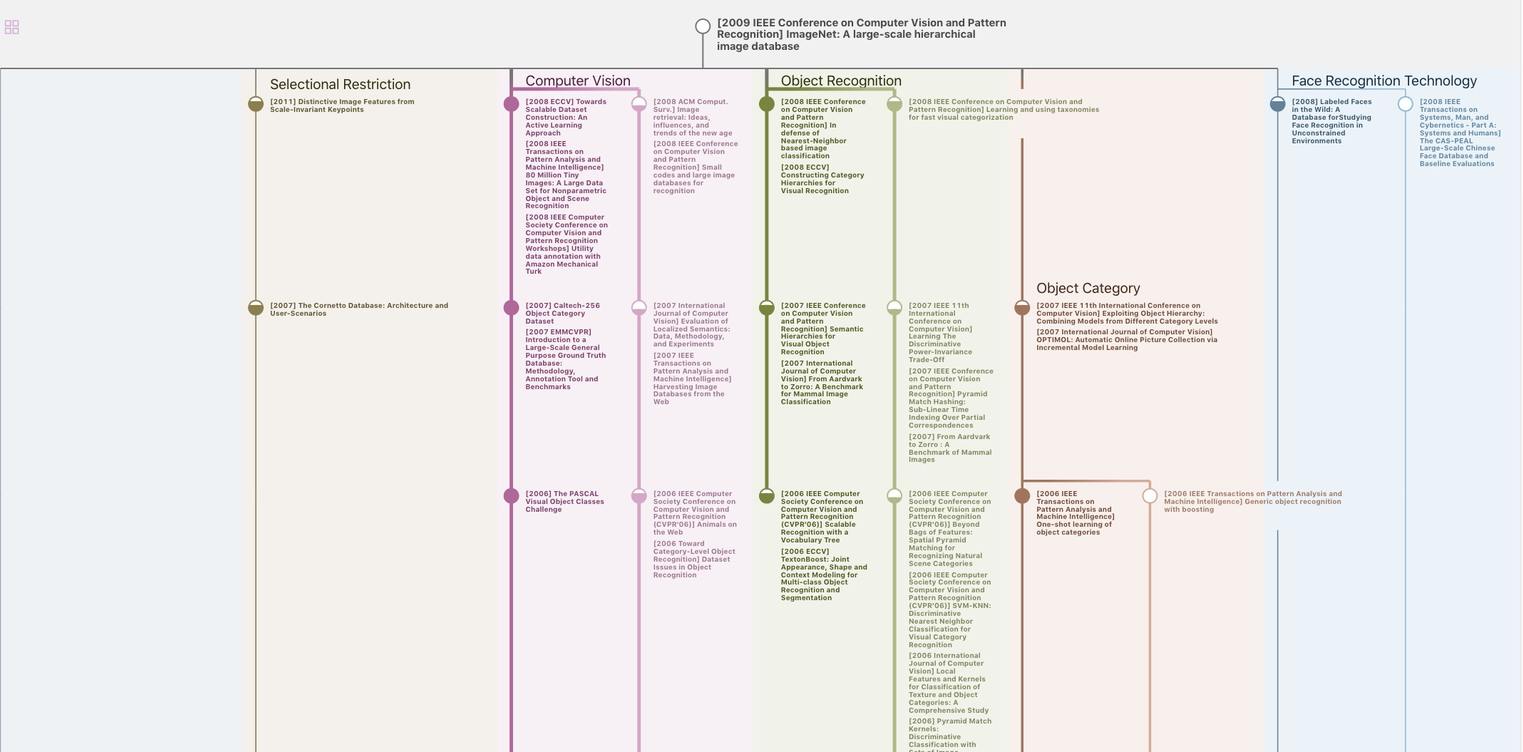
生成溯源树,研究论文发展脉络
Chat Paper
正在生成论文摘要