Machine-learning-assisted insight into spin ice Dy 2 Ti 2 O 7
NATURE COMMUNICATIONS(2020)
摘要
Complex behavior poses challenges in extracting models from experiment. An example is spin liquid formation in frustrated magnets like Dy 2 Ti 2 O 7 . Understanding has been hindered by issues including disorder, glass formation, and interpretation of scattering data. Here, we use an automated capability to extract model Hamiltonians from data, and to identify different magnetic regimes. This involves training an autoencoder to learn a compressed representation of three-dimensional diffuse scattering, over a wide range of spin Hamiltonians. The autoencoder finds optimal matches according to scattering and heat capacity data and provides confidence intervals. Validation tests indicate that our optimal Hamiltonian accurately predicts temperature and field dependence of both magnetic structure and magnetization, as well as glass formation and irreversibility in Dy 2 Ti 2 O 7 . The autoencoder can also categorize different magnetic behaviors and eliminate background noise and artifacts in raw data. Our methodology is readily applicable to other materials and types of scattering problems.
更多查看译文
关键词
Computational methods,Magnetic properties and materials,Science,Humanities and Social Sciences,multidisciplinary
AI 理解论文
溯源树
样例
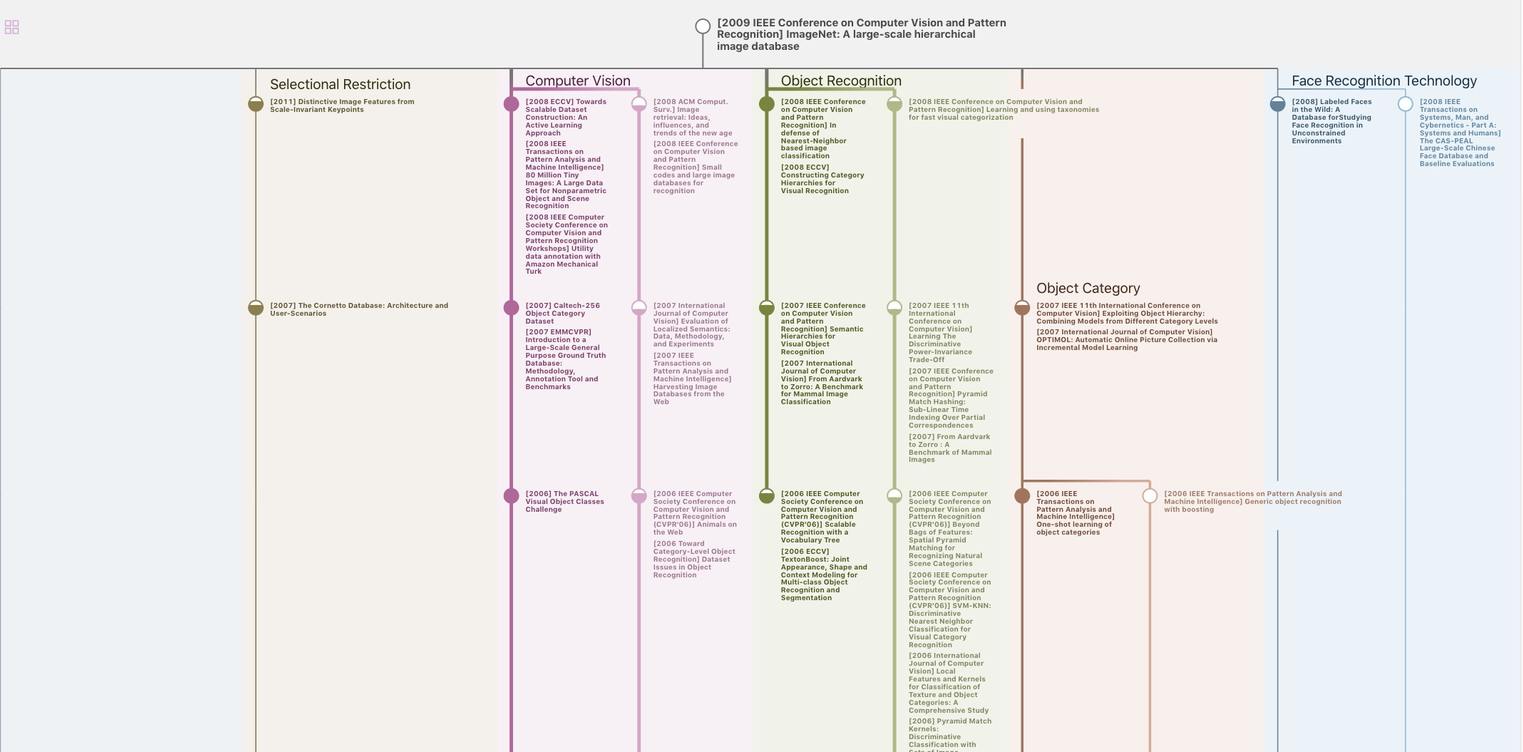
生成溯源树,研究论文发展脉络
Chat Paper
正在生成论文摘要